Model predicts deterioration of hospitalized patients with cancer
Hospital admission often is a crucial step for stabilizing or treating a person with cancer.
However, it is not without risk. Approximately 9% of hospitalized individuals with cancer experience complications that cause their condition to deteriorate, require a transfer to the ICU or lead to death.
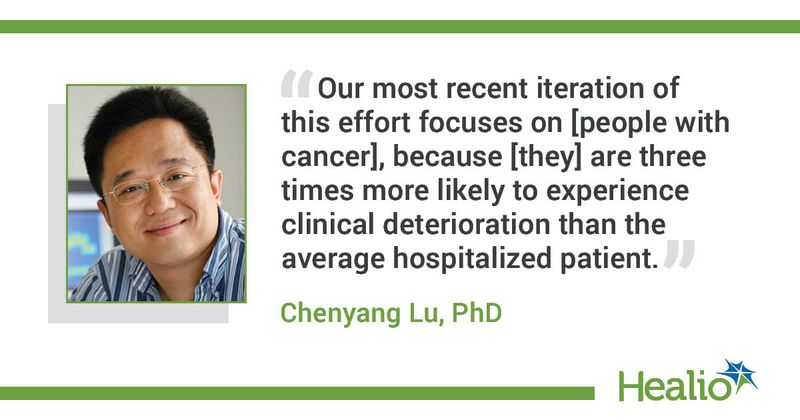
Researchers at Washington University in St. Louis are developing a machine learning-based early warning system model to identity patients at particular risk for deterioration.
“We’ve been working on this type of predictive model for early warning systems in hospitals for a long time,” Chenyang Lu, PhD, Fullgraf professor at Washington University’s McKelvey School of Engineering, told Healio. “Our most recent iteration of this effort focuses on [people with cancer], because [they] are three times more likely to experience clinical deterioration than the average hospitalized patient.”
Lu spoke with Healio about the system’s potential, how it may help improve outcomes and the possible challenges to its implementation.
Healio: How did you develop this system?
Lu: I specialize in artificial intelligence and machine learning, which is getting a lot of attention. It does everything from language translation to voice recognition to image classification. The most powerful type of machine learning models are deep learning models. To improve predictive performance, these deep neural networks use two types of data in electronic health records. Static variables are usually collected at the time of hospital admission, and time series data — such as vital signs — are captured repeatedly during hospitalization.
We used de-identified data from more than 20,000 hospitalizations of people with cancer at Barnes-Jewish Hospital, and we found a way to integrate static and time series data in one unifying model that continually takes new data and generates new predictions.
The static data help the model get the context right, and the time series data provides updated, dynamic information about the patient as the hospital stay goes on. We call this multimodal fusion. You use the information you learned from the static data and the correlation you learned between the static and the time series data, and then you can fill in the gaps to make better predictions.
Healio: You conducted a case study to see assess how the model performed in terms of ‘alarm fatigue’. How did it perform?
Lu: Early warning systems utilize patient data to determine that the patient will deteriorate. This prompts an alarm that calls providers to the patient’s bedside.
One problem with this is alarm fatigue, and this is a huge issue. We know nurses and care staff are very busy with the regular care protocol. If a provider starts getting too many false alarms, they may start to ignore those alarms. We want to prevent alarm fatigue, so we performed a simulation in which we controlled the number of alarms per hour in the oncology ward. With any machine-learning model, there is a tunable parameter — a threshold that essentially is a risk probability. You must cross that threshold for an alert to be issued. We controlled that threshold so it would never generate more alerts than that threshold allowed. We set a threshold of 48 notifications in a 24-hour period, or one every 30 minutes. We then implemented a more proactive early warning system in which the alarm rate could be high, but with limits on false alarms to avoid alarm fatigue. With the same rate of false alarms, the model captured 39.5% of clinical deterioration events. An existing model used by many hospitals only captured 3.9% of those events.
Healio: What is the next step in developing this system?
Lu: The next big thing is how to take advantage of these predictions through interventions that can change the outcome. We want to assess is what is referred to as a ‘human-in-the-loop’ artificial intelligence. In plain terms, this looks at how the clinicians and nurses work with AI to develop better interventions. What would enable them to take better advantage of it?
Another issue is that providers need to know when the predicted deterioration is going to happen. They might get an alert, but they don’t know if this is going to happen in the next hour, or tomorrow, or the day after tomorrow. We developed a new machine-learning model in 2020 that does two additional things. One is to associate a time horizon with the alert. When the provider gets an alert, it will tell them this is a 6-hour alert — meaning it will happen in the next 6 hours — or it might give a 48-hour alert. If it’s a 6-hour alert, the situation might require some urgent action. If it’s 48 hours, it might simply mean more careful observation. They can develop their intervention plan accordingly.
References:
Li D, et al. Integrating static and time-series data in deep recurrent models for oncology early warning systems. Presented at: Proceedings of the 30th ACM International Conference on Information & Knowledge Management (virtual meeting); Nov. 1-5, 2021.
Li D, et al. DeepAlerts: Deep learning based multi-horizon alerts for clinical deterioration on oncology hospital wards. Presented at: Proceedings of the AAAI Conference on Artificial Intelligence, Feb. 7-12, 2020; New York.
For more information:
Chenyang Lu, PhD, can be reached at McKelvey School of Engineering, NSC:1100-122-303, 1 Brookings Drive, St. Louis, MO 63130-48; email: lu@wustl.edu.