Machine learning-based methods predict early-stage melanoma recurrence
Researchers at Massachusetts General Hospital and Harvard Medical School have developed machine learning-based methods to predict which patients with early-stage melanoma have the highest risk for disease recurrence.
The methods could help reveal those who may benefit from greater surveillance or adjuvant immunotherapy, the investigators wrote in a study published in npj Precision Oncology.

“In 2021, immune checkpoint inhibitors that historically have been used to treat more advanced melanomas received approval for the management of stage IIB and IIC disease,” Yevgeniy R. Semenov, MD, MA, assistant professor and principal investigator of the Dermatology Clinical Informatics Laboratory at Massachusetts General Hospital, told Healio. “Clearly, not everyone who has early-stage disease will experience a recurrence. If we look at historical data, only about 20% to 30% of even the stage IIB and IIC groupings would experience recurrence, but that would mean giving these immunotherapies to 100% of patients in a situation where maybe only 20% to 30% would benefit.”
Semenov and colleagues amassed 1,720 early-stage melanomas and extracted 36 clinical and pathologic features of these cancers from electronic health records to predict patients’ recurrence risk with machine learning algorithms.
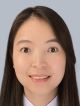
“Melanoma recurrence is a time-to-event outcome,” Guihong Wan, PhD, a postdoctoral research fellow at Massachusetts General Hospital and Harvard Medical School, told Healio. “We built machine learning models for both binary melanoma recurrence classification and time-to-event risk prediction.”
Semenov and Wan spoke with Healio about the need to accurately predict melanomas at high risk for recurrence and their research team’s approach.
Healio: What inspired you to devise a machine learning-based method of predicting melanoma recurrence?
Semenov: The main effort to prognosticate outcomes in cancer has historically come from our staging criteria. For melanoma, we currently use the American Joint Committee on Cancer, eighth edition (AJCC-8). These staging criteria are meant to look primarily at survival outcomes — recurrence is just a secondary component. Of course, if something recurs, it is more likely that the disease is aggressive and will lead to poorer survival outcomes, but that’s not always the case. Additionally, the AJCC criteria only include a small subset of available features to stage the tumor, leaving a lot of potentially useful information untapped.
Wan: Machine learning has been applied to the prediction of other cancer outcomes with EHR data. However, the cumulative predictive capacity of the included features using machine learning is underexplored.
Healio: How did you assess the efficacy of this prediction method?
Semenov: We followed very standard approaches. Whenever you develop these machine learning tools, you have to essentially come up with separate independent cohorts. You train and develop the tool on one cohort, and then another unrelated cohort is used to validate the tool. We looked at five or more different machine-learning algorithms, and we independently trained and validated each approach on distinct cohorts of patients. For example, here in Boston, we worked with the Mass General Brigham health care system, as well as Dana-Farber Cancer Institute. This particular study included over 1,700 patients, which is a very large retrospective cohort of patients with early-stage melanoma. We trained the models on a subset of the Mass General Brigham patients and tested them on subsets of the Dana-Farber population. We found that especially in our best-performing models, the area under the receiver operating characteristic curve (AUC) was closer to 0.85. So, if you were to take what has been historically possible with staging alone of 0.65, that is a significant improvement.
Wan: We used a comprehensive list of criteria to evaluate the model performance, including AUC, positive predictive value, sensitivity, specificity, and accuracy for binary classification and time-dependent AUC and concordance index.
Healio: Your study identified tumor thickness and the rate of cancer cell division as the most important predictive features. What other factors were highly predictive of recurrence?
Semenov: Although tumor thickness and mitotic rate were among the most important features contributing to our overall prediction, the benefit of these machine-learning approaches is comprehensively evaluating contributions from all included features. If you look at the features that our models selected as highly contributing, sometimes mitotic rate was number one, and sometimes tumor thickness was number one. However, there were other contributors, like age at the time of diagnosis and socioeconomic status, for example. It’s a very comprehensive array of clinical history variables, demographics and tumor synoptic features extracted from the pathology report. We don’t just look at a small set — it was 36 features altogether. In combination, we found that if you do this, you tend to get better performance than if you simply rely on what has been historically used for staging.
Healio: What is next in your work on this?
Semenov: This is the first step in a longer process. The reason we started with clinically derived variables extracted from the EHRs is that it is relatively easy for any clinician to implement at this point. We’re hoping to create an online tool to allow anyone, regardless of where they are in the country or the world, to take advantage of it. They can just plug in the patient characteristics and see that patient’s recurrence risk over the next 5 or 7 years.
Even though we’ve reached a fairly high classification performance of 0.85, we don’t intend to stop there. We would like to continue improving on it, and I believe that to do that, we need to incorporate more sophisticated data inputs. For example, we are working on a tool that incorporates digital histopathologic images from the primary tumor. These whole-slide images have been scanned using a digitizer to allow us to run computer vision models on these images and incorporate the information gleaned from that approach into the information we currently have. The step after that is to try to come up with more advanced molecular tests on top of our earlier approaches to further improve these techniques. Our goal is to get above 0.95 AUC, because with cancer, we want to be as precise as possible. However, even what we have now is an improvement over standard of care.
It also reopens the debate as to the role of mitotic rate in staging of melanoma, because it was actually dropped from the AJCC-8 criteria as part of staging. We’ve demonstrated that mitotic rate is important in predicting recurrence; in some cases, the most important feature we saw.
This tool is not meant to replace what exists today, it’s meant to augment our understanding of the disease trajectory and help clinicians make more informed decisions about how aggressively we should treat patients, especially those at risk for more aggressive disease.
For more information:
Yevgeniy R. Semenov, MD, MA, can be reached at Harvard Medical School, 40 Blossom St., Bartlett Hall 6R, Room 626, Boston, MA 02114; email: ysemenov@mgh,harvard,edu. Guihong Wan, PhD, can be reached at Harvard Medical School, 40 Blossom St., Bartlett Hall 6R, Room 626, Boston, MA 02214; email: gwan@mgh.harvard.edu.