'The more data ... the better': AI poised to alter diabetes care
Click Here to Manage Email Alerts
Artificial intelligence is not yet a routine fixture in medicine, but it may soon change how physicians approach medical care, including prediction and diagnosis of diabetes complications.
Using AI, a health care provider can combine large data sources to capture a picture of where a patient is at a certain point in time, identify outcomes of those with particular combinations of data and then calculate the likelihood that an individual patient will develop a specific condition or complication, according to David C. Klonoff, MD, FACP, FRCP (Edin), Fellow AIMBE, clinical professor of medicine at the University of California, San Francisco, and medical director of the Diabetes Research Institute at Mills-Peninsula Medical Center.
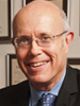
“The idea is that the information is so detailed and you have access to so many patients in your large database that you can make a good prediction of what’s going to happen next,” Klonoff told Healio.
AI plays a pivotal role here: Whereas individual physicians can analyze relatively small amounts of information, AI can handle large quantities of data from many sources. This ability is why it may prove so game-changing in the realm of diabetes care.
“It’s not that the conclusion is beyond what the human brain can come up with. In fact, humans could typically come to the same conclusion. However, there are so many combinations of data and, in some cases, the relationships are very small. To add up all of those relationships, a human would need months or even years to reach a conclusion,” Klonoff said. “So, while in theory, the human brain could do this, the practical situation is that for a given patient, the physician does not have months or years to reach a conclusion. They want to make that prediction immediately, and for that, we have computers and the processing activity named AI.”
A multitude of data
When considering AI in diabetes care, a number of data sources are available, including genetics, genomics, blood tests, medical records, pharmaceutical records and insurance claims, according to Klonoff.
For diabetes in particular, Klonoff noted, data on metabolism from wearables, such as continuous glucose monitors or exercise monitors, and physiological monitoring of the cardiovascular system with rhythm strips, wearable blood pressure devices or respiration trackers, are also important. Additionally, information on behavior and environment should be considered.
Although the amount of data can be daunting, having multiple sources of information can mitigate the burdens placed on the patient and physician, Klonoff noted. Genetic and genomic data offer insight from two different perspectives about a patient’s susceptibility to a specific condition while blood tests can diagnose different diseases or raise red flags about future health problems. Insurance claims can fill in gaps in a patient’s medical history and pharmaceutical data can provide information on treatment adherence.
“Each piece of information by itself might only translate to a slight difference in risk, perhaps only increasing it by 1%. However, if something else increases risk by another 1% and so on, it can actually become a meaningful number,” Klonoff said.
To make a prediction, AI compares information from a specific patient with that of patients with similar characteristics. In this case, the goal is to match the patient as closely as possible to others within the data set.
“The more you can define who your patient is, the better you can make a comparison,” Klonoff told Healio. “When doctors do studies using AI, they try to characterize each of the patients as specifically as possible so that they really are comparing people who are very similar in their risk.”
One glitch in this system, however, is that real-world data are not only retrospective in nature but also often not on par with the quality found in a randomized clinical trial. In such trials, the data are collected more meticulously. In contrast, the electronic health record, which would largely supply the information used by AI in routine clinical practice, was designed to treat patients, not for research purposes, and therefore may be incomplete, Klonoff noted.
Even so, randomized clinical trials look at very specific questions, which complicates extrapolation of their conclusions.
“Generally, when you compare your patient with data from a randomized clinical trial, you’re going to find maybe hundreds of patients with whom to compare them, but if you get access to charts with lower-quality real-world evidence, you may be able to compare your patient to millions of other patients. Sometimes, the quantity may make up for the lack of quality,” Klonoff said.
When used in the context of AI, images can also prove important for diagnosing and predicting complications of diabetes, particularly diabetic retinopathy, diabetic peripheral neuropathy and diabetic foot ulcers. For these conditions, the pattern recognition — a form of AI known as machine learning — involved in diagnosis or prediction can sometimes rely on analysis of 2D images, according to Klonoff.
“Pattern recognition is useful for making diagnoses of conditions where a picture is involved, such as imaging the retina to look for retinopathy, looking at a skin biopsy to see if there’s a loss of nerves indicating neuropathy or pictures of feet to detect diabetic foot ulcers,” Klonoff said.
Refining diagnosis, prediction of diabetes complications
Researchers are examining AI’s potential to improve prediction and diagnosis of a number of diabetes complications, according to a commentary published by Cynthia Huang and colleagues in the Journal of Diabetes Science and Technology in 2022.
Although not quite mainstream, the most significant strides have arguably been made in the use of AI for diagnosing diabetic retinopathy. In 2018, the FDA granted clearance to IDx-DR (IDx LLC), a software program that uses an AI algorithm to analyze images of the eye taken with a retinal camera and provides a physician with results. It was the first device authorized for marketing that yielded a screening decision without the need for a clinician to also interpret the image or results, according to an agency press release. In 2020, EyeArt AI diagnostic system (Eyenuk Inc.), another autonomous AI system, also received FDA clearance for diagnosing diabetic retinopathy. Most recently, in 2022, AEYE Health (AEYE Health Inc.) received FDA clearance to market an AI-based screening system that diagnoses retinopathy.
Several studies, including one published in JAMA Network Open in 2021 evaluating the EyeArt system, indicated that the sensitivities and specificities of many AI systems for diagnosing diabetic retinopathy were high for the detection of referable diabetic retinopathy. However, one study published in Diabetes Care in 2021 showed that sensitivities and specificities of seven automated AI-based diabetic retinopathy screening systems varied significantly when using real-world retinal imaging data.
Questions about how the overall conclusions are drawn remain, according to Huang and colleagues.
“Because of the black box nature of deep learning algorithms, it can be difficult to understand which specific features of a fundus image contribute to the determination of a retinal grade,” they wrote. “Active research is being conducted to address these concerns, such as explainable AI programs that annotate the lesions and regions of interest and AI programs that detect comorbidities from retinal images, such as CVD, nephropathy and neuropathy.”
Diabetic foot ulceration is another area that has seen some success with AI. Specifically, several machine learning algorithms have demonstrated the ability to detect diabetic foot ulcers in images. For example, in a study published in the IEEE Journal of Biomedical and Health Informatics in 2019, researchers developed a deep learning model — another aspect of machine learning — using a database of images that performed relatively well for diabetic foot ulcer localization.
Prediction of diabetic foot ulcers before actual development, which would be a major win, has remained somewhat elusive. However, one study published in Vascular in 2021 showed that an AI algorithm created using six risk factors for diabetic foot ulcers predicted the likelihood of developing the condition with nearly 80% accuracy.
AI has also been studied for diagnosis of diabetic nephropathy, with several studies indicating that use of AI in addition to clinical data performed well compared with traditional risk scores for outcomes in patients with the disease. For example, one study published in Diabetologia in 2021 showed that a machine-learned prognostic risk score improved prediction of kidney outcomes when compared with other clinical models in patients with early-stage diabetic nephropathy.
Researchers are also turning to machine learning to improve early diagnosis of diabetic peripheral neuropathy. According to Huang and colleagues, qualitative, quantitative physiological and anatomical tests can use AI to diagnose diabetic neuropathy. The authors noted, for instance, that researchers have developed an algorithm that uses major clinical factors to classify painful and nonpainful neuropathy, and others have connected a vibration perception threshold sensor to a machine learning algorithm that incorporates patient clinical characteristics for risk assessment of diabetic neuropathy. Additionally, deep learning algorithms involving anatomical imaging, including MRI and corneal confocal microscopy, are showing promise for diagnosing the condition.
“Continued development of these AI-based algorithms is predicted to play a central role in diabetic peripheral neuropathy diagnosis as clinical diagnostic aids for clinicians or as an online risk or diagnosis calculator for patients,” Huang and colleagues wrote.
Future of AI in medicine
Although AI has made inroads into many fields, its incorporation into routine medical practice has lagged, largely because the quantity of data required to power the process did not exist before implementation of the EHR. As health care systems finally caught up, the EHR hit its stride and opened the door for use of AI in medicine, according to Klonoff.
In addition to the information generated by the EHR, companies are now assembling data from many different hospitals and making data sets available for purchase. These give physicians and researchers access to de-identified data on millions of patients, as compared with the thousands contained in one hospital system, which will allow further refinement of AI for prediction, diagnosis and treatment of various medical conditions, Klonoff noted.
Although these massive amounts of data have significant benefit, there are some drawbacks, according to Klonoff. First, more information translates to more combinations and possible outcomes, which requires complicated software that may not exist yet. Second, highly detailed characterization of patients is important for creating algorithms that can speak to the outcomes of a specific patient, but making someone too unique can also prove problematic.
“If you drill down too much, then you have nobody to compare that patient with,” Klonoff told Healio. “We have to figure out how deeply to drill before we’re actually hurting ourselves.”
In looking at what could help elevate AI, Klonoff said biobanks could be integral to providing more insight into diseases and conditions and their associations with clinical data.
“If somebody provides a blood sample to a biobank, then we can do a whole lot of tests, including those for certain conditions or genomic tests for things that do not even necessarily have apparent clinical value. We could even do tests someday that don’t yet exist,” Klonoff said.
Currently, though, physicians and researchers have a wealth of information at their disposal and should continue to use it to search for ways to not only diagnose but also predict and prevent diseases.
“The more data that we can collect, the better we are able to predict outcomes from where we are now,” Klonoff said. “Part of medicine is predicting what’s going to happen next. Of course if it’s an adverse outcome, then we want to predict what we can do to prevent that from happening. And we can make better predictions by having more data and employing technology like AI.”
References:
- Baskozos G, et al. BMC Med Inform Decis Mak. 2022;doi:10.1186/s12911-022-01890-x.
- Chan L, et al. Diabetologia. 2021;doi:10.1007/s00125-021-05444-0.
- Dubey VN, et al. J Diabetes Sci Technol. 2022;doi:10.1177/1932296820965583.
- Eyenuk. Eyenuk announces FDA clearance for EyeArt autonomous AI system for diabetic retinopathy screening. https://www.eyenuk.com/us-en/articles/diabetic-retinopathy/eyenuk-announces-eyeart-fda-clearance. Published Aug. 5, 2020. Accessed April 8, 2023.
- FDA. FDA permits marketing of artificial intelligence-based device to detect certain diabetes-related eye problems. https://www.fda.gov/news-events/press-announcements/fda-permits-marketing-artificial-intelligence-based-device-detect-certain-diabetes-related-eye. Published April 11, 2018. Accessed April 7, 2023.
- Goyal M, et al. IEEE J Biomed Health Inform. 2019;doi:10.1109/JBHI.2018.2868656.
- Huang J, et al. J Diabetes Sci Technol. 2022;doi:10.1177/193229682211245.
- Ipp E, et al. JAMA Netw Open. 2021;doi:10.1001/jamanetworkopen.2021.34254.
- Lee AY, et al. Diabetes Care. 2021;doi:10.2337/dc20-1877.
- Preston FG, et al. Diabetologia. 2022;doi:10.1007/s00125-021-05617-x.
- Stefanopoulos S, et al. Vascular. 2021;doi:10.1177/17085381211040984.
- Teh K, et al. Diabetologia. 2021;doi:10.1007/s00125-021-05416-4.