AI an emerging tool, not substitute, for oncologists
Advances in artificial intelligence technology and deep learning algorithms are leading the way to more timely and accurate cancer diagnoses, with the potential to improve patient outcomes.
Artificial intelligence (AI) techniques can be used to help clinicians diagnose patients with a variety of cancer types by recognizing biomarkers that may be difficult to identify on scans and tests.
“We are seeing AI take off and pass human performance in a large number of tasks,” Rodney LaLonde, PhD candidate in computer science at the Center for Research in Computer Vision at University of Central Florida, told HemOnc Today. “I’m at an internship right now for self-driving cars, and we are using the same types of methodologies to detect cancer as we are for these cars to detect pedestrians crossing the street. It’s very exciting to see the flexibility of these algorithms.”
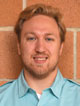
There is a growing consensus, however, that these machines will not take the place of oncologists. Although AI is useful in processing large amounts of data and making predictions based on statistics, it lacks the ability to reason and think beyond the numbers when dealing with patients. Experts say this human component is crucial to diagnosing and treating patients with cancer.
“There needs to be a symbiotic relationship between machine and man when it comes to AI. It does not have general intelligence, it just does what you tell it to do,” Ulas Bagci, PhD, professor of AI in medicine at University of Central Florida, told HemOnc Today. “Yes, AI can learn, and yes, it is very efficient, but it is complementary to humans so that they can help each other to solve problems more accurately and efficiently.”
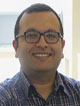
HemOnc Today spoke with experts about the evolving role of AI to help detect cancer, how AI may be used to prevent unnecessary procedures by distinguishing between benign and malignant lesions, and where AI’s future lies in the field of oncology.
An AI ‘amenable’ malignancy
In 2018, researchers demonstrated for the first time that a form of AI, a deep learning algorithm called convolutional neural network (CNN), is more effective than experienced dermatologists for melanoma detection.
Studies have shown an average sensitivity for detecting melanoma of less than 80% among dermatologists trained in dermoscopic algorithms. To improve upon that sensitivity, researchers have evaluated automated computer image analysis — which incorporates human-entered dermoscopic segmentation criteria — to help physicians screening for melanoma.
Taking that approach a step further, deep learning CNNs seek to mimic the biological processes that occur when neurons in the brain respond to what the eyes see. These networks can perform image analysis and teach themselves to improve performance through machine learning.
In a comparative cross-sectional study published in Annals of Oncology, investigators evaluated a deep learning CNN for the diagnostic classification of dermoscopic images of melanocytic lesions, which they then compared with the diagnostic ability of 58 dermatologists.
The analysis included a 100-image test set divided into two levels. Level 1 included dermoscopy only, whereas level 2 included dermoscopy plus clinical information and images.
Results showed a mean sensitivity of 86.6% (standard deviation [SD], ± 9.3%) and mean specificity of 71.3% (SD, ± 11.2%) for lesion classification among the dermatologists in level 1. With the addition of clinical information in level 2, sensitivity increased to 88.9% (SD, ± 9.6%) and specificity to 75.7% (SD, ± 11.7%).
The CNN receiver operating characteristics area under the curve showed an 82.5% specificity, which was significantly higher than that of the dermatologists in both levels 1 and 2 at their corresponding sensitivities (P < .01 for both).
“Dermatology is certainly much more amenable to this technology because the first step of diagnosis is based on looking at the lesion, which is different than something like breast cancer that requires internal imaging,” Roger Ho, MD, MPH, assistant professor and director of resident education in the Ronald O. Perelman department of dermatology at New York University, told HemOnc Today. “This is still developing in the field of dermatology, and making medical diagnoses with AI is still in its primitive stage. The holy grail is having the machines themselves making diagnoses based on images of the lesions, but right now we just don’t have the repertoire of images to train these systems to come up with a near-perfect algorithm that can make accurate predictions every time.”
Training AI systems involves uploading hundreds of thousands of photos of lesions that have been confirmed as benign or malignant. These are used to teach the technology to recognize skin cancer. Apps such as SkinVision can check smartphone photos of suspicious lesions and give users a low- or high-risk indication within 30 seconds.
Although this technology may lead to a quicker diagnosis, it can’t match physicians’ ability to distinguish variations among individuals.
For instance, people have different skin types and colors, and different lesions on different parts of the body don’t always match, Ho said.
“I don’t think it’s possible to get to 100% accuracy with AI recognizing specific lesions,” Ho said. “The goal is to generate a system that can make reasonable predications on a lesion that can facilitate and triage different medical resources to the right people.
“If there is a lesion with a higher chance of being a malignancy, that person should have a higher priority of seeing a dermatologist vs. another person who has a lesion that is less likely to be a malignancy,” he added. “The best case scenario is to get to a point when we can triage patients based on submitting these pictures.”
Preventing unnecessary procedures
A top priority for the use of AI in cancer diagnosis is to accurately distinguish between benign and malignant lesions.
Such an ability could reduce the number of unnecessary procedures performed on patients, improving their quality of life and lowering overall health care costs.
A team of researchers from Massachusetts General Hospital and Massachusetts Institute of Technology sought to develop a machine learning model for breast cancer that could predict the probability of a high-risk breast lesion developing into cancer.
About 14% of image-guided biopsies performed following suspicious mammograms result in the discovery of high-risk breast lesions. Most high-risk breast lesions are benign, but surgery is usually performed because of the risk for an upgrade.
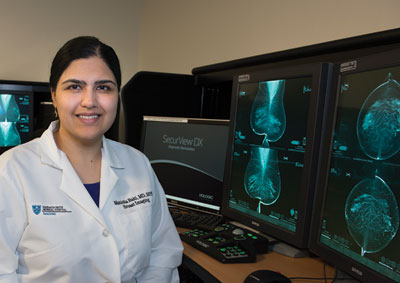
Source: Laurie Lizotte© Massachusetts General Hospital.
However, use of the machine learning model is projected to decrease unnecessary surgery by 30% and could help physicians and patients decide whether to remove the lesion or move forward with surveillance.
“The status quo has been overtreatment with unnecessary surgery for high-risk lesions that aren’t associated with cancer,” Manisha Bahl, MD, MPH, assistant professor at Harvard Medical School and radiologist at Massachusetts General Hospital, told HemOnc Today. “Given this clinical scenario, we decided to apply machine learning to better risk-stratify patients. Multiple studies have investigated patient features and imaging features to better stratify patients, but currently there are no features that allow us to distinguish high-risk lesions that need surgery from those that can be safely followed. This has led to a wide variation of treatment.”
In one study, Bahl and colleagues analyzed 1,006 high-risk lesions and used 66% (n = 671) of them to train the machine learning model with mammogram and biopsy reports as well as patient age and risk factors. They tested the resulting model on the other 33% (n = 335) of the high-risk lesions.
Results showed that the model was able to detect 97.4% (37 of 38) of cancers that would have been diagnosed at surgery. Overall, the model allowed researchers to reduce the number of benign-lesion surgeries by 30%.
“This is very important for the patient’s quality of life,” Bahl said. “There is a growing trend in breast cancer care toward less invasive treatment. Our model could be used to inform decision-making regarding surveillance vs. surgery of high-risk lesions and therefore could support more personalized approaches to patient care.”
Another potential application for such a model is the management of ductal carcinoma in situ (DCIS). Some argue standard-of-care surgery with radiation is overtreatment for a noninvasive cancer that may or may not progress to invasive cancer in a woman’s lifetime.
In addition to several active surveillance trials underway, Bahl and colleagues are developing an AI model to better risk-stratify patients with DCIS.
“One of the strengths of machine learning vs. traditional logistic regression is that it can process and synthesize huge amounts of data,” Bahl said. “For this particular model on high-risk breast lesions that we developed, we inputted more than 20,000 overall different data points, which a machine can process but a human can’t.”
The machine takes into account relationships among risk factors, age, and mammogram and pathology reports and computes a score of whether surgery should be conducted.
A score of more than 5% predicted the need for surgical excision, whereas a score of less than 5% indicated surveillance would be appropriate, Bahl said.
A limitation, however, is that the model is based solely on the initial time when the high-risk lesion is diagnosed.
Bahl said researchers are discussing how to best monitor the patients who do not have surgery.
“Now that we are not excising all high-risk lesions, we are working to develop appropriate protocols for monitoring patients,” Bahl said. “For someone who did not have a high-risk lesion excised, we generally recommend follow-up imaging in 6 months. At that point, we re-assess the finding that led to the biopsy and high-risk lesion diagnosis. If it’s stable or resolved, then continued surveillance is appropriate. However, if the finding has increased in size or is more suspicious in any way, then we would likely recommend surgery.”
AI also may help with breast cancer diagnosis on mammograms.
In a study published last month in Radiology: Artificial Intelligence, researchers trained an AI system to learn a large number of digital breast tomosynthesis (DBT) data sets and report suspicious findings from the images.
Researchers enrolled 24 radiologists and 13 breast subspecialists to each read 260 DBT exams (65 total cancer cases) with and without the help of AI.
Results showed that AI improved accuracy and reduced the time it takes to read DBT exams. Sensitivity increased from 77% without AI to 85% with AI, specificity increased from 62.7% to 69.6%, and the recall rate for noncancers decreased from 38% to 30.9%.
The time it took to read DBT exams decreased from 64 seconds without AI to 30.9 seconds with AI.
“We know that DBT imaging increases cancer detection and lowers recall rate when added to 2D mammography, and even further improvement in these key metrics is clinically very important,” study author Emily F. Conant, MD, professor and chief of breast imaging from the department of radiology at Perelman School of Medicine at University of Pennsylvania, said in a press release. “And, since adding DBT to the 2D mammogram approximately doubles radiologist reading time, the concurrent use of AI with DBT increases cancer detection and may bring reading times back to about the time it takes to read digital mammogram-alone exams. The results of this study suggest that both improved efficiency and accuracy could be achieved in clinical practice using an effective AI system.”
A new frontier for deadly cancers
Researchers also hope AI could lead to earlier detection of cancers that have a high mortality rate when diagnosed at a late stage, such as lung and pancreatic cancers.

For instance, a computer model developed at University of Central Florida has been trained to detect tiny specks of lung cancer in CT scans that may be missed by human eyes.
The model is unique in that it contains a capsule neural network that looks at high-level visual attributes such as sphericity, spiculation and lobulation.
“When our model is making its prediction about whether the nodules are cancerous, it will tell the doctor, ‘I believe it is cancerous because it has this much spiculation and it’s this much lobulated,’” LaLonde said.
The algorithm can determine whether the nodule is malignant through a deep learning architecture that was fed hundreds of thousands of examples explaining which nodules are and are not cancerous.
“We are looking to detect small nodules using deep learning algorithms that radiologists may miss,” Bagci said. “Unlike conventional algorithms, we are using capsules which are less known in the community. It can label, it can tell why a nodule is cancerous or not, and it is superior to the conventional deep learning algorithm.”
Under the current standard, nodules up to 5 to 6 mm do not get tested via biopsy, Bagci said.
This machine model, however, can detect smaller nodules — 2 or 3 mm — and can help quickly determine if they are cancerous.
“We can put this into our system and see if it’s benign or malignant,” Bagci said. “Radiologists can use these to help aid their decisions with patients, especially when it comes to ordering a biopsy. A biopsy can then help make final decisions, and what is great is that if this algorithm assists and a biopsy is required, doctors can then diagnosis lung cancer very early, meaning the patient has over a 95% chance of living because they can undergo a surgical resection.”
The model has demonstrated about 95% accuracy in detecting tiny nodules, whereas human eyes are about 65% accurate.
Bagci and LaLonde said their team at University of Central Florida is working to expand this model to several malignancies beyond lung cancer, including prostate and colorectal cancer.
They also hope the model will open a new frontier in diagnosis and treatment of pancreatic cancer.
Tests for pancreatic cancer have limited accuracy and can be invasive, sometimes leading to pain, false-positive results, adverse reactions to anesthesia and pancreatitis.
The treatment most likely to improve survival is surgery at an early stage, which is associated with median OS of 36 months. However, more than 80% of cases are detected in advanced stages, when it is too late for surgery. The 5-year OS rate for those patients ranges from 2% to 5%, compared with 8.5% overall.
“If we can continue to show which lesions turn into cancer, then we can help society come up with new screening practices,” Bagci said. “When these patients undergo MRI scans using the algorithms, it could help doctors detect which ones are going to become cancer, and it could become a very important technique for screening.”
Training the machine and preparing an algorithm for pancreatic cancer, however, is extremely difficult because of the limited number of examples of precancerous lesions.
“It’s very rare that they are detected in the first place,” LaLonde said. “For breast cancer, lung cancer and colorectal cancer, we have fairly large clinical imaging databases that we can use to help train the algorithms.”
Thus, LaLonde, Bagci and colleagues have explored the idea of applying knowledge from other malignancies to pancreatic scans. An initial study — which will be published and presented at the International Conference on Medical Image Computing and Computer Assisted Intervention in October — showed an 8% improvement in detection and diagnosis over previously published results, LaLonde said.
Just ‘another data point’
Experts with whom HemOnc Today spoke said the use of AI will continue to expand beyond cancer diagnosis into treatment by helping physicians choose the best therapy for their patients.
Bagci and LaLonde said their model is currently being tested in several malignancies to help determine treatment plans based on the stage of the tumor.
“Later-stage tumors are where this system helps doctors the most,” Bagci said. “Our system recommends to doctors how to do radiation or whether surgery is an option. It finds the tumor region segment, and it helps a doctor kill the unhealthy tumor areas while preserving the healthy area. We can also estimate how long the patient is going to live based on the treatment and the tumor area.”
Despite the rapid growth of AI, experts said clinicians should not worry about being replaced by a machine.
Instead, they should be excited to have the extra tools they need to diagnose and treat complex forms of cancer without having to constantly analyze thousands of scans, LaLonde said.
“What has happened is that, with the high volume of scans [physicians] are forced to go through, they themselves are turning into pattern-recognizing machines instead of actually working with patients and discussing treatment planning,” LaLonde said. “If AI can help alleviate a lot of that, we can then get a much better relationship between the AI-specific knowledge and the human general knowledge.”
Bahl said that, ultimately, decisions regarding treatment will still always come from a joint discussion between the patient and the physician.
“We think that this model can provide patients and providers with another data point,” Bahl said. “There’s more comfort with risk assessment tools. ...These tools don’t dictate what a patient should or shouldn’t do; instead, these can help patients make a more informed decision.” – by John DeRosier
Click here to read the , “Will advances in AI lead to more effective screening practices for ovarian cancer?”
References:
Bahl M, et al. Radiology. 2018;doi:10.1148/radiol.2017170549.
Conant EF, et al. Radiology: AI. 2019;doi:10.1148/ryai.2019180096.
Haenssle HA, et al. Ann Oncol. 2018;doi:10.1093/annonc/mdy166.
Khosravan N and Bagci U. S4ND: Single-Shot Single-Scale Lung Nodule Detection. Available at: arxiv.org/pdf/1805.02279.pdf. Accessed on July 26, 2019.
Lamb LR, et al. J Am Coll Surg. 2018;doi:10.1016/j.jamcollsurg.2017.12.049.
For more information:
Ulas Bagci, PhD, can be reached at University of Central Florida, 4328 Scorpius St., HEC221, Orlando, FL 32816; email: bagci@crcv.ucf.edu.
Manisha Bahl, MD, PhD, can be reached at Massachusetts General Hospital, 55 Fruit St., Boston, MA 02114; email: mbahl1@mgh.harvard.edu.
Rodney LaLonde can be reached at University of Central Florida, 4328 Scorpius St., HEC221, Orlando, FL 32816; email: lalonde@Knights.ucf.edu.
Roger Ho, MD, MPH, can be reached at NYU Langone Preston Robert Tisch Center for Men’s Health, 555 Madison Ave., 4th Floor, New York, NY 10022; email: roger.ho@nyulangone.org.
Disclosures: Bagci, Bahl, Ho and LaLonde report no relevant financial disclosures.