AI-identified CAC via routine CT scan may reveal risk for heart attack, stroke
Key takeaways:
- A deep learning algorithm quantified coronary artery calcium scores from routine chest CT scans.
- Patients with a deep learning CAC score of at least 100 were at elevated risk for death and adverse CV outcomes.
Routine CT scans could serve as a useful tool to identify patients at high risk for atherosclerotic CVD when paired with a deep learning algorithm that calculated coronary artery calcium scores, researchers reported.
“Artificial intelligence algorithms can be used to quantify CAC on non-gated chest CTs with similar accuracy as compared with ECG-gated chest CTs,” Allison Peng, MD, an internal medicine resident at Stanford University, told Healio. “Incidental CAC greater than 100, quantified on non-gated chest CT imaging using this AI algorithm, was associated with worse CVD and mortality outcomes, beyond traditional risk factors and variables used in the pooled cohort equations.”
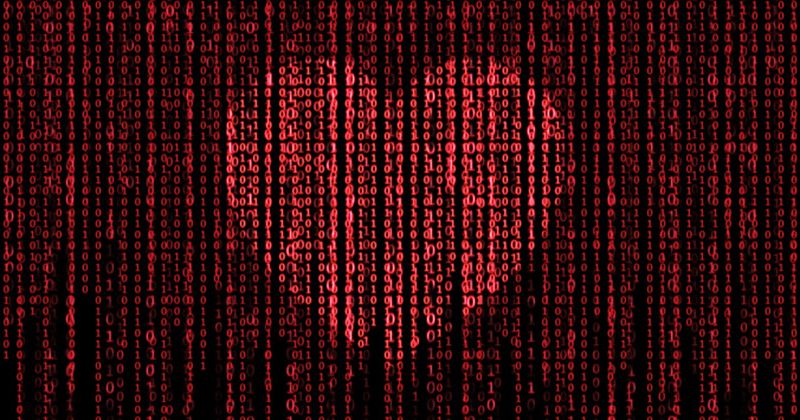
Image: Adobe Stock
Data from routine CT scans
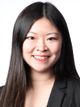
Peng and colleagues analyzed data from 5,678 adults without ASCVD (51% women; 18% Asian; 13% Hispanic) who underwent non-ECG-gated chest CTs for routine care in all settings at a large academic medical center from 2014 to 2019. Researchers quantified incidental CAC using a deep learning algorithm and measured the association between deep learning CAC (scores of 0, 1-99 or 100) with the primary outcome of all-cause death and the secondary composite outcomes of death/MI, MI/stroke and death/MI/stroke/revascularization. Researchers also calculated 10-year ASCVD risk using pooled cohort equations.
The findings were published in the Journal of the American College of Cardiology.
Within the cohort, 52% of patients had a deep learning CAC score of greater than 0.
During an average of 4.8 years of follow-up, the mortality rate was 3.87 per 100 person-years for the overall population. There was a graded increase in mortality rates by increasing CAC grouping: mortality rates were 2.75 per 100 person-years, 3.61 per 100 person-years and 6.06 per 100 person-years for those with deep learning CAC scores of 0, 1 to 99 and at least 100, respectively.
Patients with a CAC score of at least 100 had an average 10-year ASCVD risk of 24%; yet only 26% of these patients were prescribed statins. After adjustment for multiple factors including age, sex, race, comorbidities, systolic BP, lipids and smoking status, patients with a deep learning CAC score of at least 100 were at greater risk for death (HR = 1.51; 95% CI, 1.28-1.79), death/MI/stroke (HR = 1.57; 95% CI, 1.33-1.84), and death/MI/stroke/revascularization (HR = 1.69; 95% CI, 1.45-1.98) compared with patients who had a deep learning CAC score of 0.
“There are over 19 million non-gated chest CTs performed every year for reasons other than CV risk assessment,” Peng told Healio. “Our results highlight the potential for opportunistic screening for CAC on non-gated chest CTs to significantly advance preventive care by identifying patients at high CV and mortality risk and facilitating earlier preventive intervention.”
Peng said it will be important for researchers to determine any sociodemographic differences among patients receiving dedicated CAC imaging vs. non-gated chest CTs, in addition to evaluating whether the AI algorithm for quantifying incidental CAC improves CV risk discrimination beyond the pooled cohort equations, and testing a risk prediction model on diverse populations who are not pooled cohort equation eligible.
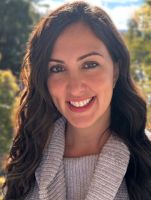
“The exciting implication of this work is that we can use information that is already available and sitting in radiology archives to identify individuals at higher risk of heart attacks and strokes,” Fatima Rodriguez, MD, MPH, FACC, FAHA, FASPC, associate professor in cardiovascular medicine at Stanford University, told Healio. “We can then promptly begin preventive therapies.”
Underutilized ‘free’ information
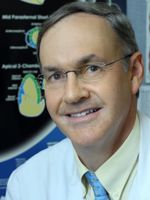
In a related editorial published in JACC, Healio | Cardiology Today Prevention Section Editor Roger S. Blumenthal, MD, director of the Johns Hopkins Ciccarone Center for the Prevention of Cardiovascular Disease and professor of medicine at Johns Hopkins University School of Medicine, and colleagues wrote that by using AI deep learning algorithms, researchers can easily quantify and measure CAC from noncardiac CT chest scans without any additional monetary or radiation-related cost to the patient.
“These results highlight the underutilization of this ‘free’ information and demonstrate a real-world, high-impact use for AI algorithms that is ready right now to directly improve patient care,” Blumenthal and colleagues wrote. “The pragmatic use of this AI algorithm and increased uptake for reporting of incidental CAC has the potential to transform the appropriate allocation of primary prevention medications, motivate asymptomatic adults to make sustained lifestyle improvements, reduce the burden of ASCVD and potentially reverse the recent trend of increased ASCVD in the United States.”
Reference:
For more information:
Allison Peng, MD, can be reached at awpeng@stanford.edu.
Fatima Rodriguez, MD, MPH, FACC, FAHA, FASPC, can be reached at frodrigu@stanford.edu; X (Twitter): @farodriguezmd.