Machine learning algorithms improve mortality prediction in hypertension after CABG
Among adults with hypertension who underwent CABG, five machine learning models using patient characteristics each demonstrated acceptable predictive ability for 1-year mortality risk, data from a single-center study show.
“Besides traditional risk scores, machine learning‐developed models are getting attention for outcome prediction after cardiac surgeries,” Kaveh Hosseini, MD, MPH, of the Tehran Heart Center, Cardiovascular Diseases Research Institute at Tehran University of Medical Sciences, and colleagues wrote in Clinical Cardiology. “However, there are controversies about the accuracy of machine learning models compared to risk scores currently being used. Knowing the greater need for mortality prediction in the hypertensive population, we aimed to use and compare different machine learning methods to predict 1‐year mortality of hypertensive patients after isolated CABG.”
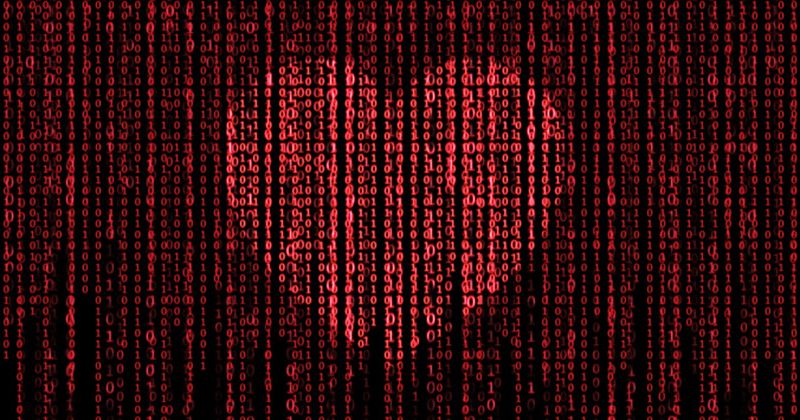
Source: Adobe Stock
Hosseini and colleagues analyzed baseline and periprocedural characteristics and mortality data for 8,493 patients with hypertension who underwent CABG, using data from Tehran Heart Center’s CABG data registry (mean age, 68 years; 63.86% men). Data were collected between 2005 and 2015.
The researchers chose the best characteristics using a random-forest feature selection algorithm and developed five machine learning models to predict 1‐year mortality: logistic regression, random forest, artificial neural network, extreme gradient boosting and naive Bayes, and assessed area under the curve, sensitivity and specificity for each. The primary outcome was 1-year mortality after CABG, including in-hospital and after discharge, compared between the different prediction models.
Within the cohort, 303 patients died in the first year after CABG.
Total ventilation hours and ejection fraction were top among the 11 leading features that best predicted 1-year mortality across models, according to the researchers.
The logistic regression model showed the best prediction ability, with an AUC of 0.82, whereas the lowest AUC was for the naive Bayes model (0.79). Among the subgroups of people with hypertension, the highest AUC for the logistic regression model was for two age-range groups (age 50-59 years and age 80-89 years), people with overweight, people with diabetes and smokers.
“All machine learning models, including logistic regression, random forest, artificial neural network, extreme gradient boosting and naive Bayes, demonstrated acceptable predictive performance, with logistic regression providing the greatest AUC,” the researchers wrote. “Machine learning algorithms may pave the innovative way for early and accurate prediction of post‐CABG mortality in high‐risk groups, especially hypertensive patients. It could offer individualized tools for clinical decision‐making and management. However, the current study’s findings warrant further validation by more studies.”