AI models detect pulmonary embolism early with medical record data
Click Here to Manage Email Alerts
Key takeaways:
- Models included age, sex, BMI, anticoagulant use and prior pulmonary embolism (PE), chronic lung disease and thrombosis.
- Previous diagnoses that could result in PE include PE, pneumonia and deep vein thrombosis.
Two machine learning-based models using only medical record data had high accuracy in detecting individuals with and without pulmonary embolism at hospital admission, according to results published in Journal of Medical Internet Research.
“The plan is for findings from this study and similar studies to eventually be assimilated into a large model that will support the prediction of patient deterioration and assess the risk of disease and illness,” Gad Segal, MD, head of the Education Authority at Sheba Medical Center, told Healio. “This model is currently in the process of development in hospitals and academic centers worldwide. Once it’s built, we expect to see a bigger impact on the everyday clinician.”
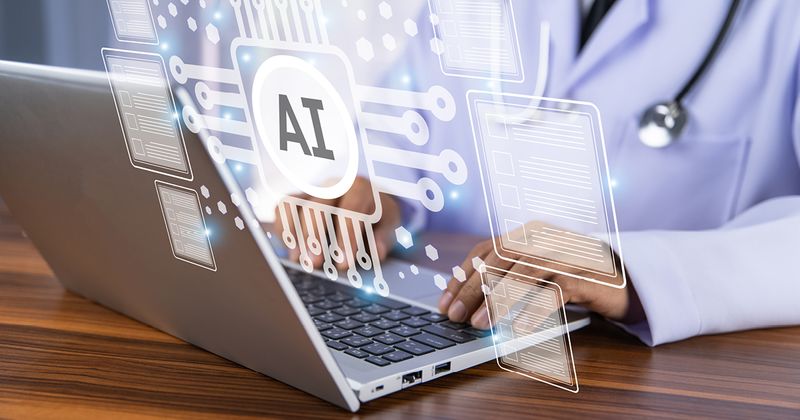
In this study, Segal, Boaz Lerner, PhD, associate professor in the department of industrial engineering and management at Ben Gurion University, and colleagues developed, trained and tested two machine learning-based methods using medical record data — demographics, comorbidities and medications — from 2,568 patients with pulmonary embolism (PE) and 52,598 control patients to see if the models could correctly detect PE at hospital admission.
“The first [method] empirically sets a classifier decision threshold to account for the minority-to-majority ratio in the imbalanced data, and the second [method] uses an ensemble of balanced classifiers evaluating the PE class vs. equal-sized disjoint portions of the imbalanced non-PE class,” Segal and colleagues from Ben Gurion University wrote.
More specifically, medical history data factored into the methods included age, sex, BMI, previous clinical PE events, chronic lung disease, previous thrombotic events and anticoagulant use.
Using only this information, researchers found high accuracy in detecting those with PE and those without PE with both method one (0.86) and method two (0.78), as well as high geometric means (method one, 0.8; method two, 0.77), true-negative rates (method one, 0.86; method two, 0.88) and true-positive rates (method one, 0.75; method two, 0.68).
Notably, precision was better with method two (0.85 vs. 0.19), but method one had a higher negative predictive value (0.99 vs. 0.73).
“The very low precision value and very high negative predictive values of method one suggest that the decision threshold that was selected by this method is low, allowing more subjects to pass the threshold, among them (because of the imbalance) more controls that are thus incorrectly predicted as PE, reducing precision,” Segal and colleagues wrote.
Of the seven features included in the methods, researchers highlighted that three are important for PE identification: previous PE, chronic lung disease and previous thrombotic events.
“We did not know before initiating the study, if indeed, pulmonary embolism could be anticipated by background patient data upon arrival to the [emergency room] and we were surprised to learn the positive answer,” Segal told Healio.
A secondary analysis within this study clustered individuals based on age, sex, BMI, 14 past diagnoses and anticoagulant use in different combinations in order to identify a cluster mainly made up of patients with PE and evaluate the features.
This analysis included a total of 46,639 individuals, of which 1,942 (4.16%) had a PE diagnosis at hospital admission.
One cluster including 1,120 individuals and the features of sex, age, BMI, previous PE, previous pneumonia, previous deep vein thrombosis (DVT), history of atrial fibrillation/atrial flutter, history of mental disorders and history of coagulopathies had 705 individuals (63%) with PE, or 36% of all patients with PE.
Researchers noted that the above cluster was part of a five-cluster scheme that had the same features. Between this cluster and the four other clusters, a previous PE diagnosis was found in more patients from this cluster.
Further, the incidence of two other characteristics was elevated in this cluster vs. the four other clusters: previous DVT (12% vs. 2%) and previous pneumonia (11% vs. 3%-4%).
“In addition to past PE, which is arguably the most important variable for marking patients with suspected PE, past diagnoses of pneumonia and DVT may also lead to PE,” Segal and colleagues wrote. “Thus, we recommend overattention to admitted patients with these past diagnoses.”
In a different five-cluster scheme that included the features of sex, age, BMI, previous PE, previous pneumonia, history of mental disorders and anticoagulant use, researchers found a high prevalence of patients with PE in two clusters. One of the clusters included a total of 701 individuals (all women), of which 427 (61) had PE, and the other cluster included a total of 549 individuals (all men), of which 340 (62%) had PE.
Similar to the other cluster scheme, a previous PE diagnosis was observed in more patients from these two clusters vs. the three other clusters.
The incidence of previous pneumonia and anticoagulant use was also higher in the two clusters outlined above vs. the remaining three clusters.
“We are seeing a shift in medicine which hints to the future of care,” Segal told Healio. “Instead of a personal experience, we are moving towards big data accumulation. Instead of clinical intuition, we are embracing AI.
“I expect future studies to continue focusing on how we can integrate big data and AI into clinical prediction models for different diseases,” Segal added.
Reference:
- Sheba Medical Center published new peer-reviewed study demonstrating success of AI model to predict risk of pulmonary embolism. https://sheba-global.com/sheba-medical-center-publishes-new-peer-reviewed-study-demonstrating-success-of-ai-model-to-predict-risk-of-pulmonary-embolism/. Published Aug. 12, 2024. Accessed Aug. 12, 2024.