AI model uses physician notes to predict survival after cancer diagnosis
Key takeaways:
- The best AI models had an accuracy of greater than 80%.
- “Breast” and “prostate” positively predicted 6-month survival; “liver,” “glioblastoma” and “lung” negatively predicted 60-month survival.
Researchers from University of British Columbia have developed an artificial intelligence model that can accurately predict survival after a cancer diagnosis using physician notes from an initial oncology consult.
The artificial intelligence (AI) model employs natural language processing to predict short- and long-term survival with greater than 80% accuracy, according to study results published in JAMA Network Open.
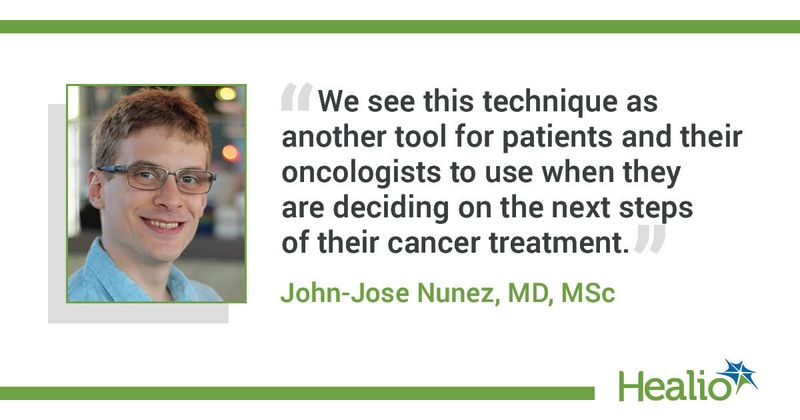
“Having a better understanding of treatment outcomes is an important component of personalized cancer care,” John-Jose Nunez, MD, MSc, psychiatrist and clinical research fellow with University of British Columbia Mood Disorders Centre and BC Cancer, told Healio. “We see this technique as another tool for patients and their oncologists to use when they are deciding on the next steps of their cancer treatment.”
Background
Despite the recent spike in publicity related to AI, Nunez said he has always had an interest in using computational techniques to help patients in medicine.
One of his group’s goals is to harness the ability of AI to go beyond its current commercial exploits and achieve more lofty objectives to help patients with cancer.
“Oncologists know all the stats inside and out when it comes to population-level survival rates, but some evidence shows that when it comes to the patient in front of them, it becomes harder to predict survival,” Nunez said.
The researchers sought to develop an AI model that would allow for a more personalized survival prediction using unstructured data that provides potential for more portability, Nunez said.
Methodology
Nunez and colleagues conducted a retrospective prognostic study of 47,625 patients (mean age, 64.9 ± 13.7 years; 53.4% women) who began care at a BC Cancer facility between April 1, 2011, and Dec. 31, 2016.
Researchers obtained patient data and initial oncologist consultation documents from BC Cancer and analyzed them using four previously established language models, including one traditional nonneural method language model and three models using neural networks.
Investigators obtained administrative mortality data from BC Vital Statistics. The analysis excluded patients with multiple cancer diagnoses.
The AI model’s performance when predicting patients’ survival at 6, 36 or 60 months served as the study’s primary outcome. Investigators assessed model performance using common metrics, including accuracy, specificity, sensitivity, balanced accuracy and receiver operating characteristics area under the curve (AUC). The secondary outcome comprised the words used in the model and their impact on predictive values.
April 6, 2022, served as the data cutoff date for updated mortality data.
Key findings
Among patients with a minimum of 5 years of follow-up observation, investigators observed mean survival time of 61.7 ± 40.3 months after diagnosis and 59.9 ± 39.9 months after initial oncology consultation for the entire study cohort.
Starting from their initial oncologist consultation, researchers reported that 41,447 patients (87%) survived 6 months, 31,143 (65.4%) survived 36 months and 27,880 (58.5%) survived 60 months.
On a holdout test set, the best AI models achieved a balanced accuracy of 0.856 (AUC = 0.928) for 6-month survival prediction, 0.842 (AUC = 0.918) for 36-month survival prediction and 0.837 (AUC = 0.918) for 60-month survival prediction.
A root word analysis of the dialogue in records and physicians’ notes showed the words “breast” and “prostate” to be positive predictors for 6-month survival, whereas “liver,” “glioblastoma” and “lung” served as negative predictors of 60-month survival.
Clinical implications
The key takeaway from a research perspective is that survival predictions can be made accurately without an overwhelming amount of structured data, according to Nunez. Accurate predictions can be derived from the unstructured data that is readily available in electronic health records at an oncologist’s office.
From a clinical perspective, Nunez said this tool is still a few years away from widespread deployment and will require further validation using documentation from other regions.
“AI will have many interesting tools come down the pipeline,” he told Healio. “I would encourage clinicians to keep an open mind to using them as they become available.”
For more information:
John-Jose Nunez, MD, MSc, can be reached at johnjose.nunez@bccancer.bc.ca.