Glycemia Risk Index provides new tool for assessing glycemic control with CGM data
Click Here to Manage Email Alerts
A new metric could help simplify continuous glucose monitoring data for people with diabetes and furnish actionable analysis for providers.

Most providers analyzing CGM tracings for people with diabetes view the ambulatory glucose profile, a report that includes seven metrics: time spent in very low glucose hypoglycemia of less than 54 mg/dL, time in low glucose hypoglycemia between 54 mg/dL and 70 mg/dL, time in target range between 70 mg/dL and 180 mg/dL, time spent in high glucose hyperglycemia between 180 mg/dL and 250 mg/dL, time spent in very high glucose hyperglycemia of greater than 250 mg/dL, coefficient of variation and mean glucose.
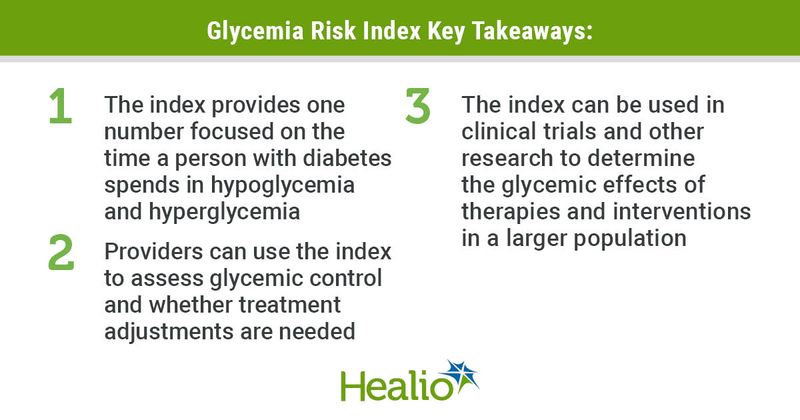
Viewing these reports requires analyzing all seven metrics over a 14-day glucose profile. Additionally, the American Diabetes Association’s Standards of Care state that time in range can be used alone as an assessment for glycemic control, but that metric does not paint a full picture of a person’s glycemic profile, according to David C. Klonoff, MD, medical director of the Diabetes Research Institute at Mills-Peninsula Medical Center in San Mateo, California.
“[Time in range] doesn’t tell you if a patient’s glucose is too high or too low, and it doesn’t tell you if they are very high or just high, and it doesn’t tell you if they’re low or very low,” Klonoff told Healio. “It says nothing about any component of variability; that’s not even built into time in range.”
In an article published in Journal of Diabetes Science and Technology, Klonoff and colleagues unveiled a new metric they call the Glycemia Risk Index. The index incorporates time in very low and low hypoglycemia and very high and high hyperglycemia along with time in range to yield a percentile number from zero to 100. The number gives providers a quick way to assess glucose management, with a low number indicating better glycemic control and a high number indicating worse glycemic control.

“Every patient can benefit from somebody looking at their CGM data,” Anne L. Peters, MD, professor of clinical medicine at Keck School of Medicine of the University of Southern California and an Endocrine Today Editorial Board Member, told Healio. “Glycemia Risk Index makes sense to my primary care colleagues, who want a simple way to interpret CGM data. It could also help when we do remote monitoring research when we want to quickly triage patients who need extra assistance vs. patients who appear to be doing OK.”
Building the Glycemia Risk Index
To develop the Glycemia Risk Index, researchers collected 14-day CGM tracings from 225 adults with diabetes on insulin therapy who participated in four clinical trials. The tracings were reviewed by 330 diabetes experts from six continents who reported reviewing at least 20 CGM tracings per month in their clinical practice. The experts each ranked 15 of the 225 tracings from best to worst in terms of glycemia. In the meantime, principal component analysis was used to identify key elements of variability and other machine learning techniques were used to predict the clinicians’ rankings.
“We looked at the seven metrics and how they varied with one another,” Michael A. Kohn, MD, MPP, professor of epidemiology and biostatistics at the University of California, San Francisco, told Healio. “What became clear was you could combine the seven metrics into two components: one component related to hypoglycemia and one component related to hyperglycemia.”
Researchers implemented machine learning techniques to select the four elements that would make up the Glycemia Risk Index. The elements identified were time in very low glucose hypoglycemia, time in low glucose hypoglycemia, time in high glucose hyperglycemia and time in very high glucose hyperglycemia.
Researchers then used linear regression to weigh the four elements in a way to best match the predictions from the 330 diabetes experts. The highest weight was assigned to time in very low hypoglycemia, followed by time in low hypoglycemia, time in very high hyperglycemia, and time in high hyperglycemia.
The formula for calculating Glycemia Risk Index score is:
Glycemia Risk Index = (3 × % time very low) + (2.4 × % time low) + (1.6 × % time very high) + (0.8 × % time high)
“If the clinician’s rankings are right, then the formula is right,” Kohn said. “We are not predicting objective patient-relevant outcomes, but these are 330 experienced clinicians who are used to reading these CGM tracings. There’s a leap of faith that these clinicians, when they rank these tracings, that represents the risk of patient-relevant outcomes.”
Having a separate hypoglycemia and hyperglycemia component allows providers to take a closer look at what type of adjustments must be made if a patient has a high score on the Glycemia Risk Index.
“The reason we wanted these two dimensions is that they are actionable,” Kohn said. “You want to know whether you need to adjust your treatment to avoid hypoglycemia or adjust your treatment to avoid hyperglycemia.”
Glycemia Risk Index in practice
Klonoff said the Glycemia Risk Index may become a standard method for measuring glycemic control for people with diabetes using CGM and that the index provides a fuller and more accurate picture than existing metrics, such as HbA1c and time in range, meaning that this new composite metric more closely matches clinicians’ opinions as to what constitutes good glycemic control. In their article, the researchers analyzed how the Glycemia Risk Index, compared with time in range, correlated with the clinician rankings. In adjusted analysis, the Glycemia Risk Index was more closely correlated with the rankings than was time in range (adjusted R2 = 0.904 vs. 0.824; P < .00001).
There are several ways the Glycemia Risk Index can be used in both clinical practice and research. In addition to indicating glycemic risk, the index score can also be used to assess a person’s risk for complications, Klonoff said.
The metric can also be used in research for population health analysis. The Glycemia Risk Index can classify people’s control into one of five zones, each containing 20 percentiles. Klonoff said the metric will have utility for trials, clinics and other settings to evaluate how well a large cohort is doing by comparing their distribution into the zones of glycemic control before and after a particular intervention, and this type of comparison will allow for recognizing whether treatments, interventions or other changes have a positive or negative impact on glycemia.
Klonoff said the metric can also be used by algorithm developers to make improvements to automated insulin delivery systems by allowing adjustments to lower a person’s time in hypoglycemia or hyperglycemia based on their Glycemia Risk Index score. If an algorithm improves hypoglycemia but worsens hyperglycemia or vice versa, the outcomes can be modeled to see whether they improve the overall pattern of glycemia according to clinician experts.
“When you have a Glycemia Risk Index, you can plot where patients are and see their scores,” Klonoff said. “If you want a single number that incorporates the effects of the seven major measurements that comprise the ambulatory glucose profile, then this metric can combine them into a score based on how expert clinicians would view the pattern.”
Klonoff added that the metric can easily be incorporated into existing glucose monitoring applications since it adds numbers that current devices already compile. CGM devices could also track a patient’s Glycemia Risk Index over time to follow their glucose trajectory over days and weeks. Klonoff said he has built a Glycemia Risk Index calculator website and will soon build a mobile app for calculating the Glycemia Risk Index from ambulatory glucose profile measurements.
From a clinical perspective, the Glycemia Risk Index can simplify the CGM’s ambulatory glucose profile for providers, especially those who may not have time to view each patient’s profile in detail. Providers can focus on people with diabetes who have higher Glycemia Risk Index scores and look at all the information in their profiles in more detail to see where adjustments can be made.
“If you’re really busy and have a bunch of patients, you’re going to want to triage the ones who need it the most,” Peters said. “For me, it’s simple because I look at CGM tracings all day long, but it’s not as simple if you don’t. The Glycemia Risk Index just makes it easy for people to process what the tracing is showing us.”
Peters said the Glycemia Risk Index is not meant to replace other metrics. It is instead a tool providers can use to simplify how well a patient is doing. It also requires providers to obtain CGM data from patients.
“The providers have to want to use the CGM data, and they have to figure out how to download it,” Peters said. “If they can do that, then we give them a simple metric for triaging patients and figure out which ones need more evaluation.”
The researchers noted a few limitations, including that the CGM tracings came from four clinical trials and may not reflect some clinical populations, the Glycemia Risk Index uses 24-hour data and does not indicate glucose level during specific times of day, and the analysis in the study examined whether the index correlated to clinicians’ rankings rather than clinical outcomes. Future studies examining how useful the metric is to providers and how the metric correlates with clinical outcomes are planned, according to Klonoff.
For more information:
David C. Klonoff, MD, can be reached at dklonoff@diabetestechnology.org.
Michael A. Kohn, MD, MPP, can be reached at michael.kohn@ucsf.edu.
Anne L. Peters, MD, can be reached at annepete@med.usc.edu.