AI tools for earlier pancreatic cancer diagnosis may yield ‘increasingly better outcomes’
Click Here to Manage Email Alerts
Use of artificial intelligence in medical imaging has increased rapidly across health care specialties, and oncology is no exception.
Researchers have developed AI tools to assist in detection and diagnosis of several cancers, such as those of the breast, colon, liver, lung and prostate.
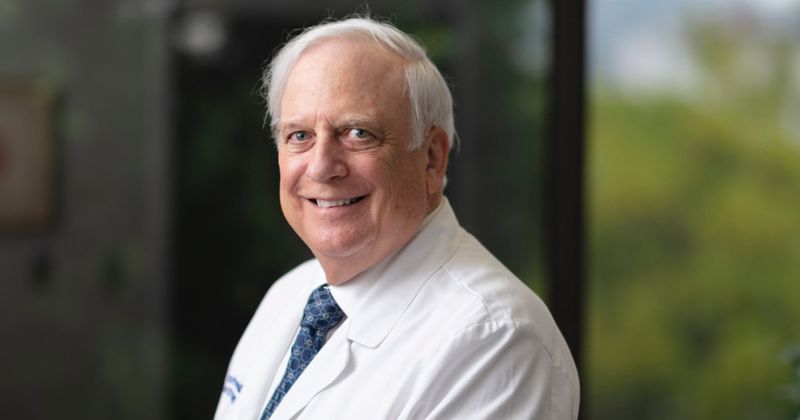
Advances in image acquisition devices, processing techniques and analysis tools also have opened the door for medical imaging-based AI screening in one of the most lethal and notoriously difficult-to-detect malignancies — pancreatic cancer.
“Pancreatic cancer is projected to become the second-leading cause of cancer mortality in the U.S. by 2030,” Stephen J. Pandol, MD, gastroenterologist at Cedars-Sinai Medical Center, told Healio. “Unlike for other cancer types, such as breast cancer and prostate cancer, we are not making progress against pancreatic cancer. This is why being able to identify novel ways to diagnose pancreatic cancer in its earliest stage is at the top of the list of things that we need to solve.”
Machine learning methods have shown the potential to identify individuals at high risk for pancreatic cancer and allow for earlier diagnosis of a disease with no specific symptoms and diagnostic biomarkers.
“Patients may experience abdominal pain, but many things can cause abdominal pain and it is difficult for clinicians to put things together and identify the pancreatic cancer early,” he said. “Pancreatic cancer is most diagnosed in late stage, when a CT scan eventually reveals the disease, so we need to figure out how to change this story and improve upon early diagnosis. We know from previous experience with other cancer types that the earlier the diagnosis, the better the outcome. If we can identify patients early enough, they can potentially undergo surgery and live longer, which is our ultimate goal.”
Healio spoke with gastroenterologists, radiologists and other experts about the development and increasing use of AI tools for early detection of pancreatic cancer, ongoing research in this space, and the role AI could eventually play in pancreatic cancer screening.
AI analysis of CT scans
One way AI has been deployed in pancreatic cancer is to identify subtle signs of the disease that are undetectable to the human eye.
A study by Pandol and colleagues showed an AI tool trained to analyze prediagnostic CT scans could predict with high accuracy which individuals would develop pancreatic cancer.
Pandol is co-leader of the national Chronic Pancreatitis, Diabetes and Pancreatic Cancer Consortium, sponsored by NCI and National Institute of Diabetes and Digestive and Kidney Diseases.
“The consortium includes several U.S. medical centers and involves chronic pancreatitis, diabetes and pancreatic cancer because they are all interactive,” he said. “The consortium was created to monitor large groups of patients with these three diseases to better understand the natural history of disease and develop better methods for diagnosis and treatment.”
As part of the consortium, researchers conducted a multisite study to determine whether pancreatic cancer could be diagnosed at early stages through AI analysis of CT scans.
“We know that even a CT scan can miss pancreatic cancer in its earliest stages,” Pandol said. “Radiologists may not be able to see the cancer either because of the way that images are presented, or the cancer is so small that they can’t see it. We, therefore, wondered whether there was a possible way to assist radiologists in pancreatic cancer screening with AI techniques.”
For the study, published in Cancer Biomarkers, researchers obtained a set of 108 retrospective CT scans from 72 individuals. The investigators identified those at high risk for early-stage pancreatic ductal adenocarcinoma from biomarkers identified in prediagnostic abdominal CT scans. They developed the AI model using 66 multiphase CT scans as the internal data set and 42 venous-phase CT scans for external validation.
Results showed the model had an average classification accuracy of 86% and distinguished textural variations on the pancreas of patients vs. healthy controls that may be due to molecular changes during cancer development.
“We were able to take a small group of patients and show with good sensitivity and specificity that we could identify a pancreatic cancer signal in prediagnostic CT scans for most patients who were later diagnosed with pancreatic cancer via CT scan,” Pandol said. “NCI was extremely excited about this and gave us a large research grant to further our work. We have now brought in multiple centers across the U.S. to collect CT scans and analyze the data. We plan to have 1,000 scans and are well on our way. We are doing a lot to improve AI so that we can have machine learning identify the pancreas without a human having to trace it and then pick out the biomarkers for pancreatic cancer diagnosis.”
Pandol said the hope is to eventually apply the AI tool prospectively to determine whether a patient at a clinic or hospital is at risk for pancreatic cancer.
“This will ultimately lead to management of those patients and even preventive therapy in the coming years,” he continued. “The overall results of our research are anticipated in a couple of years, at which time we hope to have a revised, advanced AI model.”
AI-based algorithm
Another AI-based model trained with electronic medical records from the Danish National Patient Registry also showed potential for pancreatic cancer prediction, identifying individuals with a 25-fold greater risk for developing the disease within 3 to 36 months.
Bo Yuan, a PhD candidate at Harvard University, presented the findings during this year’s American Association for Cancer Research Annual Meeting.
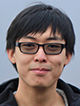
“The purpose of this study was to develop an artificial intelligence tool that can help clinicians identify people at high risk for pancreatic cancer so they can be enrolled in prevention or surveillance programs and hopefully benefit from early treatment,” Yuan said in an AACR press release.
A range of machine-learning methods underwent testing, from regression to time-series methods. Researchers specifically trained the models on the sequence of diseases in each patient’s clinical history so the models could learn diagnosis patterns most predictive of pancreatic cancer risk.
“We use the analogy that a sequence of medical records is similar to a sentence of English words,” Yuan told Healio earlier this year. “We all know that there have been many impressive AI models for natural language processing problems, such as translation, text to voice and text analysis. Why can’t we adapt such concept to a biomedical task?”
Results showed the best model substantially outperformed a model without time information (AUC = 0.88; OR = 47.5 for 20% recall, 159 for 10% recall), even when training did not include disease events that occurred 3 months before diagnosis (AUC = 0.84).
Researchers further validated the results using EMRs from Mass General Brigham Health Care System and reported similar accuracy with the model.
“If we can find a way to identify patients with cancer earlier than their usual diagnosis time, it could potentially be very beneficial for the patients and the general public,” Yuan said. “AI models have been shown to be powerful and successful in many real-world applications. We, therefore, argue the application of machine-learning methods to massive hospital data sets might be a way to design such predictive tools.”
However, applying the methods in a diverse health care system presented unexpected challenges, Yuan said.
“Blind transfer of a model trained in one country to another was not successful. But performance of models independently trained in each country, fortunately, had similarly high levels of performance, despite substantial differences in health care economics and billing and recording practices,” Yuan said. “This underscores the robustness of deep machine-learning methods on complex, large data sets.”
Researchers also had difficulty identifying precisely which diagnosis patterns predicted pancreatic cancer risk because of the complex nature of the neural network, but they found significant associations of certain clinical characteristics — including diabetes, pancreatic and biliary tract diseases, and gastric ulcers — with increased risk.
“In more general terms, these results indicate the strong potential of advanced computational technologies such as AI and deep learning in contributing to solutions to real-world health problems by making increasingly accurate predictions based on each individual’s health and disease history,” Yuan said.
Future steps toward implementation of early pancreatic cancer diagnosis and treatment in clinical practice should include detailed screening of high-risk patients and effective treatment after early detection, he added.
“With a reasonably accurate method for predicting cancer risk, one can direct appropriate high-risk patients into clinical screening trials,” Yuan said. “A sufficiently enriched pool of high-risk patients would make detailed screening tests more affordable — as such tests are likely to be prohibitively expensive at a population level — and enhance the positive predictive value of such tests.”
Other ongoing research
A novel approach for early cancer detection that utilized a machine learning algorithm identified more than 95% of early-stage pancreatic cancers among a cohort of patients at Moores Cancer Center at UC San Diego Health.
Researchers on the case-control pilot study, published in Nature Communications Medicine, assessed the use of high-concordance di-electrophoresis to detect extracellular vesicles and AI-enabled protein marker analysis to predict the likelihood for future malignancy of stage I to stage II pancreatic, ovarian and bladder cancers.
Researchers compared 139 pathologically confirmed cancer cases with 184 controls. The AI approach yielded an AUC of 0.95 (95% CI, 0.92-0.97), with a sensitivity of 71.2% (95% CI, 63.2-78.1) and specificity of 99.5% (95% CI, 97-99.9). Results also showed similar sensitivity for both stage I (70.5%; 95% CI, 60.2-79) and stage II (72.5%; 59.1-82.9) disease.
“The pancreatic cancer result is particularly promising,” Scott M. Lippman, MD, director of Moores Cancer Center and principal investigator of the Stand Up To Cancer–Lustgarten Foundation Pancreatic Cancer Interception Dream Team, said in a press release. “These results are five times more accurate in detecting early-stage cancer than current liquid biopsy multicancer detection tests.”
Lippman said if the results are validated, “we can greatly reduce mortality from this disease, which will soon become the second-leading cause of cancer mortality in the U.S.”
Despite the promising study results, AI tools to diagnose pancreatic cancer at earlier stages have yet to advance from the lab to the clinic, Ismail B. Turkbey, MD, senior clinician in the molecular imaging branch at NCI and NIH, told Healio.
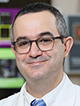
“We need to see that extension for us to see a big change in the practice of medicine,” Turkbey said. “This will require larger-scale studies, a larger scale of AI training and prospective randomized use in well-designed studies.”
Among the organizations pursuing such research is the Society of Abdominal Radiology.
“The society’s pancreas focus panels have a large research group examining chronic pancreatitis, which is a known precursor of pancreatic cancer,” Turkbey said.
Looking ahead
Experts with whom Healio spoke said next steps for research will involve making AI tools for pancreatic cancer detection even smarter.
In addition to identifying biomarkers not easily seen by a radiologist on CT scans, Pandol and colleagues are testing an AI-predicted algorithm of patient records, including medications that patients have received, and within the next 5 years hope to implement the algorithm across testing medical centers and identify patients with early-stage pancreatic cancer.
“This will be our next milestone,” Pandol said. “Once we do that, we will be able to take those patients who are at high risk and consider different types of therapy to prevent the cancer from happening in the first place or prevent disease progression. We expect this to happen within the next 7 to 10 years.”
Physicians should not be concerned that AI will take over their practice or replace them, Pandol said.
“The younger generation of clinicians are very excited about AI in medicine, and because they have grown up with some of the newer technologies, it has been a part of their world for most of their lives,” he said. “We are now at a place where we have potential for new AI methods for earlier diagnosis of pancreatic cancer that will lead to increasingly better outcomes for our patients.”
Turkbey agreed and said AI will only benefit clinicians who are able and willing to apply it in their practice.
“Physician workload is increasing continuously, including the amount of data needed to review,” he said. “For example, about 5 to 10 years ago, we were responsible for looking at maybe 200 images of the chest, abdomen and pelvis via CT scan. Now, with the increased technologies available, we are asked to look at close to 2,000 images. The good news is that AI is here to make these tasks more efficient. For the 1,000 or 1,500 image sets for patients, a radiologist can literally spend 30 to 45 minutes reviewing them, but a well-trained computer AI algorithm can get this task done in less than 1 minute and this is where AI is chiming in, to mainly cover some of these routine tasks for physicians.”
Turkbey has heard concern from certain physicians about whether they will lose their job to AI — but they won’t, he said.
“Those who are able to adapt AI to their work and learn how to properly interact with AI tools will have an easier medical practice — AI will make their life much easier,” he said. “For any individual who wants to be on the development side of AI, that’s wonderful, and for those who want to be on the user side of AI, that is also really great because they will be able to spend more time with patients. But if they ignore AI, then they may bump into problems. Either way, they will not lose their jobs to the technology. AI will certainly make the field of medicine so much easier.”
References:
- An AI model may predict elevated pancreatic cancer risk using electronic health records (press release). Available at: www.aacr.org/about-the-aacr/newsroom/news-releases/an-ai-model-may-predict-elevated-pancreatic-cancer-risk-using-electronic-health-records/. Published April 8, 2022. Accessed Nov. 15, 2022.
- Hinestrosa JP, et al. Commun Med (Lond). 2022;doi:10.1038/s43856-022-00088-6.
- New screening tool IDs 95 percent of stage 1 pancreatic cancer (press release). Available at: health.ucsd.edu/news/releases/Pages/2022-03-21-new-screening-tool-ids-95-percent-of-stage-one-pancreatic-cancer.aspx. Published March 21, 2022. Accessed Nov. 16, 2022.
- Placido D, et al. Abstract LB550. Presented at: American Association for Cancer Research Annual Meeting; April 8-13, 2022; New Orleans.
- Qureshi TA, et al. Cancer Biomark. 2022;doi:10.3233/CBM-210273.
For more information:
Stephen J. Pandol, MD, can be reached at stephen.pandol@cshs.org.
Ismail B. Turkbey, MD, can be reached at ismail.turkbey@nih.gov.
Bo Yuan, PhD candidate, can be reached at bo_yuan@hsph.harvard.edu.