Public websites show low accuracy in predicting pollen counts in five U.S. cities
Click Here to Manage Email Alerts
Key takeaways:
- Pollen forecasts varied significantly among websites used in the study.
- The forecasts showed high variability and low overall accuracy.
Pollen predictions on public websites across five U.S. cities showed significant inaccuracies, according to a study published in The Journal of Allergy and Clinical Immunology: In Practice.
None of the websites that were analyzed consistently provided accurate pollen counts when compared with data from the National Allergy Bureau (NAB), Divya Shah, MD, resident in the department of internal medicine, The University of Arizona College of Medicine, and colleagues wrote.
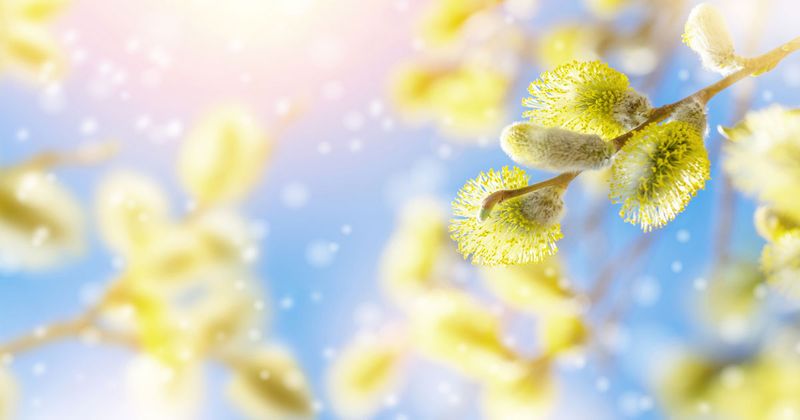
The study looked at tree, grass and/or ragweed pollen levels from NAB stations in Washington, D.C.; Atlanta; Scottsdale, Arizona; Seattle; and Omaha, Nebraska, from March 1 to May 1, 2023. It then compared these levels with data from four prominent websites, identified in the study as A, B, C and D, chosen through a Google search for “pollen forecasts.”
Data from the websites were recorded based on numerical counts to establish a classification system of “none, low, medium, high” to align with NAB guidelines. Data analysis involved comparing tree, grass and ragweed pollen levels from NAB with 1-day pollen forecasts from the websites alongside a persistence model forecast method that used an NAB count from the day before to predict the next day. The days were then classified as either a match, a near match or a mismatch.
Data analysis of tree pollen forecasting accuracy in the five cities revealed that the persistence model continuously outperformed the chosen websites. Among the websites, C and D were more accurate in reporting tree pollen levels than the others. C was most accurate in Washington, D.C., Seattle and Scottsdale, whereas D worked best in Atlanta and Omaha.
The persistence model was the most accurate in tree pollen forecasting for exact matches. The model’s predictions were 73%, 65%, 66%, 54% and 53% for Washington, D.C., Atlanta, Scottsdale, Seattle and Omaha, respectively, compared with prediction rates for the websites of 54%, 44%, 34%, 25% and 34%.
In assessing grass pollen forecasting, website A did not report on grass pollen, and B, C and D did not include grass pollen in their reports on Seattle and Omaha.
The persistence model showed the highest accuracy in grass pollen forecasting for Washington, D.C., Atlanta and Scottsdale, including match counts of 18 of 19, 27 of 34 and 26 of 43. Website D had the highest grass pollen count match percentages for these cities at 80%, 71% and 23%.
For ragweed, website A did not provide reports, whereas B, C and D did not have consistent reports. Websites C and D were most accurate in reporting ragweed pollen forecasts in Washington, D.C., with two of two. Websites B and C had more matches in Atlanta than the persistence model with 25 of 33 vs. 11 of 17. The persistence model was most accurate in Scottsdale with 50 of 61 matches. In Omaha, the persistence model along with websites B, C and D had accurate reports of ragweed pollen counts of none (12 of 12; 21 of 21; 20 of 20; and 20 of 20).
Authors of the study emphasized the significant variability and low accuracy in these publicly accessible websites. They speculated that the factors impacting this inaccuracy could be unpredictable weather conditions and a lack of existing data to serve as a basis for forecasting. Also, they encouraged a reevaluation of current forecasting methods.