AI technology predicts benefit of neoadjuvant chemotherapy for breast cancer
Click Here to Manage Email Alerts
Researchers at University of Waterloo have developed an artificial intelligence system, designed in combination with new MRI technology, to identify patients who might benefit from neoadjuvant chemotherapy for breast cancer.
“It’s actually very hard to predict whether or not a patient would benefit from [neoadjuvant chemotherapy], and that’s been a key challenge,” Alexander Wong, PhD, professor and Canada research chair in AI and medical imaging at University of Waterloo, told Healio. “Currently, one can assess the condition and size of tumors based on clinical experience to make predictions, but there are also some subtle signs and patterns that a clinician might not be able to see clearly.”
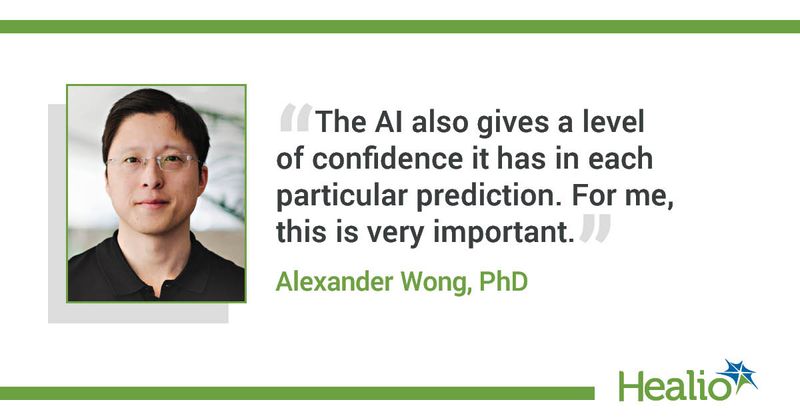
The AI algorithm, presented at the NeurIPS 2022 conference in New Orleans, is part of the open-source Cancer-Net initiative.
The researchers trained the AI software — called synthetic correlated diffusion imaging (CDI) — using images of breast cancer obtained through the new MRI modality that Wong and his team invented. Using images of previous breast cancer cases and information about the outcomes of those cases, the AI software determines whether neoadjuvant chemotherapy would likely be beneficial in a particular case.
Wong spoke with Healio about the combination of the new MRI technology and AI software, and the concept of “explainable AI” to guide clinical decision-making.
Healio: How does your AI software work?
Wong: This is a combination of a new form of MRI that can capture nuances and special tumor characteristics that current MRI systems cannot. We then combine that more detailed imaging with AI, which can meticulously look at these different cases and understand these subtle patterns. The AI is able to look through these MRI images and identify different patterns that are indicative of whether neoadjuvant chemotherapy would be effective. Then it will give a prediction on whether that particular chemotherapy treatment would be effective for that specific patient.
The AI also gives a level of confidence it has in each particular prediction. For me, this is very important. Instead of just a simple “yes” or “no,” we want to be able to have further insights and clarity. This AI looks at an image and it won’t just say, “I think this patient will benefit from this form of chemotherapy.” It will say, “I think this patient will benefit from this form of chemotherapy, but I’m only 51% sure that this is the case.” Physicians can use this information to make their best judgment. They can factor in the AI’s level of certainty when making their decisions.
Healio: How have the AI system and your new MRI technology performed so far?
Wong: In a retrospective study on a patient cohort of about 254 patients with breast cancer across 10 different institutions, we found that it was providing higher predictive accuracy than is achieved with other MRI modalities. We had around 87% accuracy. It’s not perfect, but it is doing a good job so far, especially compared with existing approaches.
Healio: What is next in your work on this?
Wong: We have a few goals. One is to apply this to larger cohorts so we can have a better understanding of how the AI system performs on more patients. Even if we find that the current AI system doesn’t perform as well on a larger cohort, we will then have more information to teach the AI to do better.
Another area we are working on in the lab is “explainable AI.” We would like to probe or query our AI to have a better understanding of what is driving its decisions. If it says a certain case might benefit from neoadjuvant chemotherapy, what is that determination based on? Is it looking at where the tumor lies, or at certain parts of the tumor? We want to try to understand these additional biomarkers and indicators, just to ensure the AI is making the right decisions for the right reasons.
This will also provide insights into what might be good biomarkers and patterns that clinicians might not yet know about or might not take into consideration. This is especially valuable as far as building trust in AI for clinicians. It’s one thing to provide a simple “yes” or “no,” but more meaningful for the AI to tell us how confident the system is in a prediction and now, at this next level, to explain its rationale for that prediction. That just makes it more powerful.
For more information:
Alexander Wong, PhD, can be reached at University of Waterloo, 200 University Ave. West, Waterloo, ON, Canada N2L 3G1.