ChatGPT-4 accurately interprets ophthalmic images
Click Here to Manage Email Alerts
Key takeaways:
- ChatGPT-4 correctly answered most multiple-choice questions pertaining to image recognition in ophthalmic cases.
- The chatbot performed better on nonimage-based vs. image-based questions.
The latest version of an artificial intelligence chatbot accurately responded to 70% of multiple-choice questions pertaining to ophthalmic cases based on imaging interpretation, according to a study published in JAMA Ophthalmology.
“As the use of multimodal chatbots becomes increasingly widespread, it is imperative to stress their appropriate integration within medical contexts,” Andrew Mihalache, MD candidate at the Temerty School of Medicine of University of Toronto in Ontario, Canada, and colleagues wrote.
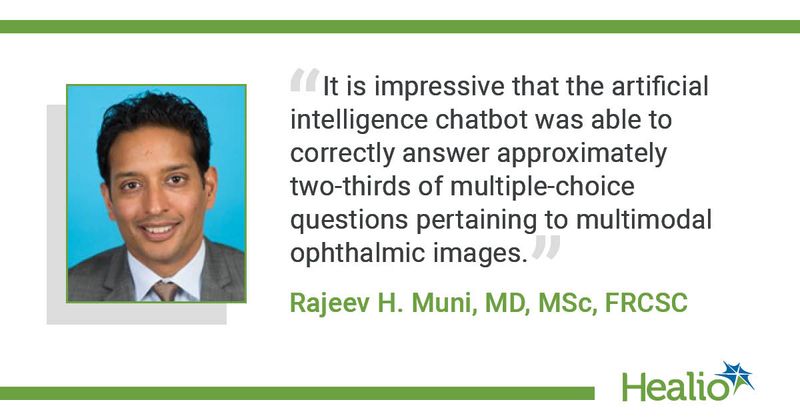
In a cross-sectional study including 136 ophthalmic cases and 448 images provided by the medical education platform OCTCases, researchers evaluated the performance of ChatGPT-4 (OpenAI), an AI chatbot capable of processing ophthalmic imaging data. They used multiple-choice questions in the statistical analysis rather than open-ended questions to allow for objective grading of the chatbot’s responses.
The primary endpoint was the chatbot’s accuracy in answering multiple-choice questions pertaining to image recognition in ophthalmic cases — organized into categories including retina, neuro-ophthalmology, uveitis, glaucoma, ocular oncology and pediatric ophthalmology — measured as the proportion of correct responses, according to the researchers.
Secondary endpoints included the differences in the chatbot’s performance on image- vs. nonimage-based questions, as well as the association between the number of images inputted and the proportion of multiple-choice questions answered correctly per case.
Researchers conducted χ2 tests to compare proportions of correct responses across different ophthalmic subspecialties.
Of the 429 multiple-choice questions included in the analysis, ChatGPT-4 answered 299 (70%) correctly across all cases.
“Given the complexity of ophthalmic image interpretation, it is impressive that the artificial intelligence chatbot was able to correctly answer approximately two-thirds of multiple-choice questions pertaining to multimodal ophthalmic images,” Rajeev H. Muni, MD, MSc, FRCSC, co-author and vice chair of clinical research in the university’s department of ophthalmology and vision sciences, told Healio. “Over time, improvements in AI models could improve the chatbot’s accuracy considerably.”
The chatbot performed better on retina questions than neuro-ophthalmology questions (77% vs. 58%; difference, 18%; χ21 = 11.4; P < .001).
The chatbot’s performance also appeared better on nonimage-based questions compared with image-based questions (82% vs. 65%; difference, 17%; χ21 = 12.2; P < .001).
Additionally, the chatbot demonstrated intermediate performance on questions from the ocular oncology (72% correct), pediatric ophthalmology (68% correct), uveitis (67% correct) and glaucoma (61% correct) categories.
“Our findings show that the artificial intelligence chatbot has the potential to serve as a valuable educational resource for clinicians and trainees one day, as it is capable of identifying and interpreting abnormalities present on ophthalmic imaging modalities with moderate accuracy,” Muni said. “As its knowledge and sophistication advances, the artificial intelligence chatbot may eventually play a role in clinical decision-making.”
The researchers noted that their present investigation, which assessed the AI chatbot’s performance on multiple-choice questions pertaining to multimodal ophthalmic cases, may not translate to its real-world clinical utility.
“Our team’s future work aims to assess the chatbot’s diagnostic accuracy on ophthalmic imaging cases without the use of multiple-choice prompts,” Muni added. “We also aim to compare the performance of the AI chatbot relative to board-certified ophthalmologists.”