Machine learning AI may enhance automated insulin delivery for type 1 diabetes
Key takeaways:
- Automated insulin delivery can be controlled by a neural network that learns using a saturated dataset.
- A neural-network artificial pancreas performed similarly to a control algorithm in a small clinical trial.
An automated insulin delivery system controlled by an artificial intelligence machine learning model may perform just as well as a system using a traditional equation-based insulin dosing algorithm, according to a speaker.
Since the invention of continuous glucose monitoring, researchers have been able to gather real-time data for people with type 1 diabetes that was previously unavailable, according to Boris Kovatchev, PhD, director of the University of Virginia Center for Diabetes Technology and professor at the University of Virginia School of Medicine and School of Data Science. These data can be used to train a neural network, a type of AI machine learning. Someday soon, a neural-network artificial pancreas, or “NAP,” could be utilized by people with type 1 diabetes, Kovatchev said during a presentation at the International Conference on Advanced Technologies & Treatments for Diabetes.
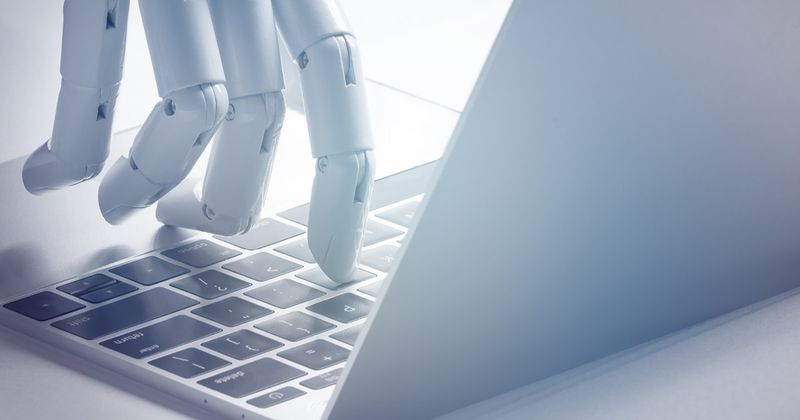
“NAPing is a process by which any insulin dosing rule can be encoded in a neural network, regardless of how it’s formulated,” Kovatchev said. “In particular, an automated insulin delivery algorithm can be encoded in a neural network.”

A neural-network artificial pancreas is trained using a saturated dataset, which covers all possible actions that an automated insulin delivery control algorithm may perform, according to Kovatchev. He said the aim is for the neural-network artificial pancreas to be able to implement an insulin dosing rule at any level of precision.
Researchers developed a neural-network artificial pancreas that was trained on a dataset and is being assessed in two clinical trials. Kovatchev said the neural-network artificial pancreas was approved by the FDA for clinical trials in humans.
Neural network in hybrid-closed loop system
In the first clinical trial, researchers compared the performance of the neural-network artificial pancreas with a hybrid closed-loop system’s normal insulin delivery algorithm. The study included 15 adults with type 1 diabetes who used a Tandem t:slim X2 insulin pump with ControlIQ technology. Participants were randomly assigned to have their device controlled using the neural-network artificial pancreas or the University of Virginia model-predictive control algorithm at a hotel for 18 hours. After a between-session period of 0 to 4 weeks, participants crossed over to the other arm.
The neural-network artificial pancreas conferred a similar decline in mean glucose and a similar increase in time in range as the control algorithm. While using the neural-network artificial pancreas, participants had a mean glucose of 130 mg/dL and a time in range of 86.1% during the hotel session.
Participants had higher mean glucose during dinner time with the neural-network artificial pancreas compared with the control algorithm, Kovatchev said, but they maintained similar glucose levels during the same time of day between the two arms.
The two algorithms ran in parallel on the same device during the study, with one algorithm controlling the insulin delivery system and the other sitting in the background. Kovatchev said the neural-network artificial pancreas and control algorithm behaved the same way when given the same data to work with. However, the neural-network artificial pancreas had a sixfold lower processing time than the control algorithm.
“Maybe [the neural-network] approach is more useful for small devices with low processing power than model-predictive control algorithms such as insulin pumps,” Kovatchev said.
Early data on fully closed-loop mode
Kovatchev also presented preliminary data from the first six adults who completed a clinical trial assessing the performance of the neural-network artificial pancreas in fully closed-loop mode. After 2 weeks of usual care at home, participants used the neural-network artificial pancreas in fully closed-loop mode for 5 days at a hotel. The neural-network artificial pancreas was then used at home for 1 week. Meals and physical activity at home were not standardized, and participants were remotely monitored at all times with automated notifications enabled.
The first six participants in the study had a time in range of 72% with the neural-network artificial pancreas compared with a 68% time in range with usual care. During the neural-network artificial pancreas portion of the study, time in range was 73.7% during the hotel session and 72.3% at home.
“We are happy with both results because in hybrid mode, it got about 87% time in range, and in full closed-loop mode, it got 72%,” Kovatchev said. “Maybe that’s what we are going to expect in the future when we transition to fully automated closed-loop. Low-to-mid 70s [time in range] may be a benchmark to try and achieve.”
Reference:
Kovatchev BP, et al. Diabetes Technol Ther. 2024;doi:10.1089/dia.2023.0469.