AI model predicts gestational diabetes risk in first trimester
Computer models using machine learning and artificial intelligence successfully predicted gestational diabetes status among Chinese women using clinical data before 12 weeks’ gestation, 2 months earlier than previous models, data show.

“Previously when we prevented gestational diabetes, we were only focusing on pregnant women with obesity or pregnant women with a history of gestational diabetes or those with elevated blood glucose during early pregnancy,” He-Feng Huang, MD, PhD, of Shanghai Jiao Tong University School of Medicine and the International Peace Maternity and Child Health Hospital in Shanghai, told Healio. “Now we have expanded the scope of target population through the prediction model, which will enable more high-risk pregnant women to gain potential benefits.”
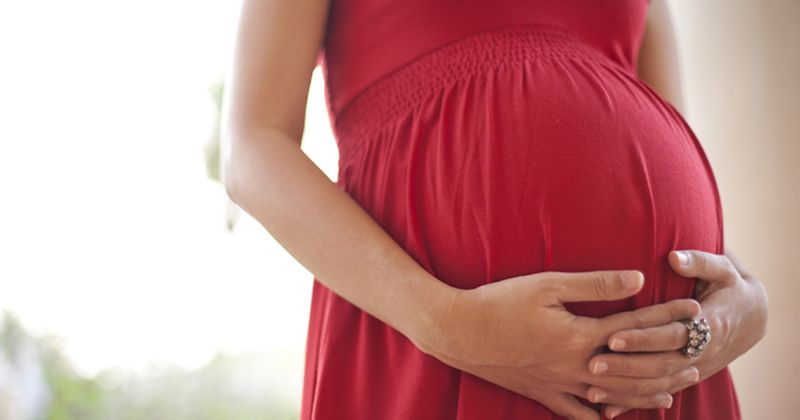
Using electronic medical records data from a 2017 obstetrical database, Wu and colleagues analyzed records from 16,819 women with a diagnosis of gestational diabetes, selecting variables for early gestational diabetes prediction such as sociodemographic characteristics, clinical variables and laboratory indexes during the first trimester. To evaluate the prediction model, researchers then analyzed data from 14,992 women using the 2018 obstetrical EMR, which served as a testing group.
“We used machine learning variable selection methods to screen for risk factors for early development of gestational diabetes,” the researchers wrote. “Of the 73 extracted variables, 17 variables were selected for our models, which included sociodemographic data, clinical characteristics, glucose metabolism, lipid metabolism and thyroid function.”
Of the 17 variables, researchers selected seven based on intra-variable correlation and clinical importance: age, family history of diabetes in a first-degree relative, multiple pregnancies, previous gestational diabetes history, fasting plasma glucose, HbA1c and triglycerides. The researchers used advanced machine learning approaches using the seven-variable data set and the 73-variable data set to build models predicting early gestational diabetes. The findings were published in The Journal of Clinical Endocrinology & Metabolism.
The researchers found that the deep neural network model using 73 variables achieved high discriminative power, with area under the curve values of 0.8. The seven-variable logistic regression model also achieved effective discriminate power, with an AUC of 0.77.
Researchers found that low BMI ( 17 kg/m²) was associated with increased risk for gestational diabetes compared with a BMI in the range of 17 kg/m² to 18 kg/m², the minimum risk interval (11.8% vs. 8.7%; P = .0935).
The model demonstrated that total thyroxine and total triiodothyronine were superior to free T4 and free T3 in predicting gestational diabetes. The model also suggested that lipoprotein(a) was a promising predictive value, with an AUC of 0.66.
“Using a machine learning-based variable selection approach, 17 important gestational diabetes predictive variables were selected,” the researchers wrote. “These 17 indicators are worthy of in-depth study in the gestational diabetes field; in particular, lipoprotein(a) may be closely related with gestational diabetes. A seven-variable linear regression model was developed for more practical clinical applications.”
The researchers noted that further research is required to clarify the relationship among total T4, free T4 and gestational diabetes, as well as the relationship between excessively low BMI and gestational diabetes among Chinese women.
“The effect of the prediction model still needs to be verified by prospective studies,” Huang said. “Now we are conducting a multicenter randomized controlled study based on this predictive model. The research content is mainly to explore whether nutritional intervention can reduce the incidence of gestational diabetes among high-risk pregnant women.”
For more information:
He-Feng Huang, MD, PhD, can be reached at huanghefg@sjtu.edu.cn.