Computer algorithm tracks alopecia ‘in standardized way’ using cell phone camera images
A novel computer-based algorithm can track the progress of hair loss in patients with various types of alopecia, according to a study.
“Hair loss impacts so many people,” Elena Bernardis, PhD, of the department of dermatology at the Perelman School of Medicine at the University of Pennsylvania, told Healio. “If you have had alopecia or know anyone who is affected by it, you know how profound that impact is. Despite the staggering numbers, technology to help quantify, diagnose and treat alopecia patients is still very limited and there are still many unknowns leading to many active areas of research.”
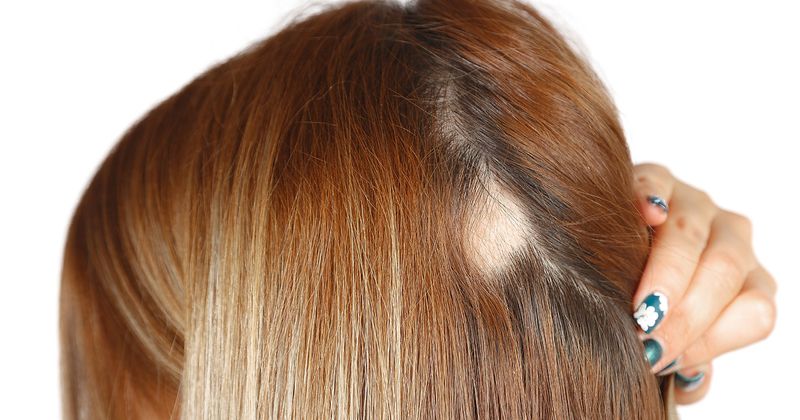
For each of these clinical scenarios, the first step is to quantify the hair loss, according to Bernardis.
“That is where computer vision comes in,” she said.
In the study, Bernardis and colleagues aimed to develop and validate a new model, HairComb, to automatically compute the percentage hair loss from images regardless of the alopecia subtype of the patient.
“It is very interesting to design an algorithm that needs to resolve discrepancies between clinical interpretation vs. pixel information,” Bernardis said. “For example, some of the discrepancies include how to deal with loose hair strands over scalp areas; same hair densities having different hair loss meanings; or the fact that the concept of density is over an area and not a single pixel.”
The unique design of both the algorithm and dataset created by the group combine overall clinical alopecia and pixel-level hair information, according to Bernardis.
Images were gathered at three centers in Philadelphia between 2015 and 2021 and analyzed between 2019 and 2021. Correlation between scoring systems served as the primary endpoint. The researchers used image segmentation accuracy, density probability regression error and average percentage hair loss error for labeled images to assess these endpoints.
The analysis included 404 participants aged 2 years or older. Ultimately, data for 250 patients (70.4% female; mean age, 35.3 years) were included, with 70 patients with androgenetic alopecia; 66 with alopecia areata; 50 with central centrifugal cicatricial alopecia; and 27 with frontal fibrosing alopecia, lichen planopilaris, telogen effluvium or other alopecia diagnoses. There were also 32 scalps with no alopecia included.
Results indicated that the scoring systems strongly correlated with underlying percentage of hair loss, including coefficient of determination R2 values of 0.793 and 0.804 for log of percentage hair loss.
The HairComb system had 92% accuracy, with a 5% regression error and a 7% difference in hair loss. Predicted scores were comparable to annotators, according to the findings.
“The algorithm presented, HairComb, was trained over a wide range of alopecia images taken in a clinical setting,” Bernardis said. “Yet, it is surprising to see how well it also handles images collected from the public.”
Bernardis noted that despite the widespread taking and sending of photos using text and social media, many images sent for clinical evaluation have variability in quality.
“They have poor framing and blurriness,” she said. “Having an algorithm that is able to handle subpar image quality is very important.”
This system could have significant clinical implications, according to Bernardis.
“To our knowledge, this is the first automated system that allows anyone with a camera to take a photo of the scalp and return in real-time the hair density at every point, in a standardized and automated way, and for all alopecia subtypes,” she said.
Because it only requires a cell phone camera, there is no need for specialized or expensive equipment, Bernardis added.
“Anyone can use it,” she said. “So, not only did we create a clinical tool that can be used for research or to assess patients during a clinical visit, but one that can be used for teledermatology and for patients at home. For example, it can be used to take images in-between visits to help follow patient progression.”