AI-enhanced breath analysis has ‘good performance’ in detecting COVID-19
Key takeaways:
- AI-selected clinical data plus breath analysis data resulted in improved distinction of COVID-19 in patients.
- Notably, this method had 98% sensitivity and a 98% negative predictive value.
Use of real-time mass spectrometry breath analysis that has been enhanced by artificial intelligence is a potential COVID-19 screening method, according to a presentation at the European Respiratory Society International Congress.
“We found that this method had good performance to detect COVID-19 with high-throughput, because ... the test’s length is less than 1 minute,” Stanislas Grassin Delyle, PhD, professor and hospital practitioner at Hôpital Foch in France and director of the health biotechnology department at UFR Simone Veil Santé, said during his presentation.
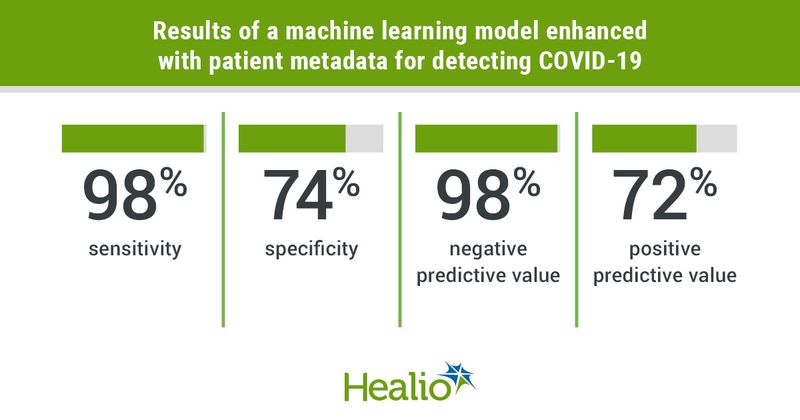
“For this test, due to its high predictive value, it can be used to quickly identify COVID-19-negative people and then maybe use confirmatory tests for positive people with a reference method,” Grassin Delyle added.
In two prospective, open, single-center, interventional studies, Grassin Delyle and colleagues assessed 173 adults who needed to be screened for COVID-19 to evaluate the efficacy of an artificial intelligence (AI)-enhanced real-time mass spectrometry breath analysis. The breath analysis used real-time proton transfer reaction time-of-flight mass spectrometry to perform a metabolic analysis of volatile organic compounds in exhaled breath. Researchers then applied this breath analysis data alone or combined with patient metadata to AI and machine learning techniques that built mathematical models.
Also, patients completed a reverse transcription polymerase chain reaction (RT-PCR) test for comparison.
Based on RT-PCR results, 67 patients (mean age, 56 years) tested positive for COVID-19, whereas 106 patients (mean age, 46 years) had a negative test.
Before adding the patient metadata, researchers found that their method had 94% sensitivity, 70% specificity, a 95% negative predictive value and a 67% positive predictive value for differentiating between COVID-19-positive vs. -negative patients.
When tested again with the addition of AI-selected patient clinical data (symptom score, past corticosteroid treatment and vaccination status), this model had better diagnostic performance, as demonstrated by an area under the receiving operating curve of 0.961, 98% sensitivity and 74% specificity in distinguishing COVID-19, according to Grassin Delyle.
Further, researchers observed an improved negative predictive value (98%) and positive predictive value (72%) compared with the prior model.
Across three different symptomatic conditions, the predictive performance of this model appeared comparable. Additionally, researchers did not observe bias in the model’s predictions based on COVID-19 vaccination status.
“Our results established a specific breath metabolomic signature which, when combined with clinical metadata, allowed reliable, noninvasive, high-throughput COVID-19,” Grassin Delyle and colleagues wrote in the study, which was simultaneously published in ERJ Open Research. “We now intend [to] embed all the hardware control and artificial intelligence tools for breath and online data analysis in a user-friendly, automated software package so that staff with basic training can screen for COVID-19 in less than 1 minute per person. This set-up could be used in subsequent validation and extension studies.
“A noninvasive breath analysis workflow with low consumable use (disposable mouthpieces only) and a rapid turn-around time might have health economic advantages over existing methods by rapidly identifying cases, halting the spread of the virus and enabling the provision of appropriate care to ill people,” Grassin Delyle and colleagues added.