AI model accurately predicts atrial fibrillation within 1 month of sinus rhythm
Key takeaways:
- Artificial intelligence accurately predicted atrial fibrillation within 31 days from outpatient sinus rhythm ECGs.
- Tools such as this could be used as a way to manage CV conditions preemptively.
With nearly 90% accuracy, an artificial intelligence-based model consistently predicted atrial fibrillation within 31 days among patients with sinus rhythm, according to findings published in JAMA Cardiology.
The model was calibrated and trained using ECG data from multiple Veterans Affairs sites and externally validated using ECG data from Cedars-Sinai, according to the study.
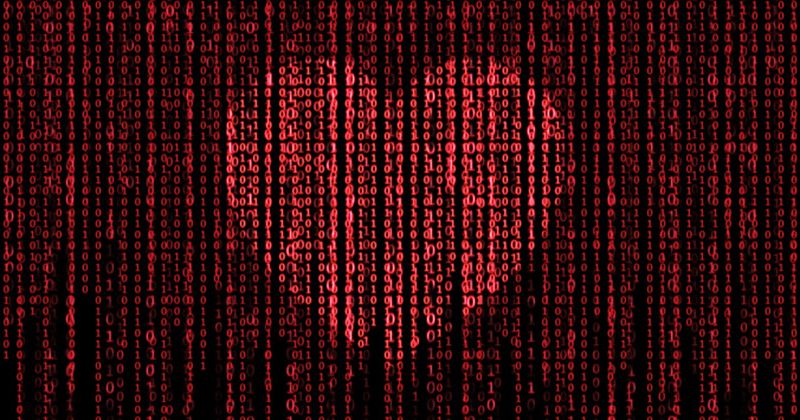
Image: Adobe Stock
“There have been many recent AI algorithms developed for ECGs, but most have only been tested in limited settings — often at the same site the model was trained at, in urban areas with non-diverse patients. We developed this algorithm to identify hidden AF in normal ECGs with a focus on an underserved population. We specifically focused on developing models for Black and veteran populations across California to better optimize and test its performance in underserved populations,” David Ouyang, MD, cardiologist in the department of cardiology at the Smidt Heart Institute and researcher in the division of artificial intelligence in medicine at Cedars-Sinai, told Healio. “The AI algorithm worked well across a range of ages, genders and ethnicities. At multiple VA sites and Cedars-Sinai Medical Center, we were able to show that our AI model works very well and this performance generalizes across many geographically and temporally distinct populations.”
Overall, 907,858 ECGs with normal sinus rhythm (mean age, 62 years; 94% men; 62% white; 11% Black) were acquired from 1987 to 2022 from the 21 regional Veterans Integrated Services Networks within the VA.
ECGs from the San Francisco and Palo Alto VA sites were used for model training, validation and testing, and external testing was conducted using 72,483 12-lead ECGs acquired at Cedars-Sinai Medical Center from 2005 to 2018 (mean age, 60 years; 53% women; 75% white; 9% Black).
Within the VA cohort of ECGs, the AI model predicted AF within 31 days of sinus rhythm with an area under the receiver operating characteristic curve (AUROC) of 0.86 (95% CI, 0.85-0.86), accuracy of 0.78 (95% CI, 0.77-0.78) and an F1 score (which evaluates precision and recall in machine learning) of 0.3 (95% CI, 0.3-0.31), according to the study.
Among the ECGs from Cedars-Sinai, the AI model predicted AF with an AUROC of 0.93 (95% CI, 0.93-0.94), accuracy of 0.87 (95% CI, 0.86-0.88) and an F1 score of 0.46 (95% CI, 0.44-0.48).
The AI demonstrated good calibration, with a Brier score of 0.02 across all sites (0 represents perfect accuracy and 1 perfect inaccuracy), according to the study.
Among those the AI model deemed high risk for AF, the number needed to screen to detect AF was 2.47 for a testing sensitivity of 25% and 11.48 for a testing sensitivity of 75%, and the results were consistent for all groups including Black patients, women, those younger than 65 years and those with CHA2DS2-VASc scores of at least 2.

“We anticipate this type of algorithm to be implemented in clinical practice soon, as many companies are developing similar algorithms. These algorithms should be tested in diverse populations, with particular care to make sure algorithms work in populations that don’t encounter health care as much — they would benefit most from such a screening test,” Ouyang told Healio. “They should be tested beyond just large academic medical centers.”
For more information:
David Ouyang, MD, can be reached at david.ouyang@cshs.org; X (Twitter): @david_ouyang.
Reference:
- Cedars-Sinai uses AI to identify people with abnormal heart rhythms. https://www.cedars-sinai.org/newsroom/cedars-sinai-uses-ai-to-identify-people-with-abnormal-heart-rhythms/. Published Oct. 18, 2023. Accessed Oct. 18, 2023.