Artificial intelligence improves detection during chest X-ray interpretation
Key takeaways:
- When assisted by artificial intelligence, physicians achieved a higher diagnostic accuracy in interpreting chest radiographs.
- Physicians’ clinical decisions did not differ based on AI assistance.
Assistance from artificial intelligence enhanced nonradiologists’ ability to detect lung lesions on chest radiographs, according to study results published in Annals of the American Thoracic Society.
“Physicians showed a better performance in [chest radiography] interpretation with AI assistance than without it,” Hyun Woo Lee, MD, of the division of respiratory and critical care in the department of internal medicine at Seoul Metropolitan Government-Seoul National University Boramae Medical Center, and colleagues wrote. “AI assistance allowed physicians to find more lung lesions.”
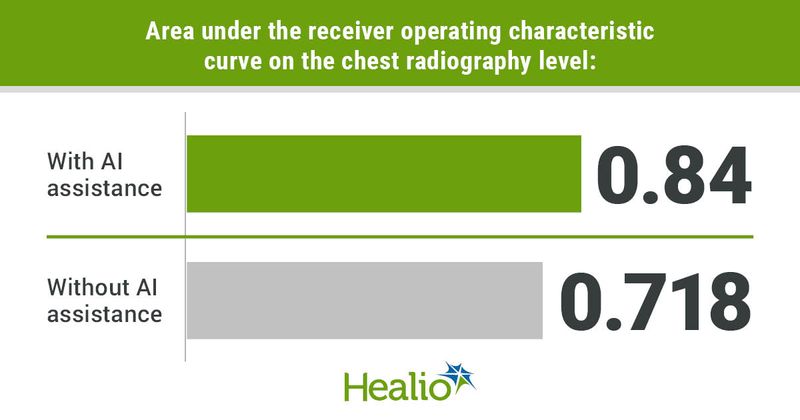
In a multicenter, prospective randomized clinical trial, Lee and colleagues analyzed chest radiographs with (n = 162; mean age, 64.8 years; 45.7% women) or without (n = 161; mean age, 65.7 years; 54% women) AI-assisted interpretation to determine how this element impacts the diagnostic accuracy — determined by a consensus reading of three thoracic radiologists — and clinical decisions of seven nonradiologist physicians from three different institutions.
To compare diagnostic and detection abilities, researchers evaluated the area under the receiver operating characteristic curve (AU-ROC).
Almost a quarter (23.8%) of the cohort had at least one previously diagnosed lung disease at baseline, whereas 31.9% reported a minimum of one respiratory symptom.
On the chest radiography level, researchers found a higher AU-ROC for the detection of any lung lesion with vs. without AI-assisted interpretation (0.84; 95% CI, 0.778-0.903 vs. 0.718; 95% CI, 0.64-0.796; P = .017), as well as increased sensitivity (87%; 95% CI, 64%-100% vs. 64%; 95% CI, 46%-87%; P = .004) and negative predictive value (92%; 95% CI, 84%-97% vs. 75%; 95% CI, 65%-83%; P = .003).
AI assistance also contributed to a greater AU-ROC on the lung lesion level (0.8; 95% CI, 0.74-0.861 vs. 0.677; 95% CI, 0.605-0.75; P = .011), with higher sensitivity (75%; 95% CI, 58%-95% vs. 58%; 95% CI, 44%-76%; P = .018) and negative predictive value (80%; 95% CI, 72%-87% vs. 63%; 95% CI, 53%-72%; P = .005) compared with no AI assistance.
In another analysis, researchers evaluated diagnostic accuracy using chest CT findings conducted within 2 weeks as the reference standard. They did not observe any significant between-group differences on the chest radiography level (n = 87) but found several between-group differences on the lung lesion level (n = 127).
Specifically, AI-assisted interpretation showed higher AU-ROC (0.869; 95% CI, 0.783-0.955 vs. 0.695; 95% CI, 0.583-0.807; P = .017), specificity (81%; 95% CI, 62%-94% vs. 56%; 95% CI, 38%-74%; P = .039) and positive predictive value (88%; 95% CI, 74%-96% vs. 63%; 95% CI, 46%-78%; P = .01).
Additionally, researchers found a decreased number of false referrals with AI-assisted interpretation (2.1% vs. 6.6%; P = .021) at this level.
In terms of clinical decisions, interpretation with or without AI resulted in similar rates of chest CTs, bronchoscopies and biopsies/surgeries, according to researchers.
“AI assistance for [chest radiography] interpretation can be a good auxiliary diagnostic tool for nonradiologist physicians by increasing sensitivity and decreasing false positivity and false referral rates,” Lee and colleagues wrote. “However, introducing AI assistance may increase unnecessary medical care or medical expenses, as it does not significantly benefit physicians’ clinical decisions.”
As AI becomes more prevalent in today’s world, this study by Lee and colleagues positively demonstrates its use in pulmonary imaging, according to an accompanying editorial by Sundaresh Ram, MS, PhD, research assistant professor in the department of radiology at University of Michigan, Ann Arbor, and Sandeep Bodduluri, MS, PhD, assistant professor of medicine in pulmonary, allergy and critical care medicine at The University of Alabama at Birmingham.
“The current study presents an interesting use of AI in pulmonary imaging and serves as a complementary diagnostic tool, providing a way to spur much-needed action for assessment of pulmonary disease that remains challenging in routine clinical practice for nonradiologist physicians,” Ram and Bodduluri wrote. “This study helps us understand some of the exciting potential of this methodology, and we look forward to more implementation studies to understand the impact of AI on the workflow of the nonradiologist physician.”