Artificial neural network can diagnose, stage COPD with single CT scan
Click Here to Manage Email Alerts
Key takeaways:
- The training process of the neural network involved clinical data and a CT scan (either single-phase or multiphase).
- Prediction accuracy became better with the addition of clinical data to models.
The accuracy of an artificial neural network in identifying COPD and Global Initiative for Chronic Obstructive Lung Disease, or GOLD, stage was similar with use of single-phase CT or inspiratory-expiratory CT, according to study results.
These findings were published in Radiology: Cardiothoracic Imaging.
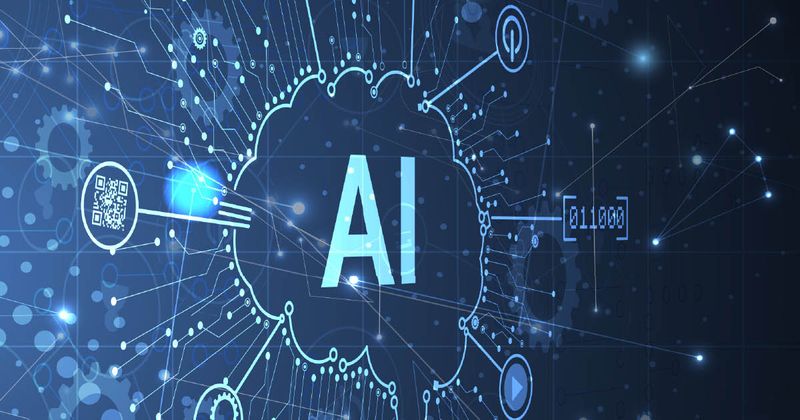
“Although many imaging protocols for COPD diagnosis and staging require two CT acquisitions, our study shows that COPD diagnosis and staging is feasible with a single CT acquisition and relevant clinical data,” Kyle A. Hasenstab, PhD, assistant professor of statistics and data science at San Diego State University, said in a Radiological Society of North America press release.
Using the COPDGene phase 1 cohort, Hasenstab and colleagues analyzed 8,893 individuals (mean age, 59.6 years; 53.3% men; 43.3% GOLD 0) with inspiratory and expiratory lung CT images, as well as spirometry measures to determine how COPD diagnosis and staging via a convolutional neural network (CNN) model differs when using single-phase CT vs. inspiratory-expiratory CT, both with and without clinical data.
The training process of the CNNs involved clinical data and a CT scan (either single-phase or multiphase), with the goal of predicting FEV1, FEV1 percent predicted and FEV1/FVC for the ultimate use of predicting GOLD stage, according to the study.
Researchers reported “moderate to good agreement” between CNN-predicted and reference standard spirometry measurements based on the intraclass correlation coefficient (ICC) in both single-phase CNNs (ICC, 0.66-0.79) and inspiratory-expiratory CNNs (ICC, 0.72-0.8). After adding clinical data, this level of agreement significantly went up (single phase ICC, 0.7-0.8; inspiratory-expiratory ICC, 0.76-0.85).
When diagnosing COPD and predicting GOLD stage (within-one stage and single stage), the accuracy range was 59.8% to 84.1% using the single-phase CNN model, according to researchers. “Moderate to good agreement” was also detected during this assessment (ICC, 0.68-0.7).
Notably, the study highlighted that the accuracy range and level of agreement both became better after clinical data were added to this model (65.2% to 85.8%; ICC, 0.72).
Researchers observed a similar accuracy range to the one found for the single-phase CNN model when evaluating inspiratory-expiratory CNN models (60% to 86.3%), as well as a similar level of agreement (ICC, 0.72).
The similarity pattern continued after clinical data were added in the inspiratory-expiratory CNNs, as accuracy improved (67.6% to 88%) and agreement went up (ICC, 0.77-0.78), according to the study.
Breaking down this model into inspiratory and expiratory, researchers found that the expiratory CNN demonstrated no improvements in these two factors following the clinical data addition.
“Reduction to a single inspiratory CT acquisition can increase accessibility to this diagnostic approach while reducing patient cost, discomfort and exposure to ionizing radiation,” Hasenstab said in the release.
Reference:
- Deep learning model accurately diagnoses COPD. https://www.rsna.org/news/2024/december/deep-learning-for-copd-diagnosis. Published Dec. 12, 2024. Accessed Dec. 12, 2024.