Deep learning may help predict mental disorder diagnosis
A multidiagnostic prediction model developed using deep learning was highly predictive of mental disorder diagnosis, according to new data published in JAMA Psychiatry.
“For mental disorders, machine learning models have shown great promise in predicting both diagnosis and prognosis within mental disorders using data sources such as genetics, MRI, EEG and clinical and demographic data,” Rosa Lundbye Allesøe, MSc, and colleagues wrote. “However, performance across studies between disorders is inconsistent with relatively small sample sizes, insufficient reporting of the model used, restriction to one type or data, no validation and has mainly been restricted to one diagnostic group or prognostic outcome. A common issue is that these do not resemble an actual clinical setting.”
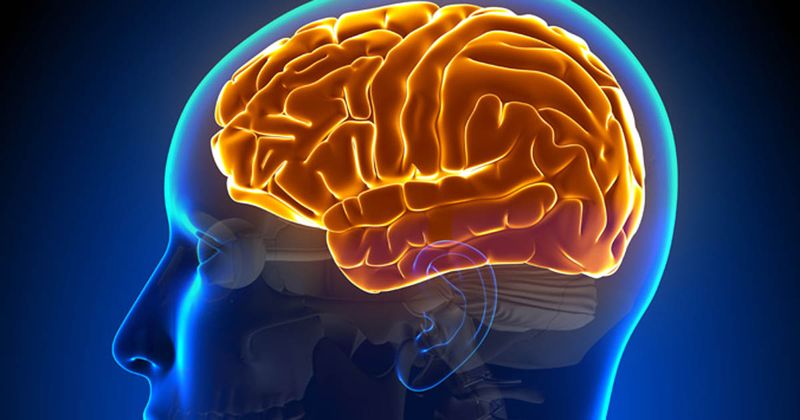
The researchers used genotype data and matched longitudinal health register data from the longitudinal Danish population-based Integrative Psychiatric Research Consortium 2012 case-cohort study. The study included all people in Denmark who had at least one hospital contact with a diagnosis of ADHD, autism spectrum disorder, major depressive disorder, bipolar disorder and schizophrenia spectrum disorders as well as population controls who were born between 1981 and 2005, with follow-up available through 2016.
Area under the curve, accuracy and Matthews correlation coefficient (MCC) were used to assess the power of both cross-diagnostic and single-disorder models to predict mental disorder diagnosis and disorder severity trajectories.
Of the 63,535 people included in the analysis, 12,878 had ASD, 15,969 had ADHD, 5,120 had schizophrenia spectrum disorders, 1,719 had bipolar disorder, 19,159 had MDD and 20,681 were controls.
Results showed that the multidiagnostic model could predict the specific mental disorder diagnosis from the background population with an AUC of 0.81, an accuracy of 44% and an MCC of 0.28. The single-disorder models, however, had an AUC of 0.84 and MCC of 0.54 for schizophrenia spectrum disorders, an AUC of 0.79 and MCC of 0.41 for bipolar disorder, an AUC of 0.77 and MCC of 0.39 for ASD, an AUC of 0.74 and MCC of 0.38 for ADHD and an AUC of 0.74 and MCC 0f 0.38 for MDD.
Allesøe and colleagues found that previous mental disorders and age, which were linked to an 11% to 23% reduction in prediction accuracy when removed, were the most important data sets for multidiagnostic prediction, followed by family diagnoses, birth-related measurements and genetic data.
When predicting postdiagnosis disorder trajectory by dividing the cases into five groups of increased severity, the AUC was 0.65, the MCC was 0.13 and the accuracy was 32%, with the most severe cases proving the easiest to predict, with an AUC of 0.72.
In light of these results, the researchers noted that such prediction models combining genetic and registry data may help improve clinical care.
“The model could eventually be implemented to summarize all historical data into an underlying probability for each mental disorder diagnosis that the clinician can use together with the regular assessment to provide more standardized care across clinics,” they wrote.