Precision medicine app can help improve individualized treatment, diabetes outcomes
PHILADELPHIA — An interactive, data-driven tool can individualize care for people with type 2 diabetes while promoting shared decision-making between clinicians and patients, according to a speaker at the Heart in Diabetes CME conference.
Type 2 diabetes is highly heterogenous with a wide range of risk factors, pathophysiological pathways and prognoses, and many therapeutic options, Richard E. Pratley, MD, the Samuel E. Crockett Chair in Diabetes Research and medical director of AdventHealth Diabetes Institute, said during a presentation. Current standards of care in type 2 diabetes promote individualization of treatment, which should account for individual patient characteristics, comorbidities, preferences and goals. However, data to guide selection of a treatment for an individual patient are not always available, Pratley said.
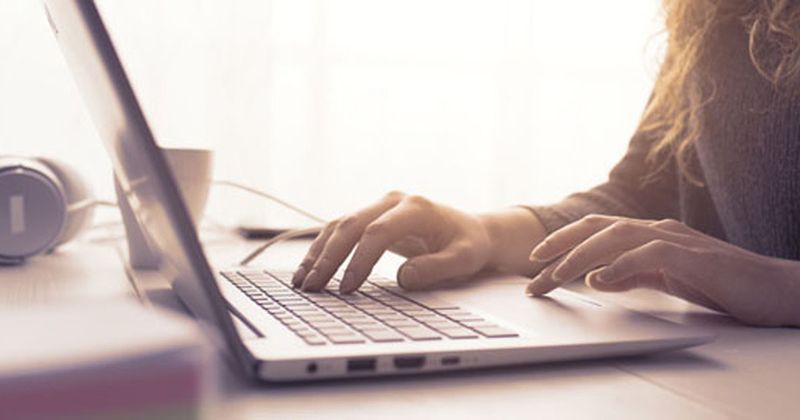
“We have a lot of work to do to advance precision therapeutics,” Pratley told Healio. “One of the biggest barriers will be provider engagement and implementation of advanced decision support as a routine in clinical practice. The implementation science is where we need a lot of work.”
Identifying predictors of response

The American Diabetes Association and the European Association for the Study of Diabetes identified the need for decision-support tools to implement precision diabetes treatment in clinical practice as a key research gap as part of the new Precision Medicine in Diabetes Initiative, Pratley said.
“How are we going to do this?” Pratley said during his presentation. “How are we going to take clinical information and translate it into something that guides therapy in a way to maximize treatment efficacy and reduce side effects?”
In a study published in Pharmaceutical Medicine in 2019, Pratley and colleagues identified patient characteristics associated with achieving and maintaining a target HbA1c of less than 7% using machine learning methodology to analyze data from two phase 3 randomized controlled trials of empagliflozin (Jardiance, Boehringer Ingelheim/Eli Lilly) and linagliptin (Tradjenta, Boehringer Ingelheim/Eli Lilly) single-pill combination therapy (Glyxambi) compared with monotherapy in people with type 2 diabetes. The machine learning analysis identified HbA1c and fasting plasma glucose as the strongest predictors of attaining glycemic control; covariates including body weight, waist circumference, blood pressure or other variables did not contribute to the outcome.
“At the end of the day, it turns out that HbA1c and fasting glucose are your predictors of a response. That was not super helpful and did not really advance precision medicine much,” Pratley said.
Insight from large databases
To gain better insight, Pratley and colleagues developed an interactive, data-driven online tool, using data from more than 30,000 participants from several large Novo Nordisk-sponsored clinical trials. The goal, he said, was to to “bridge the gap” between trial evidence and decision-making in clinical practice.
“The goal of this exercise was to dig into a large dataset and try to understand what factors were important to get to individualized predictors of response — not average predictors of response,” Pratley said.
To predict individual outcomes, specific patient baseline characteristics and the treatment option of interest — as an example, a GLP-1 receptor agonist — are selected from sliding scales and drop-down menus; the tool then automatically selects the most appropriate randomized clinical trial from the database.
The totality of trial data is used to generate estimated coefficients for each baseline characteristic such as age and body weight; predicted outcomes are based on specific randomized controlled trials. Some trials were placebo-controlled; others had an active comparator.
“The idea was to take things like age, sex, BMI, diabetes duration, current treatment, renal function ... and put that into this algorithm and then spit out what the expected responses would be, not to a variety of drugs, but to one drug,” Pratley said. “The idea is to sit down with the patient and enter information into the tool and say, ‘We predict this [drug] may lower your HbA1c by 1.2% and your body weight by 5 lb.’”
More work needed
An advantage of the decision-support tool is that it utilizes an individual’s characteristics rather than aggregate trial data, encouraging participation of the patient and the provider, Pratley said. Pratley noted, however, that the inherent bias in randomized controlled trials limit the generalizability of the predicted outcomes. Additionally, the current version of the tool does not allow direct comparisons between treatments.
“No one is going to use a tool developed by one company; it needs to be more generalized,” Pratley said. “So, where does this fit on the roadmap? Now, we are at a stage where we can translate these things into the clinic with more precision therapies. The real challenge is to get the implementation in clinical practice.”
References:
- Buse JB, et al. Diabetes Obes Metab. 2021;doi:10.1111/dom.14381.
- Del Parigi A, et al. Pharmaceut Med. 2019;doi:10.1007/s40290-019-00281-4.