Machine learning, AI models could pinpoint novel fracture risk factors
Machine learning models are increasingly used for a deeper investigation of osteoporosis, including diagnosis and fracture prediction, but technical and clinical concerns about use of such methods remain, data from a recent review show.
“We are still in the early phase of machine learning in the bone field,” William D. Leslie, MD, MSc, FRCPC, professor of medicine and radiology at the University of Manitoba in Winnipeg, Canada, told Healio. “There have been a few products that have been reviewed and approved, but most of the work requires more validation. In our review, we observed a wide range in the quality of what is being done. Using a 12-point checklist, the average score was 6. That means half are failing and half are passing. We need to identify where we can do a better job.”
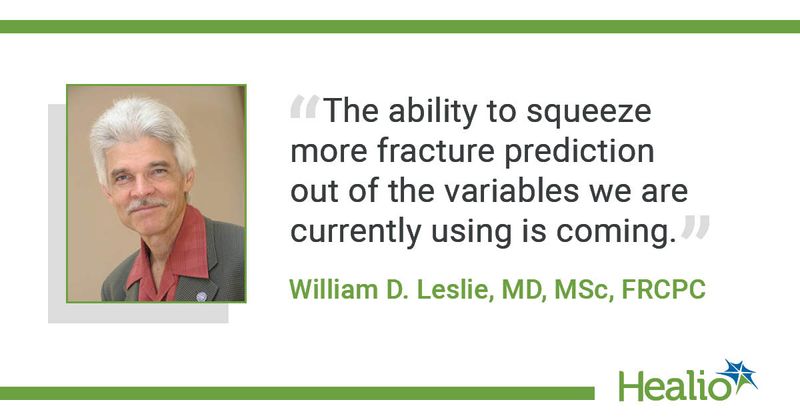
Data quality poor
In a literature review, Leslie and colleagues analyzed 89 articles investigating bone properties assessment (n = 13), osteoporosis diagnosis (n = 34), fracture detection (n = 32) and risk prediction (n = 14), with 78% published after 2018. The three most common data sources were X-rays (33%), database (26%) and CT (20%). DXA modality was studied in only five studies, although some others have used databases with bone mineral density assessed by DXA scans. Almost all studies applied directly to images (n = 26) used deep learning models. Reporting and methodological quality was determined with a 12-point checklist.
“In general, the studies were of moderate quality with a wide range (mode score 6, range 2 to 11),” the researchers wrote. The findings were reported in the Journal of Bone and Mineral Research.
The researchers found “major limitations” across several studies; incomplete reporting, especially over model selection, inadequate splitting of data and the low proportion of studies with external validation were among the most frequent problems.
Researchers found that the use of images for opportunistic osteoporosis diagnosis or fracture detection emerged as a “promising approach” and cited the method as one of the main contributions that machine learning could bring to the osteoporosis field.
“Identification of vertebral fractures is an area where there had been good progress,” Leslie said. “There is a lot of work being done on predicting bone density from non-DXA images, such as CT scans. The ability to squeeze more fracture prediction out of the variables we are currently using is coming.”
Leslie said efforts to develop machine learning-based models for identifying novel fracture risk factors and improving fracture prediction are additional promising lines of research. Some studies also offered insights into the potential for model-based decision-making.
“Though it was not the focus of this work, using genetic risk scores could be invaluable for assessing risk for a whole host of things, not just fracture risk,” Leslie said. “Early generations did not work all that well because we were limited to the number of variations we could look at. Now we have hundreds of thousands of variations. You add on machine learning to identify complex patterns in data that cannot be seen with standard statistical analyses.”
‘Common pitfalls’ of machine learning
“To avoid some of the common pitfalls, the use of standardized checklists in developing and sharing the results of machine learning models should be encouraged,” Leslie and colleagues wrote. Additionally, the researchers noted that adhering to a predefined detailed pipeline for machine learning implementation and reporting are essential for accurately assessing results and their clinical implications.
“Nevertheless, the majority of the studies reviewed in the current article suffered from the lack of a standardized approach in conducting and/or reporting the machine learning methodology,” the researchers wrote. “There is a need for journals to develop and require authors to follow standard checklists as part of the peer-review process.”
Leslie said as machine learning technology advances, the dilemma that will inevitably arise is what to trust: the clinician or the artificial intelligence model.
The trustworthiness of machine learning models largely depends on transparency.
“Machine learning technology is not like typical devices that stay fixed,” Leslie said. “This is technology that can potentially ‘learn’ as you go along. How do you license something that is going to change? People are scratching their heads about that. We do not know. With experience, these algorithms can improve over time and correct errors. We have to find a way to regulate them securely and secure patient information and confidence through approval process.”
For more information:
William D. Leslie, MD, MSc, FRCPC, can be reached at bleslie@sbgh.mb.ca.