Machine learning enhances diabetes care, education
HOUSTON — Machine learning has immense potential for improving diabetes care, particularly when used by diabetes care and education specialists, according to two presenters at the American Association of Diabetes Educators annual meeting.
“We are in no way saying, and I in no way believe at all, that machine learning will replace diabetes education,” Mark Heyman, PhD, CDE, vice president of clinical operations of medical technology company One Drop, said during the presentation. “But we really want to understand how it fits within the context of the role of the educator.”
With new technology making it easier than ever to record information about blood glucose levels, physical activity and meals, among other parameters, the amount of personal health information is exploding. Finding easy ways to put these data to use can be a challenge, which is what machine learning aims to address.
“If we can find ways to use that data effectively, we can be a lot more effective as educators,” Heyman said. “The really interesting thing about this, and what really drives machine learning, is the ability to go from an active logging of data to a passive collection of data, which is really powerful.”
From data to action
Dan Goldner, PhD, vice president of data science operations at One Drop, and colleagues are using machine learning to present this wealth of information more effectively and with more impact. There are more than 150 million blood glucose readings from 1.2 million people with diabetes in the One Drop database, Goldner said during the presentation. Machine learning models can pull from this pool of readings and use algorithms to provide personalized blood glucose predictions by incorporating both data from the individual and from the total dataset along with considerations such as time of day and recent activity.
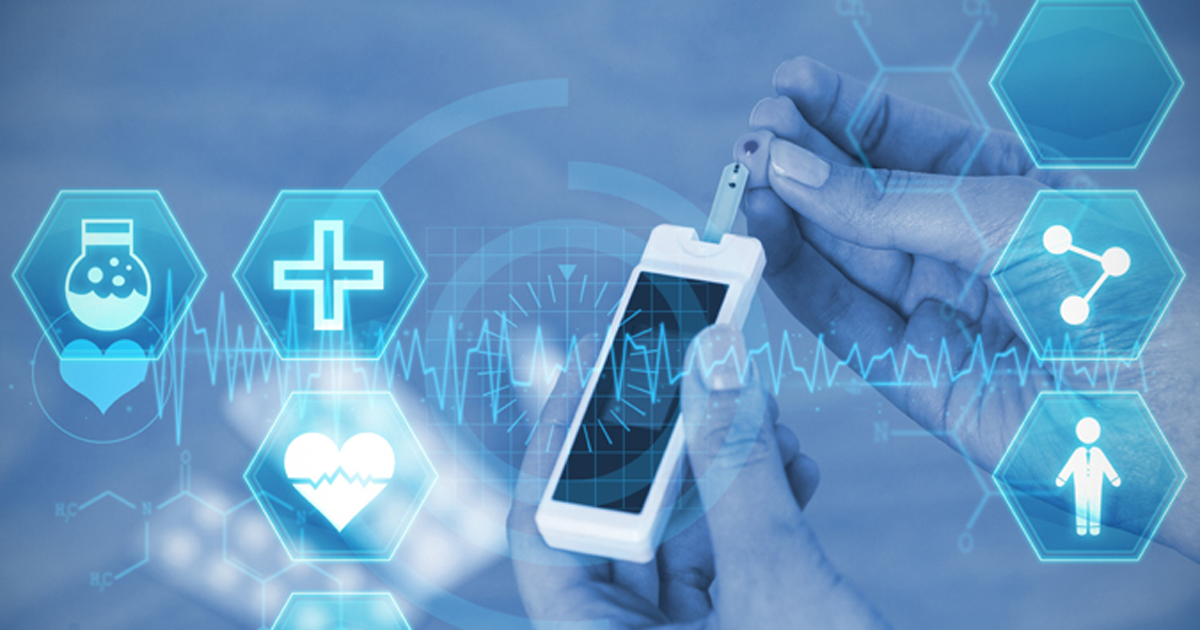
“[We] trained the model to look at 150 million past examples... and all the other data that we have around them and try to understand those patterns well enough that it can then look at the current situation for a current person and say what is that person’s blood glucose likely to do given the situation,” Goldner said.
Goldner said that the system can “make predictions that are pretty good most of the time,” but they are far from static. The system can digest new information throughout the day and adjust its predictions and then interpret inaccuracies and use those to inform future prognostications.
“What we’re actually going for ultimately is for predictions to be wrong,” Goldner said. “We want the prediction that says ‘you’re about to go high’ to end up not coming true because the person took that prediction and did something about it. ... We want the prediction that says you’re about to [go into hypoglycemia] to never come true because as soon as they see it, they know what to do.”
Tool for patients, educators
Heyman called for a “symbiotic” relationship between machine learning technology and diabetes care and education specialists.
“One of my goals as a diabetes educator is to reduce barriers to behavior change,” Heyman said. “I want to help people to change their behavior so they can live healthier and do the things that they want to do in their lives. Machine learning gives diabetes educators the opportunity to do this in a tangible way.”
One Drop has developed a mobile app that incorporates some aspects of diabetes education, providing calls to action along with its recommendations for avoiding predicted blood glucose highs and lows. Ideally, diabetes specialists would use that information — along with their focus on less quantifiable aspects of care, such as motivation, stress and emotional upheaval — to craft personalized diabetes management strategies for patients.
This can encourage patients to take a more active role in their managing their blood glucose.
“To me this is the most important part... it’s going to get me thinking, ‘Wow. What did I just do and what’s going to happen as a result of it?’” Goldner said. “Just going from not wondering to wondering is a very, very important step.”
As technology develops, machine learning can lead to major improvements at both the patient and provider level. For patients, tools for assessing other physiologic factors, as well as automated insulin delivery, are in development. Diabetes specialists will have access to better data and new ideas for diabetes management. They will need to keep up with new offerings to remain an authorities on diabetes technology, Goldner said.
“We’re just at the very, very beginning of this process. Where this is going to go, where machine learning is going to take us, the sky’s the limit,” Heyman said. “As we think about our role as diabetes educators now with machine learning and where to then be going in the future, we need to be flexible and need to be open to understanding this and really working with it as a concept.” – by Phil Neuffer
Reference:
Goldner DR, et al. F15. Presented at: American Association of Diabetes Educators; Aug. 9-12, 2019; Houston.
Disclosures: Goldner reports he is an employee of One Drop. Heyman reports he is an employee of One Drop and a consultant for Eli Lilly and Tandem Diabetes.