Q&A: Machine learning models predict risk for delirium in the ICU
By training artificial intelligence, or AI, algorithms with electronic health record data, researchers accurately predicted the risk for delirium among patients in the ICU, according to study results published in Anesthesiology.
Researchers created two machine learning models programmed with health record data from more than 200,000 ICU stays. The 24-hour model evaluated age, illness severity, physiologic variables and current medications following a patient’s admission into the ICU, whereas the dynamic model assessed blood pressure, pulse and temperature readings over the span of hours and days to predict the risk for delirium, according to a Johns Hopkins Medicine press release.
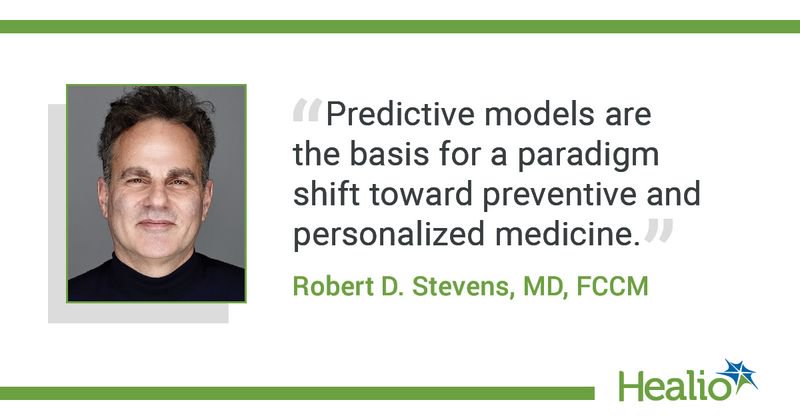
When assessing the performance of both models with data from more than 100,000 ICU stays, researchers found that the 24-hour model accurately predicted patients prone for delirium 78.5% of the time, and the dynamic model predicted these patients up to 90% of the time, according to the release.
To learn more about ICU delirium and these models, Healio spoke with senior author Robert D. Stevens, MD, FCCM, associate professor of anesthesiology and critical care medicine at The Johns Hopkins University School of Medicine.
Healio: What is ICU delirium and how prevalent is it?
Stevens: Delirium is defined as a change in brain function which occurs in susceptible individuals who are exposed to an acute illness or physiological disturbance. The hallmark features of delirium include an abrupt onset, the inability to maintain attention, other cognitive alterations (eg, disorientation, hallucinations) and a fluctuating course. The diagnosis of delirium can only be made after excluding other neurological conditions such as dementia or coma.
Delirium is particularly frequent in patients admitted to the ICU, with a mean prevalence of 31.8% according to a meta-analysis by Salluh and colleagues and reaching 85% in patients who are receiving mechanical ventilation. Delirium is even more frequent in patients admitted to ICU with severe forms of COVID-19, affecting greater than 50% in this subset (Pun BT and colleagues).
When it occurs in the ICU, delirium is independently associated with a doubling of mortality and a significantly higher likelihood of long-term cognitive decline.
Healio: What was the rationale behind developing an AI program/algorithm to predict risks of ICU delirium?
Stevens: Clinicians have limited means to accurately predict if a patient will actually develop delirium after admission to the ICU. The current approach is more reactive than proactive: If a patient develops delirium, then clinicians might respond. We hypothesized that signatures present in the clinical and physiological data could identify risk for transitioning to delirium in the future.
Healio: The study details two computerized models, the 24-hour model and the dynamic model, to predict risk for delirium. Could you explain how these models differ in terms of the data they collect and how they work together to predict delirium risk?
Stevens: The 24-hour model uses data available in the first 24 hours of intensive care to predict delirium at any subsequent time during the patient’s stay in the ICU. The dynamic model leverages cumulative data up to the time of prediction, to determine the probability that delirium will occur in the next 1 to 12 hours.
Healio: What were the most significant findings from your study?
Stevens: The principal finding is that AI algorithms trained with data routinely collected in clinical care can determine the likelihood that an ICU patient will have delirium with a high level of accuracy and precision. These models performed better than statistical models which have been tested and published previously. They were reproducible when validated in two external datasets.
Healio: Can you characterize what the impact of these findings are for the everyday clinician?
Stevens: Predictive models are the basis for a paradigm shift toward preventive and personalized medicine. With validation, clinicians will be able to leverage model outputs to mitigate risk for delirium through targeted interventions.
Healio: What are your plans for future studies?
Stevens: Several different studies are underway or planned. Studies will need to be prospective and integrate features which were unaccounted for in the current work. They will use AI to unpack the complexity or heterogeneity of delirium which encompasses many different sub-phenotypes, each with specific biological mechanisms and treatment responses. They will map ICU delirium risk assessment to specific antidelirium treatments or clinical pathways.
For more information:
Robert D. Stevens, MD, FCCM, can be reached at rstevens@jhmi.edu.
References:
- Gong KD, et al. Anesthesiology. 2022;doi:10.1097/ALN.0000000000004478.
- Johns Hopkins physicians and engineers develop search for AI program that accurately predicts risk of ‘ICU delirium’. https://www.hopkinsmedicine.org/news/newsroom/news-releases/johns-hopkins-physicians-and-engineers-search-for-ai-program-that-accurately-predicts-risk-of-icu-delirium. Published Jan. 26, 2023. Accessed Jan. 26, 2023.
- Pun BT, et al. Lancet Respir Med. 2021;doi:10.1016/S2213-2600(20)30552-X.
- Salluh JIF, et al. BMJ. 2015;doi:10.1136/bmj.h2538.