Generative AI: What it is, how it can be utilized in OB/GYN
Key takeaways:
- Generative AI is a deep learning subset that creates content based on various data and prompts.
- Though generative AI may benefit OB/GYNs, improvements are needed regarding accuracy, incompleteness and biases.
SAN FRANCISCO — AI has evolved rapidly in the past few years, and with this comes generative AI, which creates content based on short prompts and plentiful data that might be useful in health care while also posing some trade-offs.
“Unless you’ve lived under a rock in the last year and a half, we all know that generative AI has been in the news a lot,” Nichole Young-Lin, MD, MBA, FACOG, Women’s Health Clinical Lead at Google and adjunct clinical assistant professor at Stanford University School of Medicine, said during a presentation at the ACOG Annual Clinical & Scientific Meeting. “This is a very exciting wave of new technology with potential benefits, but also trade-offs.”
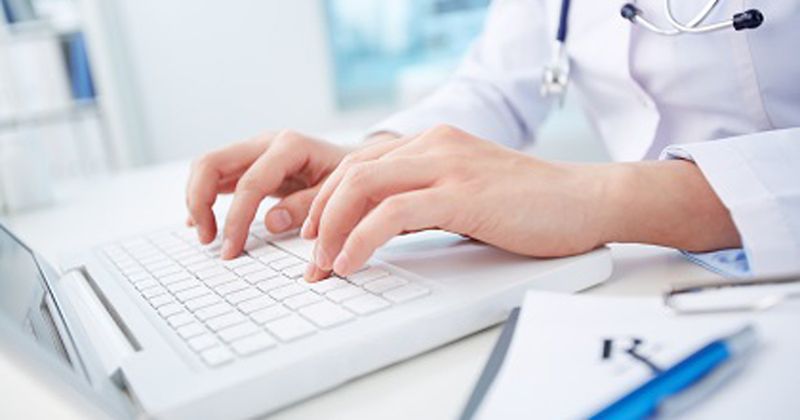
Traditional and generative AI models are widely used among consumers and many patients present to providers with AI medical diagnoses and information. Because of this, it is important for providers to understand and learn about AI tools as, despite many benefits of AI in health care, there are still some caveats to be conscious of.
What is generative AI?
AI is the concept of machines performing tasks that normally require human intelligence. Under AI, machine learning algorithms learn from data patterns and relationships without being explicitly programmed, according to Young-Lin.
There are three main types of machine learning: supervised learning, unsupervised learning and reinforcement learning. According to Young-Lin, supervised learning is when the algorithm learns from labeled training data usually from humans, unsupervised learning is when the algorithm learns from unlabeled training data and discovers relationships based on data alone, and reinforcement learning is when the algorithm learns to make decisions by interacting with the environment and with rewards.
In addition, a subset of machine learning uses artificial neural networks called deep learning, Young-Lin said, which can be used in the obstetrics and gynecology space for mammography reading, for example. Today, companies and different academic centers utilize AI to aid radiologists in diagnosing cancerous or abnormal lesions before they become cancer or before possible misdiagnosis. Generative AI is a subset of deep learning that can generate audio, video and other data.
“When we think about generative AI, we are thinking about large amounts of data that are being ingested and used to train what we call a foundation model. These are the foundation models that you’ve heard of in the news, like GPT, which stands for generative, pretrained transformer,” Young-Lin said. “In that training process, especially at big companies with a lot of engineers with expertise, we are able to do what we call reinforcement through human feedback. So, people evaluate some of the responses and retrain that model at the foundational level.”
According to Young-Lin, once these foundation models are ready, they can be fine-tuned and adapted for the required task.
“This is powerful because the models no longer need to be trained explicitly for just one task,” Young-Lin said. “You can use the same model to do a variety of tasks, and that is the power of generative AI.”
Currently, according to Young-Lin, generative AI models can provide more than 90% correct responses in the U.S. Medical Licensing Examination compared with only 50% correct responses recorded a year and a half ago.
“In the Silicon Valley world and in the tech world, there’s often a saying of ‘move fast and break things,’ but in medicine, sure we’d want to move fast, but we definitely don’t want to break anything,” Young-Lin said. “There’s still a lot that we’re learning right now as our field is adopting more technology.”
Generative AI in health care
Today, companies are working on applying generative AI to biomedical and natural language processing for protein and drug design for digital twins and biosimulation and synthetic patient data, according to Young-Lin. Generative AI is also utilized in the clinical world with radiologic image enhancement, electronic health records and coding.
Generative AI has many positive health care aspects. For example, it might simplify administrative tasks, such as scheduling, process referrals, and coding and billing. In addition, work is underway to incorporate generative AI in clinical documentation, inbox management, clinical diagnosis, treatment, decision support, error detection and abnormality detection for providers. For patients, generative AI might improve patient education, care summaries, language translation, personalized care and experiences, and access to affordable health care.
In medical education, generative AI can aid both learners and educators. For learners, generative AI can increase learning efficiency, individualize learning and feedback and engage with dynamic content while creating lesson plans and materials, providing real-time feedback and improving student assessments for educators. Generative AI can also help with research, Young-Lin said, for drug development, predictive models, connecting patients to clinical trials, conducting literature reviews and drafting abstracts and papers.
Finally, in public health, generative AI can be useful for data analysis for population health management, upskilling health care workers, developing public health campaigns and optimizing resource allocation.
“It’s not all rainbows and butterflies, for sure,” Young-Lin said. “The fact that we live in this world of AI, and not just generative AI, there’s a lot of things that we need to think about, especially as providers.”
Some important aspects to consider are privacy and security, ethical and legal issues and overreliance. In health care, sensitive data requires security and privacy, and currently, there is a lack of transparency or users and patients about what their data is being used for. Young-Lin also noted generative AI ethical and legal concerns, especially for intellectual property, copyrights, regulations, liability and ownership. With overreliance, there are concerns that generative AI might result in deskilling, dehumanization and bypassing the learning processes.
In addition, generative AI models have some negative aspects. They can invent information to provide a response, provide incomplete responses and omit key information, according to Young-Lin, which can be dangerous when dealing with patients and their care.
“We also know that bias and discrimination are pervasive in medicine,” Young-Lin said. “These models are being trained and encoded with existing data that are inherently biased, and then these models are perpetuating and amplifying these biases in their responses.”
In one study published in January, researchers evaluated use of the GPT-4 model in the same 17 clinical scenarios and observed changes in recommendations and referral patterns when the race changed.
“We have a unique opportunity and responsibility to be at the forefront of this change. To really have a voice, you need to understand it and the first step is to try it,” Young-Lin said. “Only when we understand can we actually guide the development and ensure that these tools and technology serve the best interests of our patients and women’s health.”