Artificial intelligence counts, classifies pollen grains
Key takeaways:
- The model was trained with 6,404 images of 29 pollen species.
- There was 96% correlation in identifying pollen counted by a human and by the model.
- A commercial system is now in development.
ANAHEIM, Calif. — An artificial intelligence model counted and classified grains of pollen almost as accurately as a human being, according to a poster at the American College of Allergy, Asthma & Immunology Annual Scientific Meeting.
This technology could lead to commercial devices that provide individual pollen counts to inform personalized medicine, Leonard Bielory, MD, FAAAAI, of Hackensack Meridian School of Medicine, told Healio.
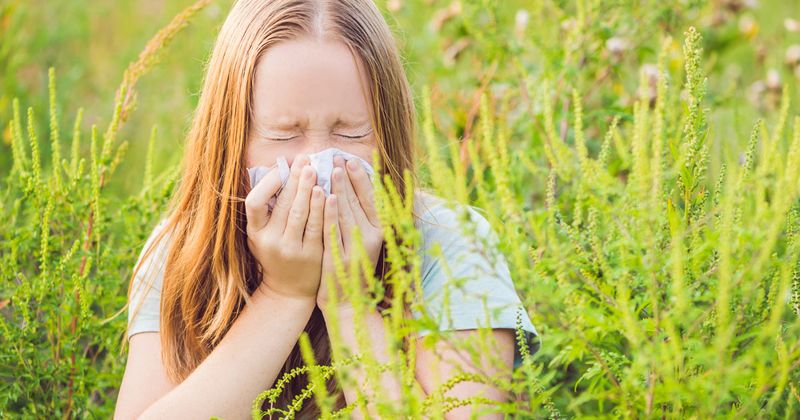
“There is a need in the United States — well, worldwide,” Bielory said.

Sensitivity to pollen varies, he continued, with patients triggered by counts anywhere between 200 or 2,000. But with this system, Bielory said, patients may be able to anticipate when pollen counts will get worse and take medicine to prevent attacks.
Pollen counting currently depends on manual approaches, Bielory continued, that can cost between $15,000 and $20,000 in personnel time.
“It’s like hieroglyphics. It’s a very ancient way,” he said, comparing the pollen counting process with the process for counting red blood cells. “Nothing is automated. The future is automated.”
AI at work
Bielory and his colleagues developed a two-stage pollen detector and classifier that they trained and tested with pure samples and with data collected in the wild, taken at a mixture of 100 times and 400 times magnification and stained with Calberla’s solution.
The researchers then used these data to train an object detection model based on Detection Transformer, or DETR, with all classifications collapsed into a single category.
Separating the model into two stages, with one that only detects pollen and another that only classifies it, enabled the researchers to simplify each task and separately measure their accuracy, the researchers said.
Using the ResNet-50 DetrForObjectDetection (Hugging Face) model, the researchers trained the system to detect and count pollen with 337 images of slides that had multiple pollen grains.
The researchers also measured detection accuracy primarily via correlation between the human and machine counts and the machine count within an image. Additional visual confirmation on sampled images was used as well.
The classifier was a conventional neural network model in PyTorch with seven layers. Training included 6,404 images of individual pollen grains from 29 different species that were rotated by 90, 180 and 270 degrees and reflected. The researchers analyzed classification via training loss, including an additional check of a confusion matrix.
There was 96% correlation during the identification stage between pollen grains counted by a human being and those counted by the DETR model for each image during validation.
Also, the researchers found, classification generated 88% accuracy during validation, with greater than 99% correlation noted with up to 60 pollen grains and slight deviations from 80 to 100 pollen grains. Classification also yielded a training accuracy of 96.8% and a validation accuracy of 94.7%.
Looking ahead, the researchers said count accuracy could be improved by cropping images that have many grains into more images with fewer grains so each grain would take up a larger portion of the total image.
The researchers also cautioned that model results from the “in the wild” samples had less accuracy when they used images that were less like the training data due to additional detritus and microscopy techniques.
Next, the researchers said they expect classification results for images will improve with additional included data and a wider variety of imaging techniques.
“Whether it’s a tree, grass or a weed,” Bielory said. “It can be done.”
Potential commercialization
Bielory and his team are now working with three engineering schools to develop and commercialize automated and semiautomated devices that patients with pollen allergies can use to get their own pollen counts.
Unlike the current devices that require manual counts, a new device could use an iPhone like a microscope, placing the glass slide under it to do the count, according to Bielory.
The smart phone or other device would be loaded with the detector and classifier model that would provide the results, Bielory said, adding that he and his colleagues are developing an adapter that would work with smart phones to collect and display pollen for them to analyze.
“It’s do-it-yourself pollen counting. Say, ‘What’s in the air? What’s bothering me now?’” he suggested.
Already, Bielory said, he and his team have developed an app for this system, which is now available.
“But we’re working on the next phase, where you can score yourself and your symptoms,” he said. “It will then predict or forecast for you when you’re going to have problems, 72 hours before the [pollen] cloud or before it rains. We will be able to tell you what’s in the air.”
The system will account for geographic differences in when pollen seasons occur as well as in the types of pollen that flourish too, Bielory continued.
“People will then know, ‘Gee, this is my season. This is when I can start taking medications to be preventative,’” he said.
The researchers will continue to improve the system’s counting and classification abilities too, Bielory said, as pollen seasons overlap.
“Trees don’t pair with weeds. But they can overlap with grass,” he said. “It’s usually trees and grasses, and grasses and weeds.”
Training the system to differentiate between pollens begins with a swipe of one pollen. Then, a second pollen is added to the slide.
“Let it count total pollen and see if it differentiates between the two pollens. And then add three pollens, and let it do it again. And do it again,” he said.
Bielory and his colleagues expect to release the first version of the system within a year, although overall training will take several years.
“The more you do, the better the training,” he said.