Smartwatch app may predict diabetes via heart rate variability
Click Here to Manage Email Alerts
Heart rate sensors in smartwatches utilizing a deep learning app detected diabetes in users with 85% accuracy, according to findings presented at the Association for the Advancement of Artificial Intelligence annual meeting.
“Using consumer-grade heart rate sensors in a medical context presents several challenges,” Brandon Ballinger, co-founder of Cardiogram in San Francisco, and colleagues wrote, adding that sensors typically have significant error and vary the rate of measurement to preserve battery life, and daily activities such as exercise, stress, and alcohol or coffee consumption can confuse device problem-solving techniques.
“Deep neural networks have shown high accuracy at pattern recognition from noisy, complex inputs, including automated detection of diabetic retinopathy from images, skin cancer from mobile phone cameras, and the onset of health conditions from electronic medical records,” the researchers wrote.
Through a partnership with the Heart eHealth Study of the University of California, San Francisco, researchers recruited 14,011 participants with Apple watch heart rate monitors worldwide, collecting 200 million unlabeled sensor measurements. Researchers then used the data in two semi-supervised training procedures. The first semi-supervised sequence training used pretrained, long short-term memory as a sequence autoencoder, making use of a limited pool of labeled data from participants with known diagnoses. For the second procedure, heuristic pretraining, the neural network was pretrained to compute heart rate-derived biomarkers from the medical literature.
Study participants completed a medical history, including previous diagnoses, blood test results and medications. The mobile app, integrated with Apple’s HealthKit, continuously stored, processed and displayed participant heart rate, step count and other activity data. Researchers randomly assigned participants to the training, tuning or testing set, with each participant’s data split into week-long segments, for a total of 57,675 person-weeks of data. Researchers compared deep-learning architecture with multiple machine-learning algorithms, using hand-engineered biomarkers from medical literature, including resting heart rate, time-windowed average heart rate and time-windowed standard deviation of heart rates.
In the procedure utilizing long short-term memory without pretraining, the deep-learning algorithm detected diabetes with 85% accuracy vs. other algorithms, which had accuracy ranging from 31% to 79% accuracy. The algorithm similarly detected sleep apnea with 80% accuracy, as well as hypertension (80% accuracy) and high cholesterol (67% accuracy), according to researchers.
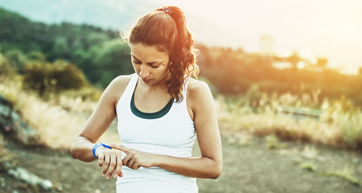
“Previous work from the medical community has suggested that diabetes may impact heart rate variability through effects on the autonomic nervous system,” the researchers wrote. “Our work builds on top of these previous findings, and is the first to show that heart rate variability changes driven by diabetes can be detected via consumer, off-the-shelf wearable heart rate sensors.”
Beyond prediction accuracy, the researchers outlined four key challenges that must be overcome for AI-based systems like DeepHeart to improve human health: confounding factors, deployment in a medical setting, interpretability and the time-scale and large volume of data.
“While these challenges may seem daunting, the constraints of medicine — scarce labeled data, confounding factors, difficulty of deployment and the necessity of interpretability — also represent opportunities to develop new techniques,” the researchers wrote. “This work is a first step in showing how health conditions can be detected using techniques first developed in natural language processing and computer vision.”
The researchers noted the algorithm is currently in use in several ambulatory, mobile trials in partnership with major medical centers to show real-world efficacy and help build a clinical evidence base. – by Regina Schaffer
Reference:
Ballinger B, et al. DeepHeart: Semi-supervised sequence learning for cardiovascular risk prediction. Presented at: AAAI Conference on Artificial Intelligence; Feb. 2-7, 2018; New Orleans.
Disclosure: Endocrine Today could not confirm relevant financial disclosures at the time of publication.