Data predict future rising costs for people with type 2 diabetes
A data-driven approach could improve the way future costs are predicted for people with type 2 diabetes and Medicare, according to a study published in BMC Endocrine Disorders.

“Diabetes is a leading cause of Medicare spending,” Julie C. Lauffenburger, PharmD, PhD, assistant professor of medicine at Brigham and Women’s Hospital and Harvard Medical School, told Healio. “Predicting which individuals are likely to be costly is essential for targeting interventions. Current approaches generally focus on composite measures, short time horizons or patients who are already high utilizers, whose costs may be harder to modify.”
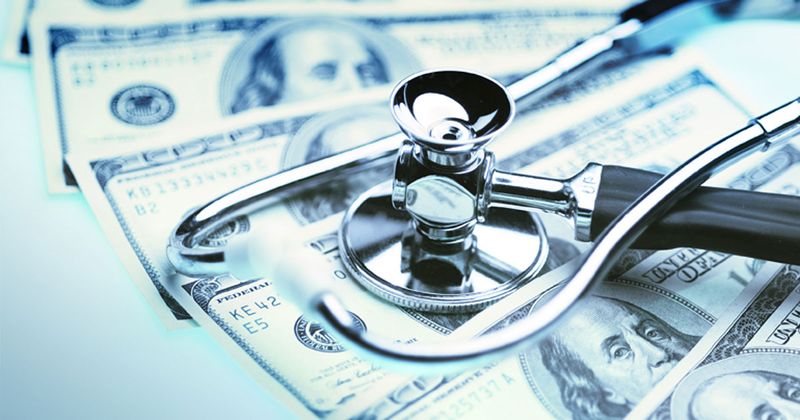
Lauffenburger and colleagues analyzed data from 33,789 Medicare beneficiaries with type 2 diabetes (mean age, 75.9 years; 55.8% women; baseline median spending, $4,153) who maintained continuous eligibility in Medicare Parts A, B or D from 2011 through 2013. The cohort consisted of people who were in the bottom 90% of spending for 2011, which was the baseline year for the study. Each person’s total health care spending was determined by adding together costs from inpatient visits, outpatient medical and physician office visits, and outpatient medications.
Baseline predictors of future spending included sociodemographic and clinical factors. Three models were created, with the first model using all baseline predictors. For the second model, researchers only used diabetes-specific predictors, such as use of insulin and non-insulin injectables, number of oral glucose-lowering agents and use of testing supplies. The third model used only potentially modifiable predictors that could be addressed through intervention or categorization by other research.
Two-year diabetes spending patterns were measured using five different groups. The cohort was divided into a minimal-user group (n = 4,907), low-cost group (n = 8,525), a rising-cost group where costs rose progressively in the first year of follow-up (n = 2,215), a moderate-cost group (n = 9,594) and a high-cost group (n = 8,548).
In the all-baseline predictors model, costs for the minimal-user, low-cost and high-cost groups were accurately predicted. The rising-cost and moderate-cost groups were modestly predicted in this model. In the diabetes-specific predictors model, predictive ability remained modest to strong. In the model using only potentially modifiable predictors, predictive ability was slightly lower, but similar to baseline.
“Patients with type 2 diabetes exhibit dynamic patterns of health care spending, even among those with initially low spending, including those whose costs escalate within the next year (25% of patients) and within the subsequent year (7%) of patients,” Lauffenburger said. “These patients could largely be predicted using basic potentially modifiable factors. These findings could inform the design and timing of interventions, such as medication adherence interventions or interactions that enhance access to care.”
The trajectory model and predicting were also estimated using data from only those in the bottom 40% of spenders (n = 15,017) to further focus on low-cost spending (median spending = $1,255). The results for this group were similar to those for the original cohort, although predictive ability was slightly lower due to the smaller sample size, according to the researchers.
“The ability to potentially discriminate between patients with differing diabetes spending patterns using variables measured at baseline could better target interventions to those who are at greatest need,” Lauffenburger said. “Many health care organizations, insurers, researchers and policymakers make predictions and identify patients for cost-containment interventions using these types of administrative data. Using these longer time horizons could allow for more time to implement potential cost-containment interventions for type 2 diabetes.”
Lauffenburger said a future study should take a deeper look into applying interventions to other populations.
For more information:
Julie C. Lauffenburger, PharmD, PhD, can be reached at jlauffenburger@bwh.harvard.edu; Twitter: @jlauffen.