Future of AI could mean streamlined clinical care, better connection with patients
Use of AI in medicine is growing quickly. Clinicians are eager to learn what the technology can do, and speakers at meetings across specialties are envisioning its effects — good and bad — in practice management and patient care.
One of the biggest advantages of AI is its ability to crunch data that can lead to better risk prediction for populations and individual patients. The human brain can assess only two or three factors at a time when considering a problem, such as predicting a person’s risk for disease, compared with AI’s much greater ability, according to David C. Klonoff, MD, FACP, FRCP (Edin), Fellow AIMBE, clinical professor of medicine at the University of California, San Francisco, and medical director of the Dorothy L. and James E. Frank Diabetes Research Institute of Mills-Peninsula Medical Center.
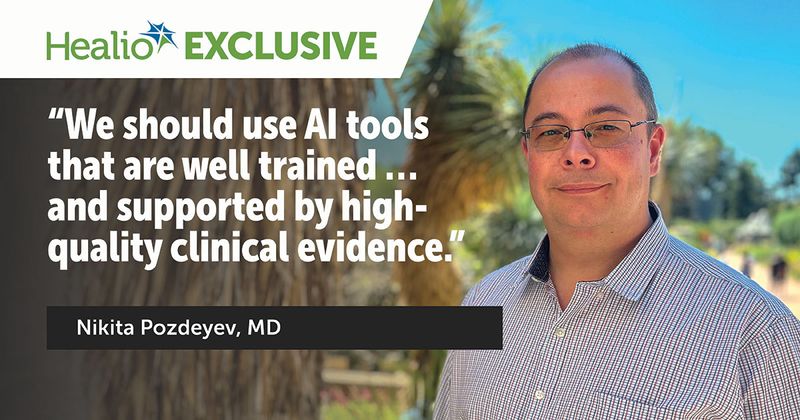
“AI draws conclusions from data based on various probabilities, similar to how we already use our brains to draw relationships,” Klonoff told Healio | Endocrine Today. “But AI can handle much more complicated types of relationships, larger amounts of data and larger numbers of factors that go into deciding on a relationship.”
AI decision tools may one day make use of patient data to allow clinicians to personalize care.
AI applications are currently being used in wearable medical devices. For people with diabetes, for example, AI applications are integral to insulin delivery systems — insulin pumps “talking to” continuous glucose monitors and responding in real time.
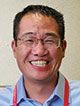
AI is also reading medical images at least as accurately as technicians and sometimes better. Edward C. Chao, DO, clinical professor of medicine at the University of California, San Diego, and a Healio | Endocrine Today Editorial Board Member, said AI models are also being trained to detect complications, such as diabetic retinopathy, by analyzing images.
The use of AI in medicine is being embraced by patients, according to a recent survey conducted at The Ohio State University. In the survey, 52% of respondents said they would be open to using AI as part of care, 71% stated they were comfortable with AI improving the speed and accuracy of a diagnosis and 64% said AI could improve efficiency in health care.
AI has potential to augment human judgment in medicine, but several barriers keep it from being implemented widely. One of those barriers is a lack of high-quality studies validating the benefits of AI in clinical care, according to Nikita Pozdeyev, MD, assistant professor of biomedical informatics at University of Colorado Anschutz Medical Campus.
“We should use AI tools that are well trained, well validated and supported by high-quality clinical evidence,” Pozdeyev said. “This will ensure we actually benefit from the AI instead of using it recklessly and potentially making the wrong decisions.”
As AI plays an increasingly larger role in medicine, stakeholders must remember how people fit into the equation, Chao said. Health care providers must be trained to use AI applications and to ensure AI is used as a tool — not as a replacement — for clinical judgment.
“We don’t want to lose the human in all of these advances,” Chao said. “Sometimes, people focus on technological wonders and changes, but we want to make sure we make the technology works for us and not the other way around.”
AI in clinical practice
One of the most impactful uses of AI could be employing models that can quickly analyze a patient’s medical records and come up with a disease prognosis or treatment plan, according to Klonoff. The introduction of electronic health records ushered in a new era of medical care with the potential to gather information on specific subgroups of people. However, sorting through and analyzing that volume of data is too much for providers to handle on their own.
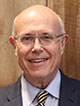
“When you have the application of AI, you’re looking at dozens or even hundreds of different factors that go into a decision, whether you are predicting a problem or you are looking at a treatment,” Klonoff said. “When you put all of those together, you can come up with a very specific description of who this person is and with specific features of treatments.”
AI models are being developed to do more than provide an answer to a simple question, which can increase confidence in using AI. During a plenary session at ENDO 2024, Su-In Lee, PhD, professor in the Paul G. Allen School of Computer Science and Engineering at University of Washington, described explainable AI, a concept in which models will not only be able to make disease predictions, but also to give a short summary of how specific clinical decisions were arrived at.
“The idea of explainable AI is to explain the outcome of the ‘black box’ of the machine learning model based on the contributions of individual features,” Lee said.
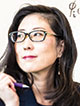
Currently, AI is being used to analyze images to identify diseases such as diabetic retinopathy and thyroid cancer. Machine learning models learn to detect disease by being trained on thousands of images. For thyroid cancer, Pozdeyev said, algorithms help providers determine whether a thyroid nodule is at low or high risk for malignancy, allowing for more-informed decision-making regarding treatment. He said the models can take away some of the subjectiveness of assessing images.
“Using a computer to estimate the risk of a nodule, to analyze those images and give recommendations is a very attractive use, because there’s no intercomputer variability,” Pozdeyev said. “It would give you a consistent answer.”
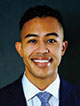
AI could be a factor in many different parts of a clinic visit. During a keynote talk at the American Association of Clinical Endocrinology annual meeting, Dereck Paul, MD, co-founder and CEO of Glass Health, described several clinical decision support tasks that AI can perform for physicians, including developing treatment plans, providing recommendations for prevention, designing and performing patient education and acting as a digital scribe.
“I’m optimistic, and we’ll see this play out over time, that large language models can actually help provider efficiency,” Paul said. “You can imagine completing patient encounters faster with decreased documentation burden.”
In fact, Pozdeyev said, the advent of a digital scribe may be one of the most beneficial ways AI can assist providers.
“The way I see it working is there is a voice recognition system [that] would listen to the interaction of the provider and the patient, convert the recording using voice recognition technology to text, analyze the text and summarize it,” Pozdeyev said. “That’s a generative AI application [that], at the end of the visit, would already have a summary of what has been discussed and what decisions have been made. It is not going to be perfect, especially early in the process of implementing a system like this. That’s why the physician would go through it and edit it as needed. But it would significantly facilitate the process of documenting the visit.”
Using AI to improve human connections
Using AI as a clinical support tool could improve quality of life for providers and improve interactions with patients, according to some experts.
During a keynote lecture at the AACE annual meeting, Helen Riess, MD, a part-time associate professor of psychiatry at Harvard Medical School and founder and chief medical officer at Empathetics, spoke about the importance of empathy in medicine. Providers are feeling burned out due to workload, documentation and regulatory requirements, and constantly learning to use new technologies. Allowing AI to take over some of those tasks could reduce burnout and build stronger health care teams that focus on empathetic care, according to Riess.
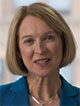
“I think the future is going to benefit from the efficiency of practice by implementing the assistance of AI,” Riess said. “Taking the burden of documentation off the clinician is going to help physicians reconnect with patients. This will establish meaningful relationships that will improve collaboration and health outcomes because patients value the human connection, which motivates them to participate more fully in their health care.” A study published in JAMA Internal Medicine in 2023 examined whether AI might have a role in patient communication. In a cross-sectional study, evaluators compared physician responses to medical questions on a social media forum with those from an AI chatbot. Most patient participants preferred chatbot responses to those from physicians. Additionally, 45% of AI responses were rated as empathetic or very empathetic compared with 4.6% of the responses from physicians.
Despite those findings, AI is not likely to replace human providers, Chao and Pozdeyev said. One of the goals of using AI to perform clinical support tasks is to allow providers to spend more time with patients, according to Chao.
“AI could free up [time] for what we do best — as far as using our creativity or bringing out compassion,” Chao said. “I don’t get a sense that AI will overshadow humans in terms of creativity, higher decision-making, compassion or empathy.”
Pozdeyev expressed skepticism about the long-term role of chatbots in medicine. Chatbots such as ChatGPT have been at the forefront of AI discussion during the past few years, but a number of limitations prevent them from replicating the interaction between patients and providers, Pozdeyev said.
“Even if we develop a chatbot that works reasonably accurately — and that’s possible, it’s just a question of resources — this will never replace a clinic visit,” Pozdeyev said. “When I see a patient, it’s not simply delivering information or prescribing some course of action. It’s a human interaction.”
Until a fully functional generative AI application is developed, a human provider will be required to make clinical decisions, Pozdeyev said.
AI and disparities
Even if AI brings several benefits to medicine, the technology must be used equitably. Chao said existing health disparities could worsen if an AI model is trained on data that do not represent diverse populations.
“If you have folks who are not represented, what you’re going to get [from AI] is not necessarily going to reflect that [population],” Chao said. “The issues would further exacerbate inequities that we already see in medicine.”
Disparities go beyond the patient population, Klonoff said. AI may cause a massive shift in health care where the hospitals and health systems with the most patient data have the best AI prediction models.
There are strengths and weaknesses to the various directions health systems can go with AI, according to Klonoff. Systems that use their own data to inform AI may create a model that cannot be applied to other patient populations with different demographics. Similarly, smaller health systems may not have enough data to adequately inform an AI model.
“Smaller hospitals don’t have as many resources as larger hospitals,” Klonoff said. “High-quality AI software is going to become one of those resources. That’s going to be expensive, and a small, struggling hospital might not be able to afford the best AI.”
One solution for smaller institutions could be to form networks or partnerships to build larger datasets for AI applications and to share expenses, according to Klonoff.
Looking ahead
AI is rapidly developing in medicine, but high-quality research confirming the benefits of AI is limited, according to Pozdeyev. The lack of research could slow adoption of AI in medicine.
“In medicine, we are very conservative for good reasons, because stakes are high,” Pozdeyev said. “For the clinical community to accept a tool, we want high-quality evidence. We don’t have that for thyroid AI and for thyroid nodule classification. ... What we need is to evaluate the [AI] application in a prospective, large multicenter clinical trial [that] is done well and published in a high-impact clinical journal.”
Regulatory standards for AI are also lacking, according to Chao. Standards need to be established by an agency such as the FDA, he said. Stakeholders from across medicine — researchers, clinicians, other medical staff, patients and caregivers — must have a voice and everyone’s concerns addressed.
“One of my interests is in applying human-centered design to technology, trying to make it easier for patients with diabetes or the people that care for them,” Chao said. “Trying to include that would be important, because a lot of times [an application] has already been developed, and we may not have made things more accessible or easier to use.”
As AI becomes used more widely, providers will need to adapt, Chao said. One way to introduce AI applications to providers is to implement dedicated training sessions for fellows.
“Ideally, you could have individuals who are on the front lines of designing AI teaching clinicians,” Chao said. “Then it could be a two-way street.”
Klonoff said the ability to use AI applications will become a mandatory skill for providers.
“AI will not replace doctors,” Klonoff said. “Doctors who use AI will replace doctors who don’t use AI.”
Chao, Klonoff and Pozdeyev all said exactly how AI will affect medicine in the future is hard to envision because of how rapidly the field is moving. However, all three agreed that AI will be an integral part of medicine.
“We’re now living in the era of the Internet of Medical Things,” Klonoff said. “Various sensors are being used, especially wearable sensors, and we’re going to see information assembled from a variety of sources that we’ve never seen before.”
- References:
- Ayers JW, et al. JAMA Intern Med. 2023;doi:10.1001/jamainternmed.2023.1838.
- Lee S, et al. Abstract PL02. Presented at: ENDO annual meeting; June 1-4, 2024; Boston.
- Riess H, et al. Keynote: The art and science of empathy and artificial intelligence accountability. Presented at: American Association of Clinical Endocrinology Annual Scientific and Clinical Conference; May 9-11, 2024; New Orleans.
- Survey finds most Americans are comfortable with AI in health care. https://wexnermedical.osu.edu/mediaroom/pressreleaselisting/most-americans-comfortable-with-ai-in-health-care. Published Aug. 21, 2024. Accessed Aug. 23, 20204.
- For more information:
- Edward C. Chao, DO, can be reached at ecchao@health.ucsd.edu.
- David C. Klonoff, MD, FACP, FRCP (Edin), Fellow AIMBE, can be reached at dklonoff@diabetestechnology.org.
- Su-In Lee, PhD, can be reached at suinlee@cs.washington.edu.
- Dereck Paul, MD, can be reached at enterprise@glass.health.
- Nikita Pozdeyev, MD, can be reached at nikita.pozdeyev@cuanschutz.edu.
- Helen Riess, MD, can be reached at hriess@mgh.harvard.edu and hriess@empathetics.com; LinkedIn: www.linkedin.com/in/helen-riess.