Recorded cough sounds may indicate COVID-19 disease severity
Key takeaways:
- Several frequency-based parameters of coughing differed among patients with mild, moderate or severe COVID-19.
- An additional two parameters differed by disease severity when dividing patients by sex.
Evaluating cough sounds of patients with COVID-19 could help determine the severity of their condition and lead to quicker treatment for those with severe disease, according to results published in ERJ Open Research.
“This approach can aid in the prompt identification and isolation of COVID-19 patients, thus facilitating proper medical care and the implementation of control measures,” Joaquim Gea, MD, PhD, emeritus head of the pneumology service at the Hospital del Mar Research Institute, said in a press release.
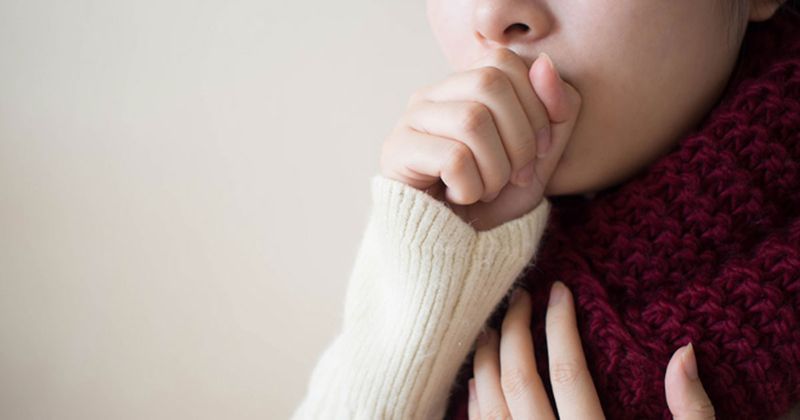
In a cross-sectional study, Gea and colleagues assessed 62 patients (37% women) aged older than 18 years with COVID-19 to determine if cough sounds recorded in the first 24 hours of hospital admittance via smartphone could be used to find those with severe disease.
Researchers used gas exchange abnormalities to group patients by disease severity level, which included mild (n = 13; median age, 52 years; 35% women), moderate (n = 14; median age, 51.5 years; 36% women) and severe (n = 17; median age, 54 years; 41% women).
With every cough effort signal, researchers collected various time- and frequency-based variables to then be evaluated in linear mixed-effects models.
Researchers defined a “cough bout” as at least two cough efforts following a single initial inspiration, with each cough effort having a first sound followed by an intermediate part and a second sound.
When assessing sounds from the recordings, researchers identified five parameters that significantly differed by whether the patient had mild, moderate or severe pneumonia.
These parameters included frequency variability (mild, 760.3 Hz vs. moderate, 767.4 Hz vs. severe, 614.9 Hz; P = .0031) and peak frequency (473.9 Hz vs. 340.1 Hz vs. 610.3 Hz; P = .0025) in the whole cough effort signal; frequency variability (729.5 Hz vs. 601.9 Hz vs. 526.7 Hz; P = .001) and frequency of maximum energy (2,191.8 Hz vs. 1,898.4 Hz vs. 1,620.4 Hz; P = .013) in the first cough sound; and the interquartile range of frequency (674.7 Hz vs. 825.7 Hz vs. 527.6 Hz; P = .026) in the intermediate part of a cough sound.
Notably, comparing those with mild disease with those with severe disease resulted in significant differences for each of the five parameters, whereas comparing those with moderate disease with those with severe disease resulted in significant differences in only three of the five parameters (frequency variability and peak frequency in the whole cough effort and the interquartile range of frequency in the intermediate part of a cough sound).
Divided by sex, researchers observed significant differences between the severity levels for two different parameters of sound. In the intermediate part of a cough sound, frequency variability differed in both men (mild, 697.2 Hz vs. moderate, 752 Hz vs. severe, 588.6 Hz; P = .0131) and women (1,054.5 Hz vs. 768.8 Hz vs. 597.5 Hz; P < .0001). In just women, frequency of maximum energy in the intermediate part of a cough sound differed between severity levels (3,567 Hz vs. 2,691 Hz vs. 2,190 Hz; P < .001).
“Using machine learning techniques and perhaps adding some extra features, the potential of this approach for discriminating different severities could be confirmed,” Gea and colleagues wrote. “This could result in an early stratification and prediction of probable clinical outcomes to triage correctly and allocate health care resources accordingly, which would be of huge benefit for both patient and health care providers.”
Reference:
- Cough sound analyzed to identify the severity of COVID-19 patients. https://ibecbarcelona.eu/cough-sound-analyzed-to-identify-the-severity-of-covid-19-patients/. Published Sept. 21, 2023. Accessed Sept. 21, 2023.