Advances in AI could improve disease prediction, reduce provider stress
Click Here to Manage Email Alerts
Key takeaways:
- Explainable AI can examine multiple health features and give providers a detailed prognosis of a patient.
- AI may reduce stress by examining electronic health records, assisting with manuscript writing and more.
BOSTON — Explainable AI and systems that help providers with day-to-day tasks will allow experts to conduct more advanced research and make impactful changes in clinical care, according to two speakers at ENDO 2024.
AI is already used throughout health care, and researchers are taking the next steps toward enhancing machine learning even further. During a plenary session, Su-In Lee, PhD, a professor in the Paul G. Allen School of Computer Science and Engineering at University of Washington, said future AI models will not just provide answers, but also explain how it came to certain decisions.
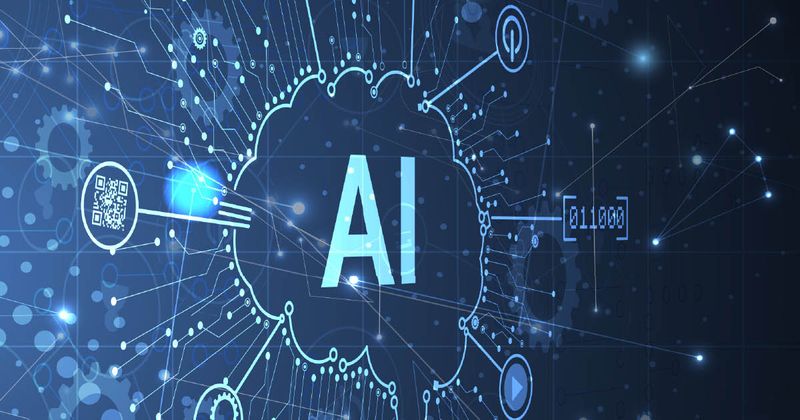
Casey Greene, PhD, director of the Center for Health AI and interim director of the Colorado Center for Personalized Medicine at University of Colorado, said AI can be used as a companion tool in clinical practice and research.
“There are a lot of opportunities for AI,” Greene said during a presentation. “We can think about how it can deliver serendipity at scale across many areas. We can imagine more equitable, effective health care that prevents physician burnout, improves our process of research and then advances our own informatics approaches to research.”
Advantages of explainable AI
AI is currently capable of examining clinical data, test results, demographics and more to make a disease prediction. However, Lee said, this type of machine learning produces only an answer and does not dive deeper into how it came up with its answer.
“The idea of explainable AI is to explain the outcome of the ‘black box’ of the machine learning model based on the contributions of individual features,” Lee said during a presentation.
The concept of explainable AI has been assessed in several studies. In a paper published in The Lancet Healthy Longevity in 2023, researchers used explainable AI to determine a person’s biological age, which differed from a person’s actual chronological age based on their demographic, health records, lab tests and lifestyle.
Explainable AI can also be used to audit other AI models. Lee discussed a study published in Nature Machine Intelligence in 2021 assessing how AI models detect COVID-19 based on chest imaging. The models used key factors detected in the images instead of genuine pathology, which could lead to misdiagnoses, Lee said. Explainable AI can use a saliency map to highlight the most important features in an image to improve COVID-19 prediction.
Explainable AI could also lead to cost-aware AI designed to reduce time, money and other resources in treating patients, according to Lee. A study published in Nature Biomedical Engineering in 2022 compared a cost-aware AI model with the Perceived Ability to Cope with Trauma (PACT) scale for predicting acute traumatic coagulopathy risk among people admitted to a trauma center. The AI model was just as accurate for predicting acute traumatic coagulopathy risk as PACT and was able to determine risk in less than 1 minute, whereas conducting the PACT scale took about 8 minutes.
“This is a general AI model that can be applied to other diseases,” Lee said. “[Cost-aware] AI can do better than many other existing clinical [risk] scores.”
AI in clinical care, research
AI will have a large impact in multiple realms of health care, according to Greene. One of the biggest uses of AI could be its ability to quickly review electronic health records. Greene discussed a survey of health care providers that found the majority of respondents said EHRs add frustration to their day and lower job satisfaction. He said using AI to work with EHRs could reduce burnout among physicians.
AI could be used to help providers communicate with patients. A study published in JAMA Internal Medicine found that among responses to patient questions on a social media website, 45.1% of responses from an AI chatbot were rated as empathetic or very empathetic compared with 4.6% of those written by physicians.
“What the chatbot can do is it can help providers to deliver more empathy to their patients,” Greene said. “There are systems developed and deployed at many health systems that can support automated drafting of potential provider responses to patient messages.”
AI could assist with other forms of writing as well. Greene discussed a system capable of suggesting revisions and automatically correcting proofreading mistakes in manuscripts. He said large language models could be used by researchers to draft and edit studies.
Despite these advances, AI still struggles to perform some tasks, such as identifying a specific image, Greene said, so expert human knowledge will continue to be a crucial part of AI.
“Predictions can be brittle,” Greene said. “It’s really important to think about how you’re going to put human factors in the loop that give you the robustness that you need.”
References:
Ayers JW, et al. JAMA Intern Med. 2023;doi:10.1001/jamainternmed.2023.1838.
DeGrave AJ, et al. Nat Mach Intell. 2021;doi:10.1038/s42256-021-00338-7.
Erion G, et al. Nat Biomed Eng. 2022;doi:10.1038/s41551-022-00872-8.
Qiu W, et al. Lancet Healthy Longev. 2023;doi:10.1016/S2666-7568(23)00189-7.