Predicting suicide risk in adults optimized with combined in-person, electronic models
Suicide risk prediction in adults was optimized when utilizing both in-person screening and electronic data, according to a study published in JAMA Network Open.
“Mental health diagnoses are often absent in records of those who subsequently die from suicide. Better risk identification and prognostication might improve outreach and prevention,” Drew Wilimitis, BS, of the department of biomedical informatics at Vanderbilt University in Nashville, and colleagues wrote.
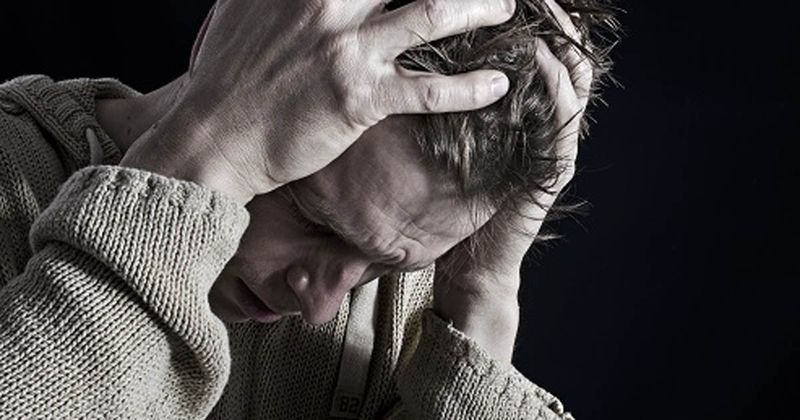
Researchers aimed to evaluate individual and combined effectiveness of the Columbia Suicide Severity Rating Scale (C-SSRS) and a machine learning model to accurately predict the risk of suicide attempt and suicidal ideation.
The observational cohort study analyzed 120,398 unique index visits for 83,394 adult patients (mean age, 51.2 years; 46% male; 77% white) between June 2019 and September 2020 from the Vanderbilt Research Derivative. The C-SSRS was given to each patient during routine care within inpatient, ambulatory surgical and ED settings, and a Vanderbilt Suicide Attempt and Ideation Likelihood (VSAIL) prediction was also generated for each. Primary outcomes were incidence of suicide attempt (SA) and suicidal ideation (SI), and the retrospective validity of the C-SSRS and VSAIL was analyzed, as well as models which combined both.
Discrimination metrics included area under the receiver operating curve (AUROC), area under the precision-recall curve (AUPR), sensitivity, specificity, positive predictive value (PPV), and negative predictive value (NPV).
Results indicated that within 30 days of an index visit, the combined models registered higher AUROC (SA: 0.874-0.887; SI: 0.869-0.879) than both the VSAIL (SA: 0.729; SI: 0.773) and C-SSRS (SA: 0.823; SI: 0.777) models.
In the highest risk-decile, combining methods registered PPV of 1.3- 1.4% for SA and 8.3-8.7% for SI and sensitivity of 77.6- 79.5% for SA and 67.4- 70.1% for SI, outperforming both VSAIL alone (PPV for SA: 0.4%; PPV for SI: 3.9%; sensitivity for SA: 28.8%; sensitivity for SI: 35.1%) and C-SSRS alone (PPV for SA: 0.5%; PPV for SI: 3.5%; sensitivity for SA: 76.6%; sensitivity for SI: 68.8%).
“Further research is needed to compare alternate ways of combining clinical and statistical risk prediction and to analyze the practical implications of implementing them,” Wilimitis and colleagues wrote.
In an editorial, Jordan E. DeVylder, PhD, of the graduate school of social service at Fordham University in New York City, wrote that “untangling the risks and benefits of suicide prevention approaches is difficult in cohort studies for several reasons, even when dealing with very large samples.”
However, he praised the researchers for producing new data on the benefits of combining both modes to facilitate decision-making on the best route in a clinical setting.
References:
- DeVylder JE. JAMA Netw Open. 2022;doi:10.1001/jamanetworkopen.2022.12106.
- Wilimitis D, et al. JAMA Netw Open. 2022;doi:10.1001/jamanetworkopen.2022.12095.