Machine-learning approach using step counts predicts hospitalization during radiotherapy
An artificial intelligence model appeared to predict the likelihood of unplanned hospitalizations during chemoradiation therapy among a cohort of patients with various cancer types.
The results, presented at American Society for Radiation Oncology Annual Meeting, showed the model, which used daily step counts measured through wearable devices as a proxy to monitor patients’ health, provided physicians with a real-time method to provide personalized care.
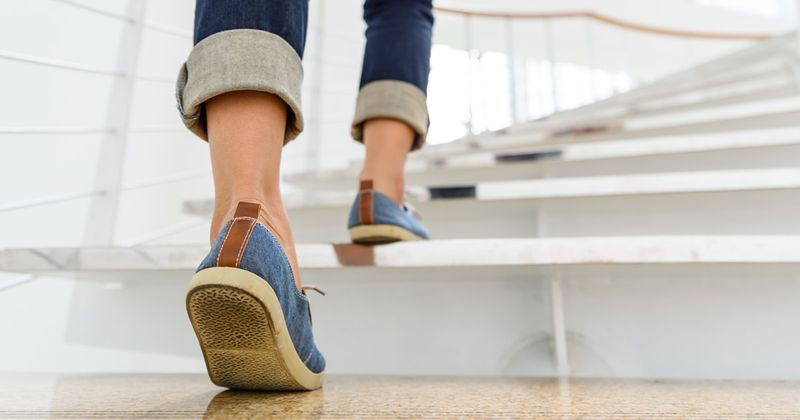
Background and methodology
About 10% to 20% of patients who undergo outpatient radiation or chemoradiation require acute care via an ED visit or hospital admission during their course of treatment. These unplanned hospitalizations can cause treatment delays and stress that may affect clinical outcomes, according to a press release.
“Wearable devices allow for continuous, objective capture of patient-generated health data outside of the clinical setting, which minimizes travel and has the potential to have a more realistic and equitable assessment of a person’s health status,” Isabel Friesner, clinical data researcher at University of California, San Francisco, said during the presentation. “While wearable device data have often been used to encourage patients to increase their physical activity, this study is unique in that we are building machine-learning models based upon step count data to identify potential clinical outcomes — namely hospitalizations.”
Friesner and colleagues used data from three prospective, single-institution trials of activity monitoring for patients undergoing chemoradiation. Each patient received a fitness tracker and wore it before and during chemoradiation treatment.
“Previous work regarding these trials showed a significant association between recent step counts and the risk for hospitalization, where a reduction in risk was associated with an increase in daily number of steps,” Friesner said.
Researchers used the data to develop and internally validate three machine-learning approaches based on daily step counts during chemoradiation treatment to predict hospitalization rates, including elastic net-regularized logistic regression, ensembled sparse-input neural networks and random forest.
The study included 214 patients (median age, 61 years) with 11 primary cancer sites, including head and neck (30%) and lung (29%). Researchers grouped 70% of patients into a training set (n = 151) and the remaining 30% into a hold-out test set (n = 63). For each patient, researchers analyzed recorded data from the fitness tracker starting 1 week before chemoradiation until either a first hospitalization event or the end of treatment.
Key findings
Researchers reported an overall first hospitalization event rate of approximately 27%.
Results of the three machine-learning approaches showed the elastic net approach demonstrated the best overall performance, with an area under the curve (AUC) of 0.8, followed by the neural net approach with an AUC of 0.72 and random forest with an AUC of 0.65.
“We then took the best-performing model, the elastic net, and wanted to see how important the step count features were to the model’s performance,” Friesner said. “We created a model with only step counts and one with only clinical features/without step counts.”
Results showed the model that included step counts significantly outperformed the model without step counts (AUC, 0.8 vs. 0.46).
Limitations of the study included the small data set of only 214 patients, Friesner said.
Future research
“These data highlight the importance of step counts to the predictive ability of a machine-learning model,” Friesner said. “Machine learning can be applied to daily activity monitoring data to predict hospitalization.”
The researchers plan to conduct more rigorous validation of the algorithm on the NRGF-001 trial, which will randomly assign patients undergoing chemoradiation therapy for lung cancer to treatment with or without daily step count monitoring. Additional studies are also planned to examine additional metrics collected by wearable devices, such as heart rate, and their utility in the clinic.
References:
- AI model using daily step counts predicts unplanned hospitalizations during cancer therapy (press release). Available at: www.astro.org/News-and-Publications/News-and-Media-Center/News-Releases/2022/AI-model-using-daily-step-counts-predicts-unplanne. Published Oct. 24, 2022. Accessed Oct. 24, 2022.