Model may help predict onset of myopia in children up to age 14 years
Key takeaways:
- Younger Asian girls had the highest probability of developing myopia, while older white boys had the lowest.
- Lower amounts of hyperopia also were associated with greater likelihood of myopia onset.
Refractive error, age, sex and race/ethnicity can be predictive factors in determining the probability of myopia onset in prepubescent children, according to a study published in Optometry and Vision Science.
“A predictive model would be useful for sample size planning in myopia prevention clinical trials, providing estimates of the likelihood of conversion from emmetropia to myopia, given different sample configurations of age, sex, race/ethnicity, baseline refractive error or other significant covariates,” Donald O. Mutti, OD, PhD, FAAO, the E.F. Wildermuth Foundation Professor in Optometry at The Ohio State University College of Optometry, and colleagues wrote. “A predictive model would help the clinician advise an individual child and their family on the probability of future refractive error.”
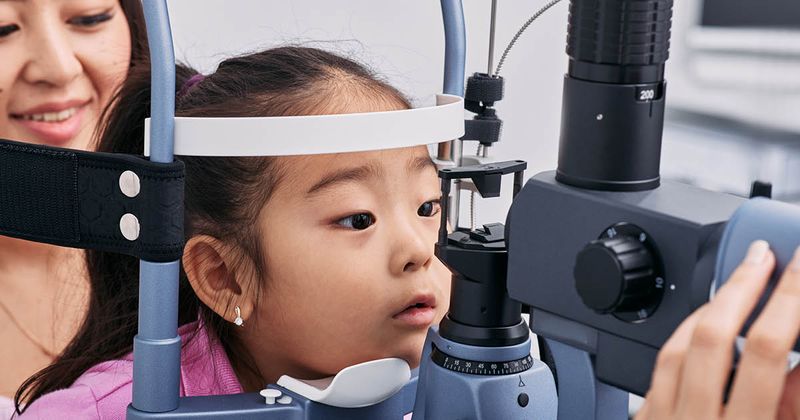
Using data from the multicenter Collaborative Longitudinal Evaluation of Ethnicity and Refractive Error (CLEERE) Study, Mutti and colleagues modeled the probability of myopia onset among children in the United States on a year-by-year and cumulative basis using spherical equivalent refractive error, the horizontal/vertical component of astigmatism, age, sex, race and ethnicity. They included 4,027 nonmyopic study participants aged 6 to 14 years at baseline (49.9% girls), who were monitored for 1 to 7 years through eighth grade.
According to results, the proportion of children who developed myopia by age 14 years was highest among those who were younger at baseline, with 18.9% of those aged 6 years and 19.9% aged 7 years developing myopia compared with 5% aged 13 years at baseline. Younger Asian girls had the highest probability of developing myopia, while older white boys had the lowest.
Lower amounts of hyperopia and less positive values of horizontal/vertical component of astigmatism also were associated with greater likelihood of myopia onset.
“Efforts toward harmonization of prediction calculators will be useful as more longitudinal studies become available,” Mutti and colleagues wrote. “In addition to providing a consensus predictive model, another aspect of harmonization of various longitudinal datasets would be to determine if the probabilities attached to the various risk factors have changed over time.”