Utilizing machine learning plus clinical features accurately predicted PCOS
Click Here to Manage Email Alerts
Key takeaways:
- Challenges persist in PCOS diagnosis and misdiagnosis.
- A workflow that incorporated machine learning accurately predicted PCOS, in combination with ovarian volume, anti-Müllerian hormone and other clinical variables.
DENVER — A prediction model that integrates machine learning-derived measures of ovarian volume, anti-Müllerian hormone levels and other clinically-derived diagnostic endpoints accurately predicted polycystic ovary syndrome.
“Diagnosing PCOS is often limited by high inter-observer variability, which can limit accuracy of diagnoses,” Adam T. Evans, MD, a reproductive endocrinology and infertility fellow at the University of Rochester Medical Center, said during a presentation at the ASRM Scientific Congress & Expo. “Integration of AI and machine learning models in pelvic ultrasound may improve accuracy in assessment for polycystic ovarian morphology.”
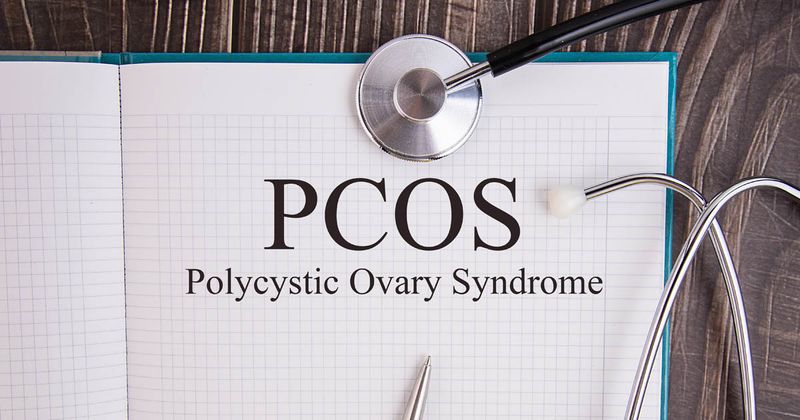
Evans and colleagues evaluated a prediction model for PCOS that incorporated machine learning-derived measures of ovarian morphology plus anti-Müllerian hormone levels and other clinical features. The study utilized transvaginal ultrasound images and other clinical data from 170 women with PCOS (mean age, 26 years) and 79 without diagnostic features of PCOS (mean age, 31 years). Researchers trained and validated a machine learning model (Ovarify) to identify and segment the ovary on pelvic ultrasound. Researchers then trained a logistic regression model to predict PCOS status using ovarian volume derived from Ovarify, anti-Müllerian hormone levels and other clinical variables including age, BMI, menstrual cycle length and testosterone.
Utilizing anti-Müllerian hormone levels only predicted PCOS status with moderate accuracy (area under the receiver operating characteristic curve [AUROC]= 0.55), according to the results. When the researchers integrated anti-Müllerian hormone levels and ovarian volume, model performance improved, with acceptable accuracy (AUROC = 0.73), Evans said. Researchers observed high performance and excellent diagnostic accuracy for PCOS prediction after integrating ovarian volume, anti-Müllerian hormone levels and all clinical features into the model (AUROC = 0.94), according to Evans.
The machine learning model had an acceptable performance for PCOS (AUROC = 0.94) when refined to a limited set of variables, including anti-Müllerian hormone levels, ovarian volume, hirsutism score and max self-reported menstrual cycle length, according to the results. When ground truth values for ovarian volume, determined by expert evaluation, replaced values derived by Ovarify, the model with a limited set of variables had similar prediction (AUROC = 0.9).
The researchers noted that this was a small pilot study with a small sample size. Currently, Ovarify is only validated to use ovarian volume. It is being validated to also measure follicle number per ovary, according to the researchers.
“The use of a standardized ovarian volume calculation from a deep-learning model, in combination with anti-Müllerian hormone and clinically derived diagnostic endpoints, has the potential to provide accurate diagnosis of PCOS while minimizing additional lab testing,” Evans said.
The researchers said these results demonstrate that machine learning has the potential to streamline and improve the diagnostic workflow for PCOS.