New error grid better tailored to assess CGM accuracy
Key takeaways:
- The Diabetes Technology Society error grid allows researchers to determine how accurate a diabetes device is at measuring glucose concentrations.
- The new error grid includes a matrix for assessing CGM trends.
A new error grid to assess the accuracy of glucose measurements could give continuous glucose monitor manufacturers and regulatory agencies such as the FDA a better understanding of how accurate their devices are.
An international panel of 94 experts in glucose monitoring from all six continents unveiled the Diabetes Technology Society error grid in a paper published in the Journal of Diabetes Science and Technology. The new error grid is a successor to the society’s surveillance error grid that was published in 2014. The new grid was created to better define risk zones and make the grid more applicable to CGMs.
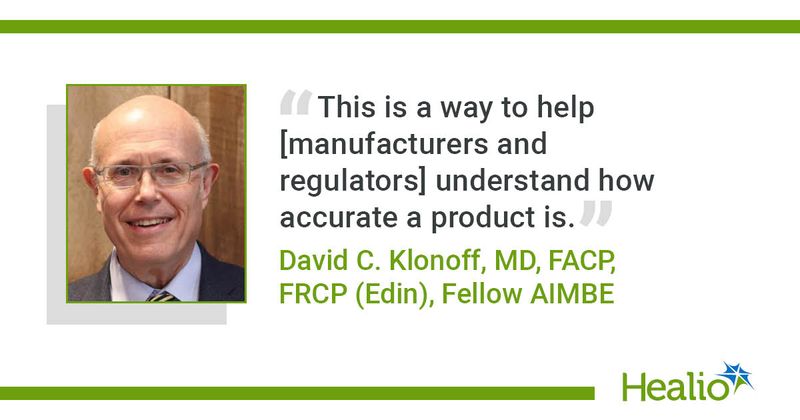
“This is a tool that will be used by manufacturers and by regulators so they can determine how accurate [devices] are,” David C. Klonoff, MD, FACP, FRCP (Edin), Fellow AIMBE, clinical professor of medicine at the University of California, San Francisco, and medical director of the Dorothy L. and James E. Frank Diabetes Research Institute of Mills-Peninsula Medical Center, told Healio. “The goal is that we want to have accurate products. This is a way to help both parties understand how accurate a product is.”
Building a better error grid
Glucose error grids are charts that allow researchers to compare glucose values measured by a diabetes device to a set of reference glucose concentrations and determine how close the measurements are to one another. Several error grids were created to evaluate to accuracy of blood glucose monitors (BGMs), according to Klonoff. The Clarke error grid was released in 1987 and the Parkes error grid was developed in 1994.
The most recent error grid was the surveillance error grid, which Klonoff and colleagues unveiled in a paper published in the Journal of Diabetes Science and Technology in 2014.
“The FDA asked us to [create the surveillance error grid] because they were processing recalls,” Klonoff said of the surveillance error grid. “When a product was recalled and it was inaccurate, the FDA wanted to get a sense of how dangerous it was or how much risk was associated with inaccurate readings.”
Klonoff said the surveillance error grid was a big step forward from the two previous error grids as it accounted for in new research in diabetes care, greater appreciate of the risk for hypoglycemia, and the introduction of newer insulins. However, Klonoff noted the grid had a few shortcomings, including questions regarding whether to use the same error grid for BGMs and CGM measurements, and the lack of an error grid for assessing a CGM’s ability to measure change in glucose.
“Until 2024, there was no error grid that was, by consensus, advisable for CGMs,” Klonoff said. “No one looked at whether you should use the same error grid for BGMs as CGMs.”
The Diabetes Technology Society error grid is similar to the surveillance error grid, Parkes error grid and Clarke error grid, in that it includes five risk categories: zone A (no risk), zone B (mild risk), zone C (moderate risk), zone D (high risk) and zone E (extreme risk).
For the Diabetes Technology Society error grid, researchers smoothed the risk zone borders of the old surveillance error grid using data from an accuracy study of 18 BGMs. Applying the smoothed error grid to data from a BGM accuracy study resulted in 2.6% of data pairs in zone A on the surveillance error grid moving to zone B and 2.6% of BGM pairs moving from zone B to zone A.
Researchers used the new error grid to evaluate point accuracy data from two sample BGMs and one combined CGM dataset. Researchers found one BGM had 89.7% of data pairs fall into zone A, the second BGM had 99.1% of measures in zone A, and the CGM dataset had 88.3% of data pairs in zone A.
Assessing CGM trends
The Diabetes Technology Society error grid includes a new component allowing researchers to assess the accuracy of CGM-reported trends compared with reference glucose trends. The Trend Accuracy Matrix uses a similar concept as the error grid, with five risk categories ranging from no risk to extreme risk. Mild risk was assigned when the CGM reported that the change in glucose was slightly less than the reference trend. Moderate risk was assigned when the CGM reported that the change in glucose was slightly more than the reference trend. High risk was assigned if the CGM substantially underestimates the rate of rise, and extreme risk was assigned when the CGM substantially overestimated the rate of rise. According to Klonoff, the FDA required that CGMs have a minimal number of trend data pairs in the high- and extreme-risk zones. Trends were categorized as change in glucose by milligram per deciliter per minute.
Researchers tested the Trend Accuracy Matrix using a combined CGM dataset. When all reference values of 600 mg/dL and under were included, 73% of CGM readings were the same as the reference measure. There were no CGM trend measures defined as extreme underestimate or extreme overestimate in the full analysis.
Researchers also investigated the relationship between the percentage of measures a diabetes device has in zone A of the Diabetes Technology Society error grid and mean absolute relative difference (MARD), which is an analytical accuracy measure used by many diabetes device companies, according to Klonoff. Researchers found 90% of measures in zone A on the Diabetes Technology Society error grid was approximately equal to a MARD of 10%. Every 1% increase in glucose measures placed in zone A is roughly equivalent to a 0.33% decrease in MARD, and every 1% decline in MARD is roughly equivalent to a 3% increase in measures found in zone A.
“Some companies will present to regulatory agencies either MARD or percent in zone A,” Klonoff said. “Using this conversion factor gives you an idea of what the other number is.”
Klonoff said he expects the Diabetes Technology Society error grid will become the definitive tool used by the FDA to assess the clinical accuracy of diabetes devices measuring glucose approval because it is the first tool intended for both real-time CGMs and BGMs.
Reference:
Klonoff DC, et al. J Diabetes Sci Technol. 2014;doi:10.1177/1932296814539589.