‘Meal detection’ algorithm could be next step for artificial pancreas
Adults with type 1 diabetes may benefit from a “meal detection” algorithm that has displayed superior accuracy in determining meal size and timing and shows promise as an additional component of artificial pancreas systems, according to researchers.
Samantha Kleinberg, PhD, associate professor of computer science at Stevens Institute of Technology in Hoboken, New Jersey, and colleagues have been working on meal detection since 2015, with their sights set on a “Fitbit for nutrition.” Their most recent work has been studying the ability to identify meal size and timing using an algorithm to aid an artificial pancreas system during the insulin dosing process. The algorithm, which Kleinberg noted also takes physical activity into account, follows the common theme of Kleinberg’s work.
“The motivation is always meal detection, and then this project, we wondered if we could have similar accuracy but in a less intrusive manner,” Kleinberg told Healio. “[We are] trying to identify meals with less burden and fewer devices.”
Working in theory and practice
Kleinberg said the algorithm determines where blood glucose levels are and where they are expected to be, and then when there is a major difference between those values, simulation data help explain the difference.
“Basically, we simulate what’s happening. We put in the real events, and then when the simulation differs from what we’re observing, we test whether a meal might explain that difference,” Kleinberg said. “That’s how it would work in practice. Basically, the simulation is going along in the background and keeps checking whether glucose is different from what is predicted.”
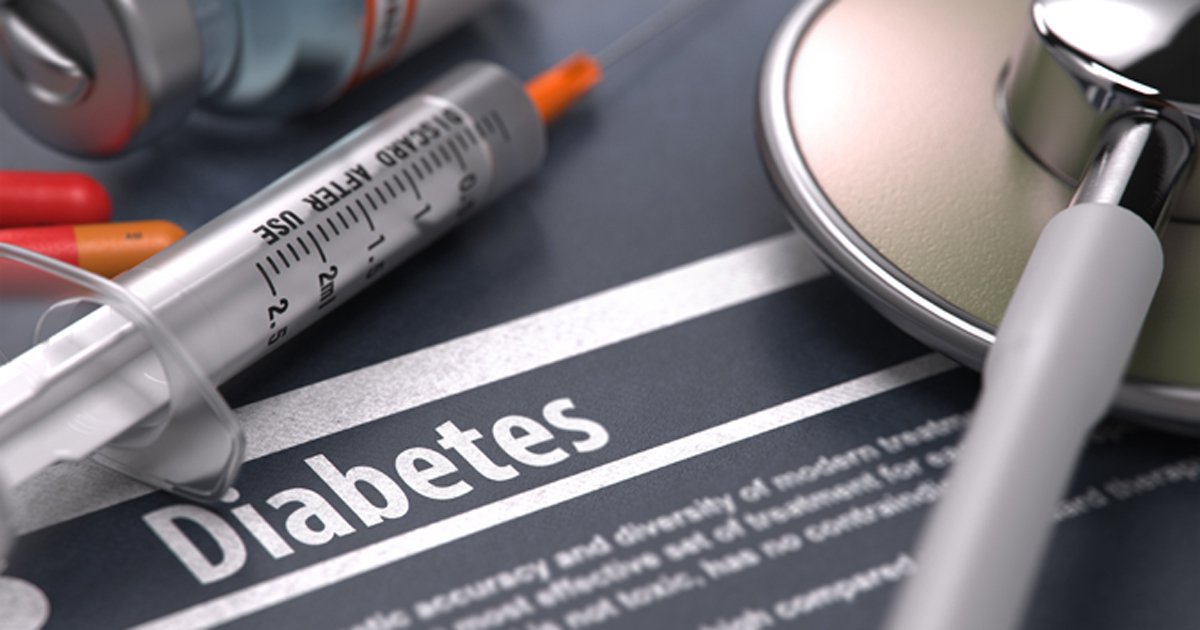
The system has shown that it can work beyond the theoretical as well. Kleinberg and colleagues found that it took a mean of 25.7 minutes for the algorithm to identify when someone ate vs. a mean of 48.3 minutes using variable state dimension, which is another system type used to identify when people are eating. In addition, meal sizes estimated by the algorithm were off by a mean of 1.2 g while meal size from the other system was off by a mean of 17.2 g.
“I didn’t necessarily expect that the meal size error would be so good,” Kleinberg said. “That size error was one of the most important metrics.”
Kleinberg and colleagues went a step further and tested the algorithm in 15 adults with type 1 diabetes from the Diabetes Management Integrated Technology Research Initiative. There was a success rate of 76.7% when it came to finding “plausible” meals using the algorithm compared with a rate of 39.4% using the other system.
“It’s positive in that it shows that it might be possible to improve artificial pancreas systems such that you don’t need meal announcements — people would have a fully closed-loop system,” Kleinberg said. “It’s exciting that this can be done without requiring people to wear even more devices.”
Future plans
Even with such positive results, Kleinberg still envisions a 3- to 5-year trajectory before this type of algorithm could be more widely used. Along the way, further research will need to be done, including larger trials over longer periods of time and analysis among users with type 2 diabetes and gestational diabetes.
Kleinberg noted that the algorithm itself may need fine-tuning, particularly when it comes to personalization, being more specific about macronutrients and taking factors like sleep and stress into account.
“One of the limitations here is a meal is just grams of glucose or carbohydrates. It’s not accounting for protein and fat, which also affect glycemic response,” Kleinberg said. “It’s still not giving you yet a prediction of how much insulin you should give and exactly how you should give it, and I think if we incorporate the food type thing, then we can [do] that a bit better.”
For the algorithm to make sleep, stress and other factors part of the equation, there may be a need to introduce more devices, which seems analogous to what Kleinberg and colleagues are trying to accomplish. However, Kleinberg said, with smartwatches becoming more popular, this potential drawback can be diminished, especially as patients evaluate the benefits.
“For all systems, we think about what’s the tradeoff in accuracy with more sensors vs. fewer. ... A person can make a choice in thinking here’s what accuracy I’m giving up by choosing this subset of sensors,” Kleinberg said. “Personal preference can be quite important.” – by Phil Neuffer
Reference:
Zheng M, et al. J Am Med Inform Assoc. 2019;doi:10.1093/jamia/ocz159.
For more information:
Samantha Kleinberg, PhD, can be reached at samantha.kleinberg@stevens.edu.
Disclosure: Kleinberg reports no relevant financial disclosures.