AI program successfully identifies posterior vitreous opacities in OCT images
Click Here to Manage Email Alerts
An AI program accurately identified posterior vitreous opacities in patients with posterior vitreous detachments, which may improve screening for retinal tears, according to a study.
“Previous studies have already demonstrated that PVOs are predictive for retinal tears, and we have now demonstrated that AI systems can automatically detect PVOs on OCTs as well,” Royce W.S. Chen, MD, of Columbia University Irving Medical Center, said at the American Society of Retina Specialists annual meeting.
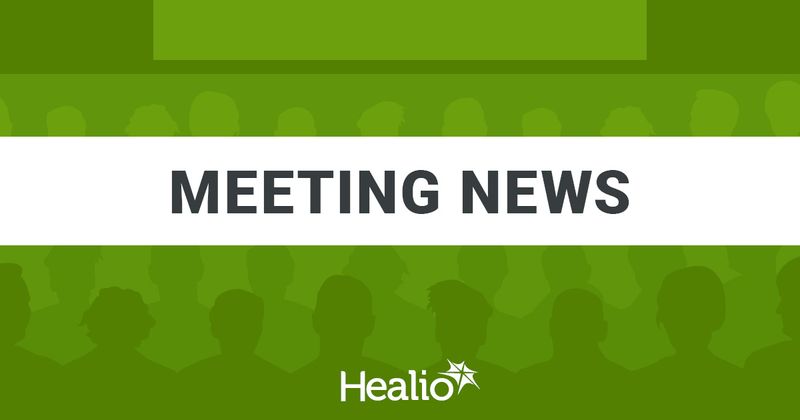
The study collected 500 spectral domain OCT scans of 99 eyes of 93 patients with acute, symptomatic posterior vitreous detachments (PVDs). Two trained graders reviewed each scan and identified all cases of posterior vitreous opacities (PVOs), classifying severity as no PVOs, low (one to four PVOs), medium (four to 15 PVOs) or high (15 or more opacities). A 2D U-Net model was then trained to identify PVOs among the same images.
Overall, the mean PVO count in the 10.1% of eyes identified as having retinal tears was 96 ± 82 compared with 4 ± 6 in eyes without retinal tears. The deep learning model was “very accurate,” Chen said, with a sensitivity of 78% and a specificity of 95.7% in the medium severity PVO group. In addition, the learning model demonstrated 88% sensitivity and 65.4% specificity in the low severity group and 50% sensitivity and 100% specificity in the high severity group.
“The deep learning model consistently and accurately identified PVOs across all severities,” Chen said. “It is therefore possible now that OCTs placed in community practices or in pharmacies or in general medical practices may help screen in at-risk patients with acute, symptomatic PVDs and efficiently direct them to retinal specialists for further evaluation.”