AI models identify five distinct heart failure types, better predicting prognosis
Key takeaways:
- Machine learning models identified distinct heart failure subtypes with predictive accuracy for 1-year mortality.
- Heart failure subtypes may inform management and prognosis.
Researchers have identified five distinct heart failure subtypes using machine learning methods across nationally representative datasets that may predict prognosis and ultimately guide treatment plans for patients.
The study, using three large U.K. datasets, is one of the largest electronic health record analyses to date to use machine learning in subtype classification and risk prediction of HF, and was the first to investigate multiple machine learning methods, multiple datasets and multiple validation methods, Amitava Banerjee, MBBCh, DPhil, MA, MPH, professor of clinical data science and honorary consultant cardiologist with the Institute of Health Informatics at University College London, and colleagues wrote in Lancet Digital Health.
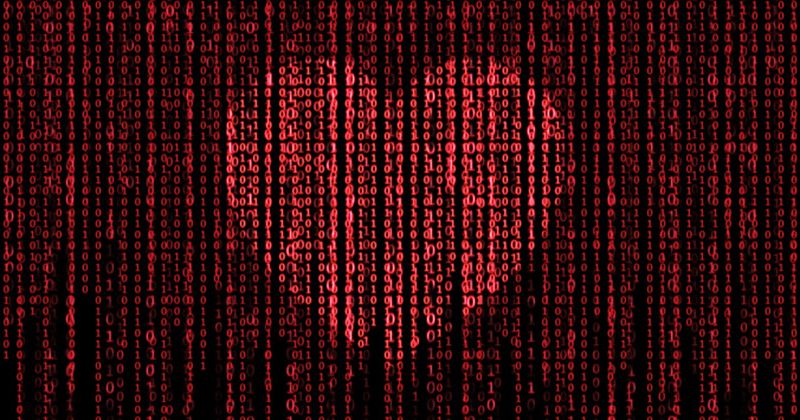
Image: Adobe Stock
“Machine learning can be used in routinely collected data to develop and validate subtypes of diseases, which can be replicated and have clinical value,” Banerjee told Healio. “The five types of HF were based on common risk factors, such as age at onset of HF, history of cardiac disease, history of cardiac risk factors such as diabetes and obesity, or atrial fibrillation, the most common heart rhythm problem. The five subtypes of HF varied widely in their prognosis, such as 5-year mortality.”
Machine learning for prediction

In a prognostic and genetic validation study, researchers analyzed data from adults with incident HF from the Clinical Practice Research Datalink (CPRD; n = 188,800) and The Health Improvement Network (THIN; n = 124,262), as well as the UK Biobank for genetic validation (n = 9,573), from 1998 to 2018. They assessed multiple factors including demographic information, history, exams, blood laboratory values and medications. Researchers identified subtypes using four unsupervised machine learning methods (K-means, hierarchical, K-medoids and mixture model clustering) with 87 of 645 factors in each dataset. The researchers evaluated subtypes for external validity across datasets; prognostic validity, defined as predictive accuracy for 1-year mortality; and genetic validity (using UK Biobank data), association with polygenic risk score (PRS) for HF-related traits (n = 11) and single nucleotide polymorphisms (n = 12).
After identifying five clusters, researchers labeled HF subtypes as early-onset, late-onset, AF-related, metabolic and cardiometabolic.
In the external validity analysis, subtypes were similar across datasets. In the prognostic validity analysis, 1-year all-cause mortality after HF diagnosis differed across subtypes in the CPRD and THIN data, as did risk for nonfatal CVD and all-cause hospitalizations.
In the genetic validity analysis, the AF-related subtype showed associations with the related PRS. Late-onset and cardiometabolic subtypes were the most similar and strongly associated with PRS for hypertension, MI and obesity (P < .0009).
Age and sex varied by subtype, with the oldest participants typically in the late-onset subtype and the youngest participants in the early-onset subtype. Most women were classified as having metabolic HF and few women were classified as having cardiometabolic HF. In THIN dataset, the cardiometabolic subtype had the highest prevalence of CV risk factors and diseases, including hypertension (72.9%), obesity (4.7%), diabetes (34.5%) and atherosclerotic CVD (59.2%).
“Our subtypes showed good accuracy within and across datasets, and good predictive accuracy for early-onset, metabolic and cardiometabolic subtypes; although, less accurate for AF-related and late-onset subtypes,” the researchers wrote. “The exact reason for these differences in predictive accuracy between subtypes cannot be ascertained from this study but might be due to more nuanced changes in risk factors and trajectories over time than we captured in our data or our models.”
Implications for practice
The researchers noted that the HF subtypes might have implications for research in both the EHR and with machine learning to identify HF subtypes in future clinical trials and observational studies, as well as for HF management and prognosis in clinical practice. The researchers developed a prototype app for routine clinical use that could enable evaluation of effectiveness.
“Health professionals should ensure they ask their HF patients about common risk factors, as these factors can help understand the subtype of HF people have,” Banerjee told Healio. “Researchers need to test how usable, generalizable and acceptable these subtypes defined in our study are in clinical practice. They should also consider whether studies such as ours, which use artificial intelligence, can help inform better understanding of disease processes and drug discovery.”
For more information:
Amitava Banerjee, MBBCh, DPhil, MA, MPH, can be reached at ami.banerjee@ucl.ac.uk; Twitter: @amibanerjee1.