Breath data, simple clinical factors identify patients with non-small cell lung cancer
Key takeaways:
- Researchers could differentiate those with lung cancer from those without by factoring in exhaled breath data in a prediction model.
- Knowledge of sex, age and pack-years improved lung cancer discrimination.
A prediction model that incorporates both exhaled breath data from an electronic nose and basic demographics was able to identify lung cancer in a cohort of patients, according to study results published in CHEST.
“Combining exhaled breath data and clinical parameters in a multicenter, multi-device validation study can adequately distinguish patients with lung cancer from subjects without lung cancer in a noninvasive manner,” Sharina Kort, MD, PhD, of the department of respiratory medicine at Medisch Spectrum Twente in Enschede, the Netherlands, and colleagues wrote. “This study paves the way to implement exhaled breath analysis in the daily practice of diagnosing lung cancer.”
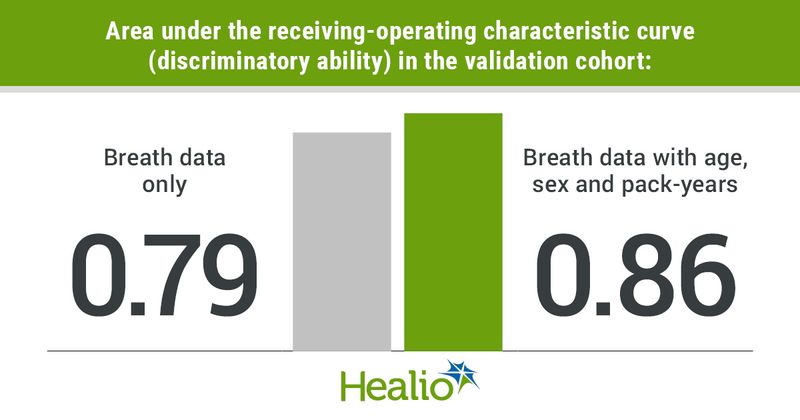
In a multicenter validation study, Kort and colleagues assessed the abilities of a prediction model that used exhaled breath patterns and clinical factors to differentiate lung cancer from non-lung cancer in a cohort of 575 individuals who had non-small cell lung cancer, were suspected but negative for lung cancer or were healthy.
Researchers obtained breath data from all patients with an electronic nose device, aeoNose (The eNose Company), that measured 5 minutes of their tidal breathing and then evaluated volatile organic compounds (VOCs).
As Healio previously reported, "VOCs are degradation products of biochemical processes in the body, and changes in VOC composition may be indicative of pathologies such as cancer."
Of the entire cohort, 376 individuals (160 with lung cancer; 51 with suspected, negative lung cancer; 165 healthy) belonged to the training set, which was where the model was established, and 199 individuals (79 with lung cancer; 32 with suspected, negative lung cancer; 88 healthy) belonged to the validation set. Notably, the validation cohort was blinded.
To test the model’s diagnostic ability, researchers evaluated the area under the receiver-operating characteristic curve (AUC-ROC).
Results
With only breath data and a cutoff value of –0.36 for determining lung cancer, researchers found that the model tested in the training set had an AUC-ROC of 0.83 (95% CI, 0.79-0.87), 91% sensitivity, 54% specificity and 89% negative predictive value. Slightly lower values were observed in the validation cohort with an AUC-ROC of 0.79 (95% CI, 0.72-0.85), 88% sensitivity, 52% specificity and 87% negative predictive value.
Compared with the model including only breath data, the model factoring in only sex, age and number of pack-years demonstrated lower predictive abilities in both the training cohort (AUC-ROC, 0.67; 95% CI, 0.61-0.72) and validation cohort (AUC-ROC, 0.75; 95% CI, 0.68-0.82).
However, researchers found improved diagnostic ability with the training model that factored in both breath and simple clinical data in multivariable logistic regression analysis (AUC-ROC, 0.87; 95% CI, 0.83-0.9). Notably, the cutoff probability of lung cancer was 16%. Improved or similar values to the model with only breath data were also observed when assessing sensitivity (95%), specificity (51%) and negative predictive value (94%) of the joint data model.
This model demonstrated comparable performance in the validation set with a 0.86 (95% CI, 0.81-0.91) AUC-ROC, 95% sensitivity, 49% specificity and 94% negative predictive value using the same cutoff probability, according to researchers.
Lastly, the Mayo Clinic nodule calculator, which factors in “age, smoking history, previous malignancy, nodule size, upper lobe spiculation and PET scan results,” was tested against the study’s model that included breath data, sex, age and pack-years.
Due to limited availability of data needed for the Mayo Clinic tool, only 55 patients with suspected lung cancer were included. The cutoff probability of lung cancer was again set at 16%, according to researchers.
Researchers found that their model showed better sensitivity than the Mayo Clinic model (94.6% vs. 83.8%) but worse specificity (22.2% vs. 61.1%), a lower positive predictive value (71.4% vs. 81.6%) and a similar negative predictive value (66.7% vs. 64.7%).
“Validation of a prediction model, as performed in this study, is a pivotal step for clinical integration of exhaled breath analysis in the diagnostic path of lung cancer,” Kort and colleagues wrote. “To assess the feasibility and acceptability of the electronic nose in clinical practice, we envision using the aeoNose in parallel with current practice in a hospital setting.”
The researchers further wrote that, despite being based on an exploratory analysis, the validated model exhibited the ability to accurately identify early-stage lung cancer from non-lung cancer.
“This finding supports the potential of the model to have a possible role in screening purposes,” Kort and colleagues continued. This would have to be investigated in the target population, and, in all likelihood, a different cutoff value needs to be chosen. In case of doubt (eg, based on CT scans), and a low probability of lung cancer, based on the validated model, a wait and see strategy could be used.”
Future of electronic noses
This study by Kort and colleagues adds to growing literature on electronic noses as a potential tool for detecting lung cancer and improving conditions for patients both with and without cancer, according to an accompanying editorial by Michael N. Kammer, PhD, research assistant professor of medicine in the division of allergy, pulmonary and critical care medicine at Vanderbilt University, and Rafael Paez, MD, clinical fellow in the division of allergy, pulmonary and critical care medicine at Vanderbilt University.
“With high enough specificity, an eNose device could serve to rule out cancer in patients with an incidentally detected lung nodule who might otherwise undergo an invasive diagnostic procedure,” Kammer and Paez wrote. “In this context, the eNose device could be the next step in management. Another future direction might include the combination of exhaled VOC analysis with other noninvasive biomarkers such as imaging or blood. Finally, after externally validating the previously locked and published VOC-based model, a clinical utility study would be necessary to demonstrate improvement in patient-centered outcomes.”