Q&A: Research to assess artificial intelligence in predicting adverse outcomes of OSA
Click Here to Manage Email Alerts
Key takeaways:
- Researchers at Mount Sinai are looking into the use of artificial intelligence for predicting obstructive sleep apnea outcomes.
- This study consists of retrospective and prospective model validation.
Over the past year, interest in artificial intelligence, or AI, in the pulmonology field has been growing.
In just January 2024, Healio reported on a study showing that assistance from AI enhanced nonradiologists’ ability to detect lung lesions on chest radiographs, as well as the FDA clearance of LungQ 3.0.0 (Thirona), an AI-based software that identifies different parts of lung anatomy and evaluates lung tissue and fissure completeness.
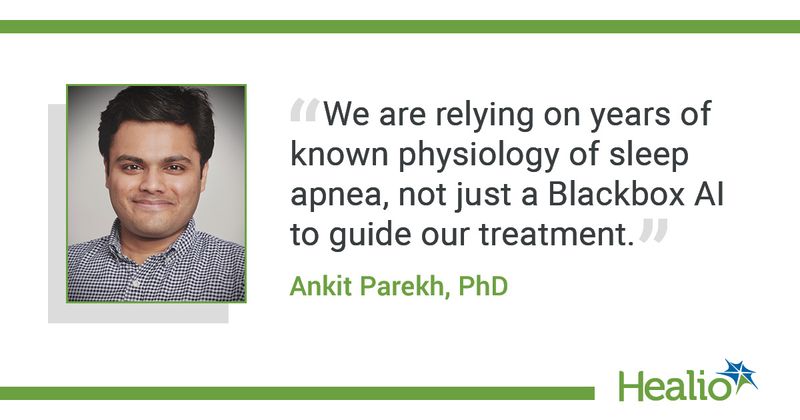
Now, researchers at Mount Sinai are investigating whether an AI model can predict short- and long-term adverse outcomes in patients with obstructive sleep apnea, according to a press release. During this study, researchers will retrospectively and prospectively validate their AI model, with hopes of creating a new tool for managing sleep apnea.
To learn more about this research, the tools currently used for diagnosing OSA and the expected benefits of the AI-powered approach, Healio spoke with Ankit Parekh, PhD, director of the Sleep and Circadian Analysis (SCAN) group and assistant professor of medicine at the Icahn School of Medicine at Mount Sinai.
Healio: What are some common adverse outcomes of OSA? How do these outcomes worsen with a delayed diagnosis of the condition?
Parekh: There are two kinds of categories of adverse outcomes: short-term and long-term consequences. Short-term consequences include daytime sleepiness, which is one of the most common complaints for people with sleep apnea. For long-term consequences, studies have shown associations between sleep apnea and Alzheimer’s disease, diabetes, cerebrovascular disorders, cardiovascular, stroke and even all-cause mortality.
When left untreated, sleep apnea can cause harmful, immediate and long-term consequences. Studies have so far shown that if left untreated, the risk for cardiovascular events is almost three to four times as compared with those without sleep apnea. There’s still ongoing research on identifying whether everyone can benefit from CPAP, the primary treatment for sleep apnea, or if it is only a certain subgroup that shows improvement.
Additionally, more recent studies are suggesting that delayed or lack of treatment for sleep apnea can actually cause irreversible brain damage and may be the reason why people even after treatment with CPAP are still feeling those consequences.
Healio: How do clinicians currently diagnosis OSA in patients? What are some disadvantages of these tools?
Parekh: Currently, it’s a multifactorial process. Several metrics and patient history are taken into account when diagnosing OSA. The main component in all of these diagnoses is the gold standard, nocturnal polysomnogram, which looks at breathing and activity in the heart, brain and so on. The primary criterion for clinical diagnosis is the apnea-hypopnea index (AHI), which is the number of times that your breathing stops during the night or is reduced with some immediate consequences, such as an oxygen desaturation or an EEG arousal. All of these factors are then combined into an index and are used as the primary metric for diagnosis of OSA. Clinicians rely on several other factors as well, but AHI is the major component that gets used in the diagnosis of sleep apnea.
In terms of drawbacks, one is that the nocturnal polysomnogram in lab is very burdensome for the patient, so clinics have moved to home sleep testing. Home sleep testing can calculate AHI, but it’s not accurate because AHI requires the total sleep time, which most of the home devices cannot give you.
The other major component is that AHI by itself is a very simple metric. It works well in cases that are on the extreme end. For those who have very severe sleep apnea and those who completely do not have sleep apnea, AHI is a good metric to use. However, in between that spectrum, we see a lot of variability where you could have two people with similar values of AHI, yet they show different consequences. One of them could be sleepy throughout the day, whereas the other could not.
Currently, there are very few, if any, clinical decision aid tools that can tell you which patients should be prioritized for treatment and whether they’re likely to show any benefit or not.
Healio: How will the developed AI-powered model by researchers at Mount Sinai address these disadvantages?
Parekh: What we have seen so far in literature is all studies that have associated sleep apnea with adverse consequences have used AHI as a metric. There is an association on an epidemiological level, but when it comes to individual patient level, things get murky.
The way we have approached this problem is to rethink the way AHI is calculated. AHI depends on oxygen desaturation, EEG arousals and other factors, so we said, ‘Let’s take those apart and develop a better metric for each of those components.’ Then, we let AI combine them, rather than the way we have been combining them by adding them up and dividing them by total sleep time.
Our hope is that the AI-powered approach can take into account these individual differences and variability that traditional statistics are limited to. We are relying on years of known physiology of sleep apnea, not just a Blackbox AI to guide our treatment. AI models that act as a blackbox, ie, Blackbox AI, while powerful, do not lend themselves to an easy interpretation of the results. As such, measures which may not be important clinically could be the factors driving the Blackbox AI’s decisions.
Healio: Could you provide some details about what will happen during the study?
Parekh: The study has two parts, with one of them being a retrospective validation. The way we are approaching this is we are looking at patients from Mount Sinai who had a sleep study done between 2016 and 2024, and we are validating our model using their data to see whether we can predict those outcomes. These patients have already had those outcomes, so this is a retrospective analysis, and the model is being developed and validated through that.
The true test of these AI models is a prospective validation, such as a patient seen at a sleep clinic where the clinician is assessing them for sleep apnea. We want to know, can we in real-time give them a probability/likelihood metric that says this person’s sleep study shows they are at a high risk of cardiovascular or neurodegenerative disorders, and can that guide treatment? If we are right, that treatment would benefit them in terms of the outcomes.
During this part of the study, researchers will be blinded to the outcome for the patient. They will make a prediction prior to the patient’s treatment on whether the patient is likely to benefit from treatment with CPAP or any other modalities. Any treatment out there for sleep apnea will be included as part of the study.
Healio: What do you hope to achieve with your research?
Parekh: With the recent focus on AI and machine learning, there is a need for clinical validation of these tools and how they can be better used to get by in sleep clinics and labs throughout the world. In order to do that, we need to validate it retrospectively and prospectively. What most studies miss out on is prospective validation, short of actually doing a clinical trial. With these AI-powered tools, things get a little challenging when you are talking about clinical trials. It’s still a new area for many in the field.
What our study is setting out to do is the next possible alternative to a clinical trial. We would be blinded to the treatment outcome but make the prediction of whether the patient is likely to benefit or not using our AI tool.
We hope to show that our AI-enabled metric, in addition to clinician’s diagnosis, allows them to better predict who is likely to improve on treatment vs. when they do the same assessment using AHI.
Healio: If successful, what are the expected benefits of the AI-powered approach for patients?
Parekh: For patients, knowing whether they are likely to be at a high risk for a certain adverse outcome may motivate them to use their treatment in a more compliant way. Currently, the major issue is that people don’t use their CPAP treatment as much as we would like. If this approach is made available to patients, it may motivate them to use and reassess their risk every so often. Seeing that their sleep apnea is improving and their risk is being lowered may motivate patients.
In addition to that, because of new treatment alternatives and options coming up for sleep apnea, addressing which treatment should be prioritized for which patients is going to be the next challenge. We are hoping that with our AI-based phenotyping and endotyping, we will be able to predict which treatment is most likely to benefit certain patients.
Healio: Also, if the study is successful, what would other sleep clinics need before they can begin using this technology?
Parekh: Our philosophy at Mount Sinai and within our group has been fostering open science. We are making the tool available publicly as part of a sleep program that clinics over the world can use without needing to know AI or having technical expertise.
We want to distribute this tool validated at our clinic and get buy-in from other clinics to validate or improve it, with the hope that it could be eventually embedded in their clinical sleep management pipeline.
If feasible and possible, we also hope to make our technology available as an app to patients. If our model allows interpreting that from a wearable device, we hope that patients themselves can see that every night and assess their risk.
Reference:
- Mount Sinai researchers awarded $4.1 million NIH grant to advance understanding of sleep apnea using artificial intelligence. https://www.mountsinai.org/about/newsroom/2024/mount-sinai-researchers-awarded-41-million-nih-grant-to-advance-understanding-of-sleep-apnea-using-artificial-intelligence. Published Feb. 12, 2024. Accessed Feb. 12, 2024.