AI approach predicts demographic, clinical features from meibography images
SAN DIEGO — A deep-learning model was able to predict patient demographics, clinical and subjective outcomes, and ocular surface health using meibography images, according to a study presented at Academy 2022.
Meng C. Lin, OD, PhD, FAAO, a professor at the Herbert Wertheim School of Optometry & Vision Science, director of the clinical research center and co-chief of the dry eye clinic at the University of California, Berkeley, shared these study findings during a virtual academy-sponsored press conference.
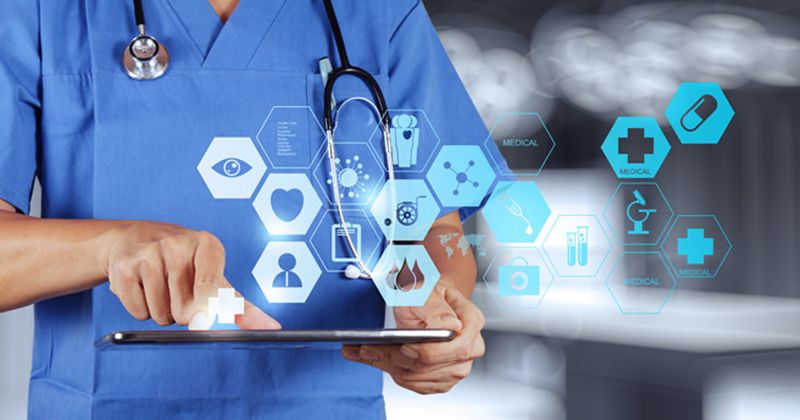
“Changes in meibomian gland morphology are a leading cause of meibomian gland dysfunction,” Lin said. “Current meibography evaluation methods have a lack of standards and are time-intensive in nature, and little evidence is available to show downstream effects.”
Data-driven prediction models take three sources, she said: Meibography image, gland level features and meta data such as demographics.
The researchers collected 689 infrared meibography images from 363 patients (170 contact lens wearers, 193 non-wearers).
According to the study, Lin and colleagues trained a deep-learning model to de-identify meibography images and learn the morphological features of the meibomian gland, then predict demographics, clinical outcomes related to gland function, tear film stability, ocular surface health, and subjective outcomes related to discomfort and dryness.
Age and ethnicity were predicted with average accuracies of 75.6% for Asian patients and 85.8% for non-Asian patients, according to the study. In addition, meibomian gland morphological features ranged from 65% to 96% accuracy as heavily weighted predictors for eyelid notching and vascularization, meibomian gland expressate quality, extent of corneal staining and Visual Analogue Scale (VAS) average comfort rating.
“Meibography images can predict expert clinical diagnosis of MGD with 75% to 85% accuracy using the number of visible glands as the most highly weighted predictor,” Lin said. “Lower gland density, fewer visible glands and more gland atrophy were all the top features used to predict clinical signs. However, they cannot predict dry eye symptoms with a high level of confidence.”
Lin concluded: “Machine learning is able to discover more relationships between meibomian gland morphology and clinical signs compared to current methods. It can detect patient age and ethnicity from algorithms.
“It is possible meibography images can soon be used as a biometric fingerprint to identify an individual patient,” she added.