Researchers use mathematics to help optimize immunotherapy for cancer treatment
The success of cancer immunotherapy comes with the risk for severe toxicity, and its efficacy in difficult-to-treat cancers has been accompanied by high rates of disease relapse.
One lab at Moffitt Cancer Center is using mathematics to help explain which patients will respond to immunotherapies and at which dose levels. The goal is to maximize therapeutic benefit while limiting exposure to unnecessary treatment, according to Heiko Enderling, PhD, associate professor of integrated mathematical oncology at Moffitt Cancer Center.
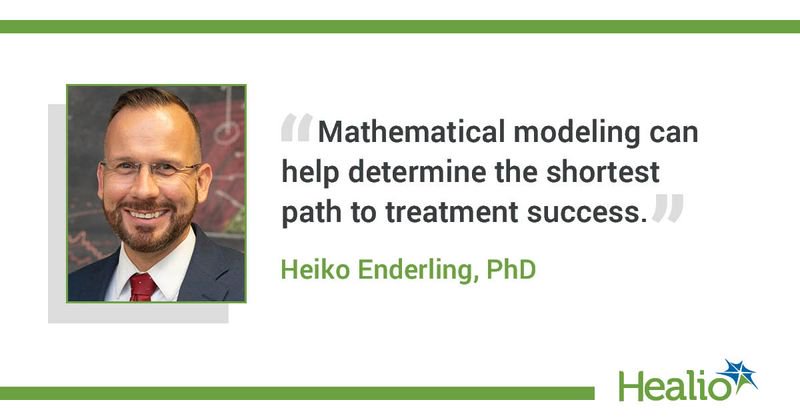
His lab is looking at ways to use mathematical modeling to optimize treatment of head and neck cancer and glioma. They are “looking for the right numbers” or, as he explained, a particular balance between cancer cells and immune cells that allows the immune system to engage effectively and win the longer-term battle.
“No immune biomarker exists to explain why two patients with similar clinical stage and molecular profiles would have different treatment outcomes,” Enderling told Healio. “It is critical to understand both the immune and tumor states to understand how the complex system will respond to treatment.”
Enderling spoke with Healio to discuss his group’s recent publication, in which they present an “integrated mathematical oncology approach” that can be used to improve responses to immunotherapy and combinational therapeutics while limiting toxicity on a personalized level.
Healio: What was the rationale for using mathematical modeling to analyze and predict treatment outcomes?
Enderling: We examined patient data — mostly biopsy data — and tried to correlate what we saw before treatment with responses and outcomes. When we spoke with biologists and clinicians about how tumor and immune cells interact with one another, it quickly became very clear that there is a nonlinear relationship. When written as a system of differential equations, you very quickly see the existence of a complex adaptive dynamic system. These systems are incredibly powerful in understanding nonlinear dynamics, and instead of looking how cell numbers change over time, we have developed a tool called phase plane analysis.
If one cancer cell is surrounded by a million immune cells that all can recognize that cancer cell, then there is a high likelihood that the immune system can kill that cancer cell. Conversely, if a million cancer cells are being targeted by one immune cell, it is almost certain that the cancer will outgrow that one immune cell. This example begs the question: What is the number of cells that would separate remission and recurrence?
Healio: How did you use mathematical modeling to determine this number?
Enderling: The key is understanding how these two different numbers interact. If you plot cancer cells on one axis and effector cells on the other axis, somewhere in between is the separation between a good outcome or a bad outcome. Providing cellular-based immunotherapy shifts one of these axes — that being, the number of effector cells — and the treatment will be successful if the curve shifts across this boundary.
Healio: How does knowing this number, or when this occurs, help inform clinical practice and guide treatment?
Enderling: It helps us to conceptualize ahead of time what is needed for each patient, and for some patients it indicates a type of immunotherapy that may not be viable. If our model is never able to get the number of cells needed such that the treatment may be successful, then it is an indicator that certain patients shouldn’t be candidates for adoptive cell transfer. Conversely, some of the new immunotherapies — such as immune checkpoint inhibitors — don’t really change the absolute number of cells at the time of therapy. Instead, these treatments change how cells interact with each other, which could increase the number of immune cell infiltrates over time. Immune checkpoint inhibition may require fewer immune cells to kill all the cancer cells than adoptive cell transfer because the immune cells being used are more potent.
Healio: So, your mathematical modeling can be useful for guiding dosage for both adoptive cell transfer and immune checkpoint inhibitors?
Enderling: Absolutely. And it could be applied to both immunotherapies in combination with chemotherapy or radiation therapy. If we understand what each treatment does to the balance in this complex system, then we can use optimal control to determine the best treatment for a patient. The optimization equation can also include the least amount of toxicity for the patient. There may be multiple ways to land on the favorable side of the effectiveness curve, including ones that are less toxic. Mathematical modeling can help determine the shortest path to treatment success given the underlying dynamics of how these cells interact.
Healio: How would your model work practically, and are you doing anything to translate this into a real-world setting?
Enderling: The goal of publishing this model was to explain the underlying dynamics and the effects of treatment on these dynamics. Our aim is to provide an educational tool or conceptual understanding about how immunotherapies impact a complex dynamic system.
A few things are required to make this model clinically translatable. First, we need to understand all the numbers and parameters of this dynamic system. For example:
How fast do cancer cells grow?
How quickly do immune cells proliferate when they are in contact with a tumor?
How often can immune cells kill cancer cells?
How quickly do the immune cells get exhausted?
It will be necessary to determine these numbers specifically for head and neck cancer, breast cancer, brain cancer, prostate cancer, melanoma and so on. Once the numbers behind the dynamic system have been determined, a predictive model can be used that produces patient-specific results. Coupling a knowledge of the underlying dynamics with patient-specific tumor biopsies will produce useful information that can help clinicians determine the complexity of a patient’s tumor microenvironment and guide treatment options. Nevertheless, this is all a long way off. If this process were a 100-step journey, then we might be on step five right now.
Healio: Is another goal of this project to prevent people from receiving unnecessary treatment?
Enderling: That is correct, and it may be the most important aspect of our endeavor. There is a lack of accurate biomarkers to determine why two patients have a different response to immunotherapy. We hope this model will help create a mathematical biomarker to determine which patients are likely to respond to immunotherapies.
Healio: Do you think that clinicians will be apprehensive to consult a mathematical model to guide treatment decisions?
Enderling: Close dialogue between different departments is necessary. At Moffitt Cancer Center, we have cultivated an environment in which this dialogue happens. We currently have a half dozen or so ongoing trials with clinical decisions being informed by mathematics. We are treating patients with calculus. We've found small teams of clinicians and mathematicians where this dialogue happens. We just completed a clinical trial where, based on mathematical modeling, intermittent hormone therapy was given for prostate cancer. The results were a doubling in the time until progression for a selected group of patients at less than half of the drug dose. Less treatment, but better outcomes by using the underlying disease dynamics.
Our latest publication is just a concept. We now need the concept translated into real-world data.
For more information:
Heiko Enderling, PhD, can be reached at heiko.enderling@moffitt.org.