AI algorithm identifies potential therapeutic targets for glioblastoma
An artificial intelligence algorithm that uses computational analysis to identify possible therapeutic targets for glioblastoma multiforme and other types of cancer could have broad treatment implications, according to its developers.
Researchers from Sylvester Comprehensive Cancer Center at University of Miami Miller School of Medicine, in cooperation with international investigators, developed the SPHINKS algorithm described in a study published in Nature Cancer.
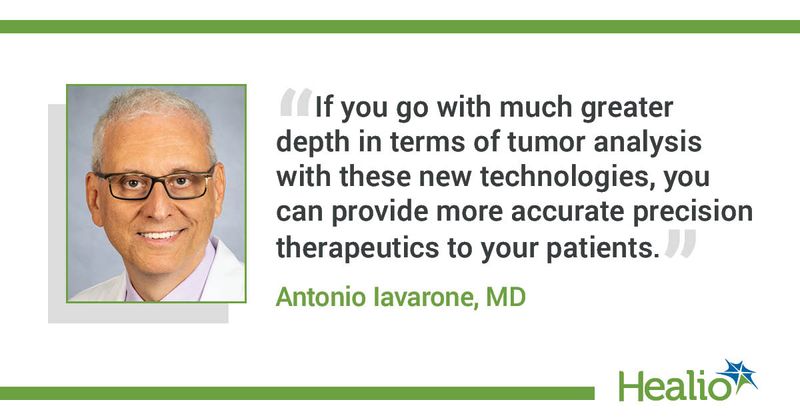
“As of today, every single patient with glioblastoma receives the same exact therapy, which is a standard of care composed of radiotherapy plus temozolomide,” study lead author Antonio Iavarone, MD, deputy director of Sylvester Comprehensive Cancer Center, told Healio. “This therapy has very little efficacy, if any, in the sense that it improves the median survival of patients with glioblastoma by only 3 months. Most importantly, it doesn’t seem to account for the exceptional degree of heterogeneity of these tumors.”
Iavarone spoke with Healio about the information the SPHINKS algorithm has yielded so far, its potential in targeting glioblastoma multiforme and how it may be applied to other types of cancer.
Healio: What inspired you to develop this algorithm?
Iavarone: Our laboratory studies glioblastoma using all the new technologies available, including those that analyze tumors with single-cell resolution. Each tumor is different between patients. Even within a patient, you have many different types of tumor cells.
This is where we got the idea of segregating and stratifying cases of glioblastoma based on the most important biologic features associated with these tumors. We extract these features from multiomics big-data analyses that go beyond genomics. Genomics is what has been used so far to drive the concept of precision therapy. However, in the case of glioblastoma and other tumor types, simply knowing there is a mutation is not sufficient to successfully target the tumor, because you don’t know what the mutation is doing. You lack a lot of crucial information, and that is essentially the reason these targeted therapies based on gene panels have not resulted in significantly improved results.
In this manuscript, we used a large array of multiomics data to reinforce a new classification of glioblastoma that we had recently reported. Most importantly, we have created a new artificial intelligence- and machine-learning-based algorithm to identify the master kinases, the protein kinases that are driving each specific tumor. We focused on these particular targets because there are many different drugs that can interfere with the function of these proteins.
Healio: What does the SPHINKS algorithm do?
Iavarone: Our algorithm uses these new proteogenomics and multiomics data to extract the master kinases. Another important translational concept we introduced is the experimental component that is essential to complement the computational part of the work. We used “tumor avatars,” which are three-dimensional cultures of tumor cells directly generated from each patient’s tumor, to validate the prediction from the algorithm. So, when we predict there is a very important master kinase in a particular tumor type, we go to the tumor cells that are collected in the operating room for a particular patient. Using these tumor avatars, we can ask whether drugs against those kinases successfully target the ability of these tumors to expand and grow. In particular, we found that drugs against two distinct glioblastoma subtype-specific master kinases, protein kinase C delta and DNA-independent protein kinase, show efficacy against the avatars of the respective subtype.
This work is ready to be implemented in the clinical setting, and toward that goal, our recently published paper includes information about a classifier that is freely available for any clinical diagnostic center, any molecular pathology lab. The sequencing data from any brain tumor can be inserted into the classifier, which outputs information about the subtype to which the tumor belongs. Obviously, together with that, there are now targeted therapeutic opportunities based on these master kinases.
Healio: How might this algorithm be applicable to other tumor types?
Iavarone: In this study, we also showed the application to data sets of breast cancer and lung cancer — particularly squamous cell lung cancer. In those contexts, we also identify the same four subtypes. We identify the same master kinases, the same therapeutic targets that are used in the case of glioblastoma multiforme. Of course, we have not done extensive experimental validation for these other tumor types, but in principle, I would say this is applicable to most solid tumor types.
The final mission that comes from our work is that the more multiomics data you generate for each patient with a solid tumor, the more accurate your therapeutic prediction can be. This is a very important new concept that I hope to have the opportunity to deliver to the clinical community at the next ASCO meeting.
Currently, precision cancer medicine in general is associated with very superficial contexts. You analyze a small panel of genes for the presence of mutations, and based on that, you get the information and now you use a drug on it. Unfortunately, when we go with such a level of simplification, we have very negative results, in the sense that these therapies don’t work.
What we show in this work is that if we have the possibility to analyze this large amount of multiomics data — and especially proteomic and phospho-proteomic data — we can identify the most active kinases or the most active targets that can be therapeutically attacked with individual drugs for each patient.
Healio: Is there anything else you’d like to say about this research?
Iavarone: What I’m trying to convey to the medical field is that if you go with much greater depth in terms of tumor analysis with these new technologies, you can provide more accurate precision therapeutics to your patients. So, we should not stop with the simple analysis of the few genes that come from these panels, but we should go with things that are more sophisticated. Our contribution is an important step in this direction.
For more information:
Antonio Iavarone, MD, can be reached at Sylvester Comprehensive Cancer Center, University of Miami, 1475 NW 12th Ave., Miami, FL 33136; email: axi435@med.miami.edu.