Q&A: Assessing body composition on lung cancer screening improves mortality predictions
Key takeaways:
- Body composition attributes can be measured in a lung screening low-dose CT scan using artificial intelligence.
- Measures of subcutaneous adipose tissue and skeletal muscle improved mortality risk predictions.
Assessment of body composition measurements on lung screening low-dose CT scans revealed patients at high risk for mortality due to lung cancer, cardiovascular disease and all causes, according to results published in Radiology.
Kaiwen Xu, MSc, PhD candidate in the department of computer science at Vanderbilt University, and colleagues found this result by using 20,768 CT scans from the National Lung Screening Trial and an artificial intelligence (AI) algorithm that identifies body composition measurements from these scans.
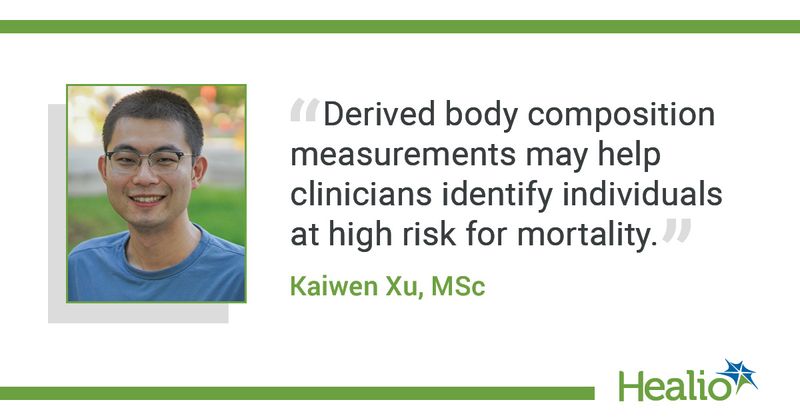
Compared with Cox proportional hazards models not including the AI-derived information, models with this information yielded better mortality risk predictions.
Healio spoke with Xu to learn more about the use of body composition measurements, challenges with implementing the AI prediction model in clinical use and how AI will continue to advance disease/death prediction models.
Healio: Why did you decide to include body composition in the AI prediction model? What measurements did you include?
Xu: Abnormal body composition phenotypes, such as obesity and sarcopenia, are associated with several chronic health conditions, including metabolic disorders, chronic inflammation and physical dysfunction. In lung cancer therapy, body composition has been shown to affect survival following chemotherapy, overall survival, quality of life and other essential oncologic metrics. Previous studies also demonstrated the risk stratification and prognostic value of body composition for cardiovascular disease and COPD.
The AI algorithm characterizes subcutaneous adipose tissue (SAT) and skeletal muscle (SM) from lung cancer screening low-dose CT of the chest. The characterization was performed on the CT sections at the levels corresponding to the fifth, eighth and 10th thoracic vertebral bodies. For both SAT and SM, three metrics were considered: 1) the area index, defined as the summed cross-sectional area across three levels divided (normalized) by patient height squared; 2) the mean attenuation, or radiodensity, defined as the average image intensity of the tissue; and 3) the standard deviation, defined as the standard deviation of image intensity of the tissue.
Healio: How does looking at body composition add to the value of lung cancer screening and potentially improve patient outcomes?
Xu: The primary indication of the low-dose chest CT scans performed during lung cancer screening is early detection of lung cancer. Our analysis suggested that these CT scans can also be utilized for body composition assessment that can improve risk stratification for other morbidities, including future lung cancer death, cardiovascular disease death and all-cause mortality.
Healio: How should everyday clinicians interpret the study’s findings? Are there any challenges to implementing the AI prediction model into the current pathway for interpreting low-dose CT scans for lung cancer screening?
Xu: The findings in this study suggest that the derived body composition measurements may help clinicians identify individuals at high risk for mortality who may benefit from targeted interventions.
There are still some barriers to seeing its application in routine clinical use. One prohibitive factor is the lack of standardized cut-off values to define what is normal and what is not. The cut-off values may depend on age, sex and race/ethnicity, and need to be characterized in subsequence population-scale studies on an application basis. Other challenges could include the build of the data processing infrastructure in clinical settings, potential bias and generalization issues when applying to a shifted patient population, regulatory approval and buy-in from clinicians and patients for the new technology.
Extensive discussion on this topic can be found in the related editorial by Fintelmann, as well as in a recent Radiology review by Pickhardt and colleagues.
Healio: Are there other measures from CT images that may play a role in predicting risk for mortality? Do you have any plans for related future studies?
Xu: In addition to body composition, we included the coronary artery calcification and quantitative emphysema in this study, both characterized using fully automatic methods that have been previously validated on this type of CT images. As a limitation, other incidental imaging data that are available and known to impact survival have not been included. These include bone mineral density and liver steatosis, as well as lung abnormalities other than emphysema (eg, interstitial lung abnormalities).
The presented study considered baseline screening only, but lung cancer screening is typically conducted in a longitudinal setting. It would be of considerable interest to follow the participants longitudinally and see if the change in body composition over time can improve the prediction for future health outcomes.
Healio: How will AI continue to advance disease/death prediction models?
Xu: Our study represents a type of clinically relevant AI research that seeks to automatically extract explainable and clinically relevant features from high-volume medical data and analyze if these features are relevant to certain diseases. In our case, this is the body composition measurements from lung cancer screening low-dose CT. This type of AI research could advance our understanding of underlying disease risk factors by enabling automatic data analysis at population-scale, which may not be feasible without AI.
Another attractive feature is that the fully automatic AI solutions can make it much easier for implementation in routine clinical practice compared with classic manual semiautomatic approaches.
References:
- AI uses lung CT data to predict mortality risk. https://www.rsna.org/news/2023/july/ai-predicts-mortality-risk-using-lung-ct-data. Published July 25, 2023. Accessed July 25, 2023.
- Fintelmann FJ. Radiology. 2023;doi:10.1148/radiol.231205.
- Pickhardt PJ, et al. Radiology. 2023;doi:10.1148/radiol.222044.
- Xu K, et al. Radiology. 2023;doi:10.1148/radiol.222937.
For more information:
Kaiwen Xu, MSc, can be reached at kaiwen.xu@vanderbilt.edu.