Noninvasive radiofrequency sensor with machine learning may accurately measure glucose
Key takeaways:
- A noninvasive sensor may measure glucose values by using dielectric spectroscopy to scan radiofrequencies.
- Larger clinical trials are being planned to assess a wearable, noninvasive CGM.
NEW ORLEANS —A noninvasive device using a radiofrequency sensor may be able to measure glucose levels for people with diabetes in the future, according to a speaker at the AACE Annual Meeting.
In a clinical trial, researchers used a radiofrequency dielectric sensor (Know Labs) to rapidly scan a large range of frequencies using dielectric spectroscopy. Voltage values are detected at each frequency and used to measure real-time glucose levels, according to Dominic Klyve, PhD, professor of mathematics at Central Washington University and Know Labs Data Science & Engineering.
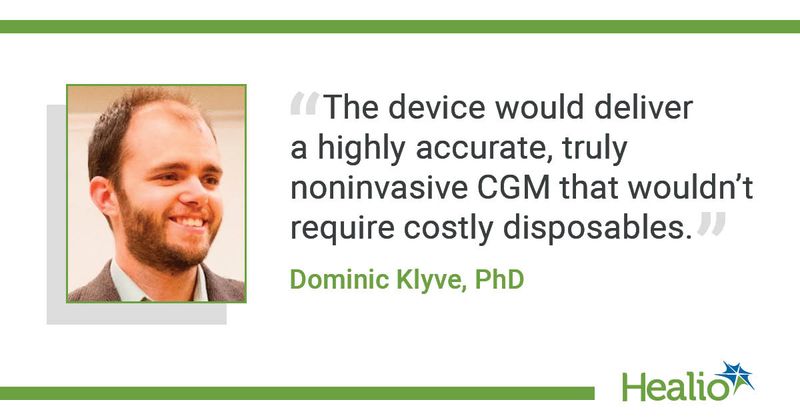
“The importance of continuous glucose monitoring usage in managing diabetes is clearly established,” Klyve told Healio. “Barriers to greater global adoption of CGM are economic costs, discomfort and medical waste. The Know Labs device would deliver a highly accurate, truly noninvasive CGM that wouldn’t require costly disposables.”
Researchers conducted a clinical trial with more than 30 people with prediabetes and type 2 diabetes. The presentation at AACE included data from 10 participants. The radiofrequency sensor continuously scanned participants’ forearms during a 75 g oral glucose tolerance test. Venous blood samples were collected every 5 minutes and paired with radiofrequency sensor scans for data analysis.
There were 520 paired values collected in the study used to train a machine learning model to estimate glucose values from the radiofrequency sensor data. The remaining 130 pairs were used to test the model’s accuracy. Mean Absolute Relative Difference (MARD) was calculated to measure accuracy.
“Machine learning plays a meaningful role in our development of the Know Labs’ noninvasive CGM, accelerating our ability to manage and interpret the large volumes of data we collect from our radiofrequency sensor during clinical studies,” Klyve said. “To date, we’ve employed machine learning to make accurate, real-time blood glucose estimates for study participants that we've collected data on in our lab in Seattle.”
The machine learning model had a MARD of 11.1% compared with venous blood samples. The accuracy for blood samples in the normoglycemic and hyperglycemic ranges were similar. The MARD for glucose samples in the hypoglycemic range with a glucose of less than 70 mg/dL was 9.5%, though only four glucose values were measured within that range.
A surveillance error grid was used to plot the accuracy of measurements by the machine learning model. Of the measurements, 82.3% were placed in risk grade A, the lowest risk grade in the grid, and 17.7% fell into risk grade B. No measurements fell into higher risk grades.
Klyve said the sensor holds promise for the accurate noninvasive measurement of blood glucose and could help reduce the waste, lower cost and make glucose measurements pain- and needle-free. Know Labs is planning large-scale, external clinical studies for a wearable, noninvasive CGM, according to Klyve.
“These new studies will help determine the technology’s performance throughout continuous wear, in more real-world environments and within more extreme glycemic ranges of less than 70 mg/dL and above 350 mg/dL,” Klyve said.