Q&A: Cough-detecting AI enables early pneumonia diagnosis in hospitals, homes
A new machine learning algorithm can detect whether an individual has pneumonia by recording coughs and measuring the acoustics of rooms and spaces, according to a study presented at the 183rd Meeting of the Acoustical Society of America.
Based on the data collected, this algorithm can be used in hospitals or at home to diagnosis people with pneumonia or other respiratory diseases, according to a society press release.
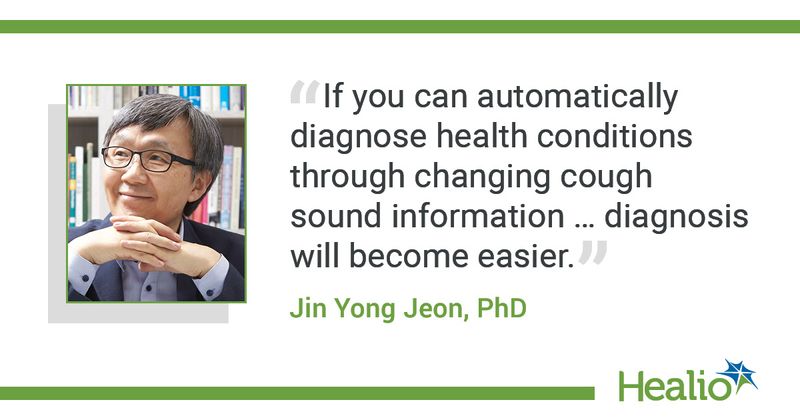
To learn more about this research, Healio spoke with Jin Yong Jeon, PhD, study researcher and head of the department of medical and digital engineering at Hanyang University in Seoul, South Korea, about how the algorithm works, the benefits it offers in real-world practice and plans for future studies.
Healio: What was the rationale behind developing a machine learning algorithm using cough sounds to diagnose pneumonia?
Jeon: Kim Sang-Heon, MD, PhD, professor and chief of internal medicine at Hanyang University Hospital, who proposed this study for the first time, often mentions that physicians in the past predicted respiratory diseases with hearing and fingertip sensations more accurately than stethoscopes.
This can be said to be a predictive model created by human learning through many medical field experiences along with the information of sound. Advances in artificial intelligence (AI) technology offer us the possibility of creating these models in a short period of time. In addition, if you can automatically diagnose health conditions through the changing cough sound information that continues to occur during daily life, diagnosis will become easier, including non-face-to-face medical treatment, and appropriate treatment selection based on the information you have obtained. The range of choices expands.
Overall, medical costs can be reduced in terms of providers (medical staff, developers) and consumers (users, patients), and more accurate and prompt diagnosis will continue to be possible through the information accumulated in the database.
Healio: What factors does the algorithm consider in order to correctly determine whether a patient has pneumonia?
Jeon: First, an impulse response convolution is obtained in an additional space (reverberant sound field according to various possible sizes and types of interior finishing materials) including the patient’s coughing sound collected in that space. Rather than arbitrarily expanding the data in the conventional way, we performed augmentation by changing the spatial element of the coughing sound. In this state, it is important to convert the sound field extended by numerical sound quality data into an image using Gramian Angular Fields and, as a result, combining Mel-spectrum and heterogeneous data consisting of 3D image dataset. In other words, efficiently generating data augmentation is key.
Healio: What were the most significant findings from your study?
Jeon: It is important to utilize Zwicker’s sound quality parameters of cough data augmented through spatial information (impulse responses) and frequency characteristic analysis data over time. We performed pattern analysis by constructing a convolutional neural networks model based on the image dataset that went through the conversion process in order to identify the pneumonic sound that has already been spatially and compositionally clearly analyzed and defined. In a situation where the size of various spaces and finishing materials change (spatial characteristics), AI recognizes the sound quality characteristics of the cough sound of a specific disease and the level characteristics (acoustic characteristics) according to time and frequency.
Healio: What are some of the potential benefits/advantages of this algorithm? How do you see it being implemented in real-world practice?
Jeon: Because data are augmented by utilizing room impulse responses, applicability can be increased to data that fluctuates no matter what environment it is recorded in depending on the space. In the field of speech recognition, it is used to control the distortion of sound by spatial elements, and there are cases where other augmentation methods also use impulse response. In the case of our research results, the performance of the AI model was optimized because the spatial shape was set in consideration of the homogeneity of the input data caused by the room mode in the spatial shape and the cough sound was analytically defined.
Healio: The abstract said that the results of your study are expected to be used to develop diagnostic algorithms for various respiratory diseases. What diseases are encompassed in that statement?
Jeon: We are trying to expand the cough sound, one of the representative symptoms of respiratory disease patients, to COPD or asthma. In addition, we are developing an Activities of Daily Living-based respiratory disease diagnosis algorithm by utilizing another technology we have, a sound-based data handling methodology. We are also developing an algorithm for counting the number of coughs for each individual to predict the condition through changes in cough conditions using an AI classification algorithm. In order to discriminate individual cough sound, we use autocorrelation function/interaural cross-correlation function, or ACF/IACF, together to advance the algorithm.
Healio: What are your plans for future studies?
Jeon: We are collaborating with the company HicareNet with plans to actively deal with deteriorating health of respiratory patients by applying it to Medicare remote patient monitoring in the United States. Through app and software development, it can be installed in the home care system to check the respiratory patient’s condition and respond appropriately. It was initially developed as a service that runs lightly within the app without using a server or cloud and was introduced at MEDICA in Germany last year. Our research team plans to work with companies to increase convenience and applicability by fully automating each step-by-step process that is currently manually carried out.
References:
- Jeon JY, et al. Presentation 1pCA8. Presented at: 183rd Meeting of the Acoustical Society of America. Dec. 5-9, 2022; Nashville, Tenn.
- Machine learning diagnoses pneumonia by listening to coughs #ASA183. https://acoustics.org/machine-learning-diagnoses-pneumonia-by-listening-to-coughs-asa183/. Published Dec. 5, 2022. Accessed Dec. 5, 2022.
For more information:
Jin Yong Jeon, PhD, can be reached at jyjeon@hanyang.ac.kr.