Machine-learning model superior in community-acquired pneumonia mortality prediction
Key takeaways:
- A machine-learning model predicted mortality in patients with community-acquired pneumonia.
- This model performed better than commonly used scoring systems.
A machine-learning model showed excellent discriminative ability in predicting 30-day mortality in patients with community-acquired pneumonia, according to study results published in CHEST.
“[SepsisFinder adapted for CAP with machine learning] offers some advantages over current scoring methods, for example, calculations easily made on routinely collected data and based on structured [electronic health record] data (vs. subjective criteria and arterial blood gas sampling needed for Pneumonia Severity Index [PSI], for instance); and tunable performance allowing risk cutoffs to be tailored to workflow requirements and the capacities of the individual institution (compared with fewer states in CURB-65),” Catia Cilloniz, PhD, senior investigator at the Hospital Clinic of Barcelona and associate professor at the University of Barcelona, and colleagues wrote. “In addition, SeF-ML performance seems not to depend on data availability, therefore allowing for more effective calculation of risk scores for CAP based on data sources with limited access to or completeness of certain variables.”
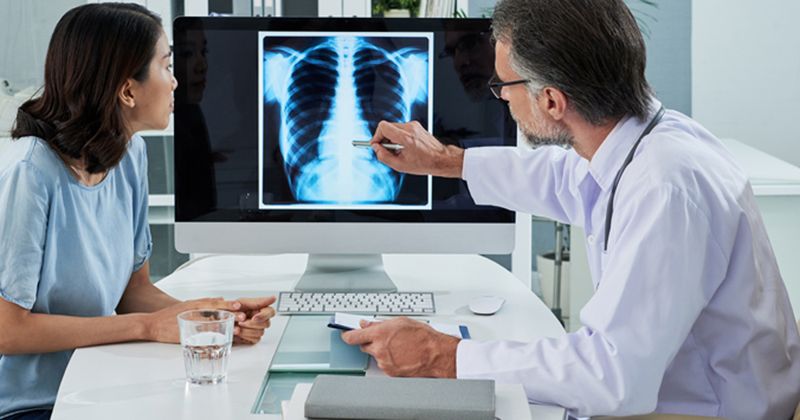
In a derivation-validation retrospective study, Cilloniz and colleagues analyzed 5,565 adults hospitalized with CAP from two hospitals in Spain to see if a causal probabilistic network (CPN) model offers a more accurate prediction of 30-day mortality compared with other severity scoring systems.
To predict mortality in patients with CAP, researchers used the SepsisFinder (SeF) model, a CPN for patients with sepsis that can predict mortality, but modified it for CAP (SeF-ML) by including respiratory rate, oxygen saturation (SpO2), bilirubin, blood urea nitrogen/urea, pH, hematocrit, partial pressure of oxygen, fraction of inspired oxygen, bicarbonate and leukocytes.
Researchers then looked at their machine-learning model against PSI, sequential organ failure assessment (SOFA), quick sequential organ failure assessment (qSOFA) and CURB-65 criteria (confusion, urea, respiratory rate, blood pressure, aged 65 years or older) scores.
To compare predictive abilities, researchers evaluated the area under the receiver operating characteristic curves.
Of the total cohort, 4,531 (median age, 73 years; 60% men) belonged to the derivation cohort, and 1,034 (median age, 72 years; 62% men) belonged to the validation cohort.
Researchers found that SeF-ML had the highest area under the curve (AUC) in the derivation cohort at 0.801, classified as excellent discrimination ability, among CURB-65 (0.759; P < .001), SOFA (0.671; P < .001), and qSOFA (0.642; P < .001).
There was no significant difference between the AUC found for SeF-ML and PSI (0.799), but researchers noted that only 58% of patients had PSI data.
In terms of calibration, researchers reported that this model was “visually well calibrated,” but according to the Hosmer-Lemeshow goodness of fit test, this observed calibration might not be accurate (15.62; P = .048).
The validation cohort showed a comparable AUC of the SeF-ML model at 0.826, which was significantly greater than the AUC found for CURB-65 (0.764; P = .03) and qSOFA (0.729; P = .005). Findings from this cohort and the derivation cohort demonstrate that SeF-ML offers improved 30-day mortality predictions compared with qSOFA and CURB-65 scoring systems, according to researchers.
No significant differences were found when comparing SeF-ML to PSI (0.83) and SOFA (0.771), according to researchers.
In this cohort, the model was “well calibrated” (Hosmer-Lemeshow statistic, 11.93).
Although additional validation is necessary, the SeF-ML model showed better discriminative capacity when differentiating those at high risk from those at low risk; it could identify high-risk patients with low CURB-65 and PSI scores and vice versa.
When evaluating the abilities of SeF-ML, researchers found that custom thresholds can be set and adjusted based on the care environment because of continuous probability output.
“Our findings need further validation in other cohorts from different settings to assess the actual clinical usefulness of SeF-ML in predicting CAP prognosis,” Cilloniz and colleagues wrote.
This study by Cilloniz and colleagues brings attention to a way to predict mortality in patients with CAP, but additional studies are needed to understand how it interacts with diagnostic or antibiotic stewardship, according to an accompanying editorial by Richard G. Wunderink, MD, FCCP, a professor of medicine in the division of pulmonary and critical care at Northwestern University’s Feinberg School of Medicine.
“The real validation is not whether the SeF-ML score predicts mortality better than the comparators, but whether diagnostic or antibiotic stewardship can be impacted by use of the score,” Wunderink wrote. “This SeF-ML validation will require a randomized trial of diagnostic test strategies based on the score vs. usual care, not simply comparison with other scores that were themselves developed for alternative purposes. Until then, the American Thoracic Society and Infectious Diseases Society of America guidelines recommendation for enhanced testing for critically ill patients and those thought to have risk factors, those initiated on broad-spectrum antibiotics or both is most likely to even the score between diagnostic and antibiotic stewardship.”