Deep learning model predicts risk for distant recurrence of endometrial cancer
Key takeaways:
- The model can be applied to standard workflow using just one histopathologic tumor slide and stage, according to researchers.
- It outperformed a Cox hazards model with histopathologic and molecular variables.
A deep learning model accurately identified women with endometrial cancer at low and high risk for distant recurrence, according to research presented at American Association for Cancer Research Annual Meeting.
“[The model] can be put into a standard workflow using only H&E [hematoxylin and eosin] tumor slide and stage,” Sarah Fremond, MSc, a PhD candidate in the department of pathology at Leiden University Medical Center in the Netherlands, said during a presentation.

Background and methods
Surgery remains the primary treatment for endometrial cancer, the most common gynecologic cancer. Adjuvant chemotherapy is recommended for women at risk for distant recurrence, but causes morbidity, Fremond said.
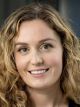
“The clinical question that we tackle in this study is, how do we accurately identify patients at low and high risk [for] distant recurrence, to reduce under- and overtreatment?” she said.
Assessing prognostic factors to determine risk carries challenges, including variability among pathologists evaluating histopathologic slides, and cost and turnaround time of molecular classification, according to Fremond.
To address these challenges, Fremond and colleagues created a deep learning model to predict risk for distant recurrence among women with endometrial cancer using routine diagnostic H&E tumor slides and stage. They named the model HECTOR (Histopathology-based Endometrial Cancer Tailored treatment and distant recurrence Outcome Risk prediction deep learning model).
The data set included 1,761 women enrolled in the PORTEC-1, -2 and -3 randomized trials and three clinical cohorts, excluding those who did not have stage IV disease and did not receive previous adjuvant chemotherapy. Median follow-up was 8 years.
Researchers trained and optimized HECTOR using one representative digitized H&E tumor slide from each of 1,408 patients, then tested its performance on an unseen test set of 353 patients.
They measured performance with the concordance index and compared it using Cox proportional hazards models fitted onto histopathologic variables and molecular class.
Results
HECTOR achieved a concordance index of 0.795 ± 0.031 on fivefold cross validation and 0.788 on the unseen test set. This outperformed the baseline Cox model with type, grade, lymphovascular space invasion and stage (0.723 ± 0.045 fivefold cross-validation, 0.771 unseen test set) and the baseline Cox model with those factors plus molecular class (0.736 ± 0.033 fivefold cross-validation, 0.778 unseen test set).
Among the unseen test set, HECTOR identified 81 patients as low risk, 176 as intermediate risk and 96 as high risk for 10-year distant recurrence. The predictions appeared consistent with outcomes.
Fremond described how the model would work in clinical practice using the case scenario of a woman with stage I endometrial cancer. Feeding HECTOR one digitized H&E tumor slide would produce three types of results: predicted probabilities of distant recurrence over time, a predicted HECTOR risk score, and — superimposed on the tumor slide — regions highly predictive of that risk score, she said.
Implications, next steps
During a press conference, Fremond said HECTOR has been externally tested on a diagnostic cohort of 152 women, for whom they had three tumor slides each. Among this cohort, HECTOR achieved a concordance index of 0.816, “a great performance,” she said.
HECTOR needs further external testing at other institutions and among minority patients, as well as validation in prospective studies, Fremond acknowledged.
The model could, theoretically, be used in other cancers, she said.
“I think you can get inspired by that multimodal, deep learning model and then apply it to another cancer type,” she said.
References:
- An investigational deep learning model may help stratify risk for patients with endometrial cancer (press release). Available at. Published April 18, 2023. Accessed April 18, 2023.
- Fremond S, et al. Abstract 5695. Presented at: American Association for Cancer Research Annual Meeting; April 14-19, 2023; Orlando.